Developing and optimizing machine learning algorithms for predicting in-hospital patient charges for Congestive Heart Failure Exacerbations, Chronic Obstructive Pulmonary Disease Exacerbations and Diabetic Ketoacidosis
medrxiv(2023)
摘要
Background Hospitalizations for exacerbations of congestive heart failure (CHF), chronic obstructive pulmonary disease (COPD) and diabetic ketoacidosis (DKA) are costly in the United States.
Objective The purpose of this study is to predict in-hospital charges for each condition using Machine Learning (ML) models.
Methods We conducted a retrospective cohort study on national discharge records of hospitalized adult patients from January 1st, 2016, to December 31st, 2019. We used numerous ML techniques to predict in-hospital total cost.
Results We found that linear regression (LM), gradient boosting (GBM) and extreme gradient boosting (XGB) models had good predictive performance and were statistically equivalent, with training R-Squared values ranging from 0.49–0.95 for CHF; 0.56–0.95 for COPD; and 0.32–0.99 for DKA. We identified important key features driving costs, including patient age, length-of-stay, number of procedures. and elective/non-elective admission.
Conclusions ML methods may be used to accurately predict costs and identify drivers of high cost for COPD exacerbations, CHF exacerbations and DKA. Overall, our findings may inform future studies that seek to decrease the underlying high patient costs for these conditions.
### Competing Interest Statement
The authors have declared no competing interest.
### Funding Statement
Funding: The research reported in this manuscript is supported, in part, by the Institutional Clinical and Translational Science Award (CTSA) with Dr. Boland as a co-Investigator (UL1-TR-001878) with Dr. Garret Fitzgerald as PI. Generous funding also provided by the University of Pennsylvania.
### Author Declarations
I confirm all relevant ethical guidelines have been followed, and any necessary IRB and/or ethics committee approvals have been obtained.
Yes
I confirm that all necessary patient/participant consent has been obtained and the appropriate institutional forms have been archived, and that any patient/participant/sample identifiers included were not known to anyone (e.g., hospital staff, patients or participants themselves) outside the research group so cannot be used to identify individuals.
Yes
I understand that all clinical trials and any other prospective interventional studies must be registered with an ICMJE-approved registry, such as ClinicalTrials.gov. I confirm that any such study reported in the manuscript has been registered and the trial registration ID is provided (note: if posting a prospective study registered retrospectively, please provide a statement in the trial ID field explaining why the study was not registered in advance).
Yes
I have followed all appropriate research reporting guidelines, such as any relevant EQUATOR Network research reporting checklist(s) and other pertinent material, if applicable.
Yes
All data produced in the present study are available upon reasonable request to the authors.
更多查看译文
AI 理解论文
溯源树
样例
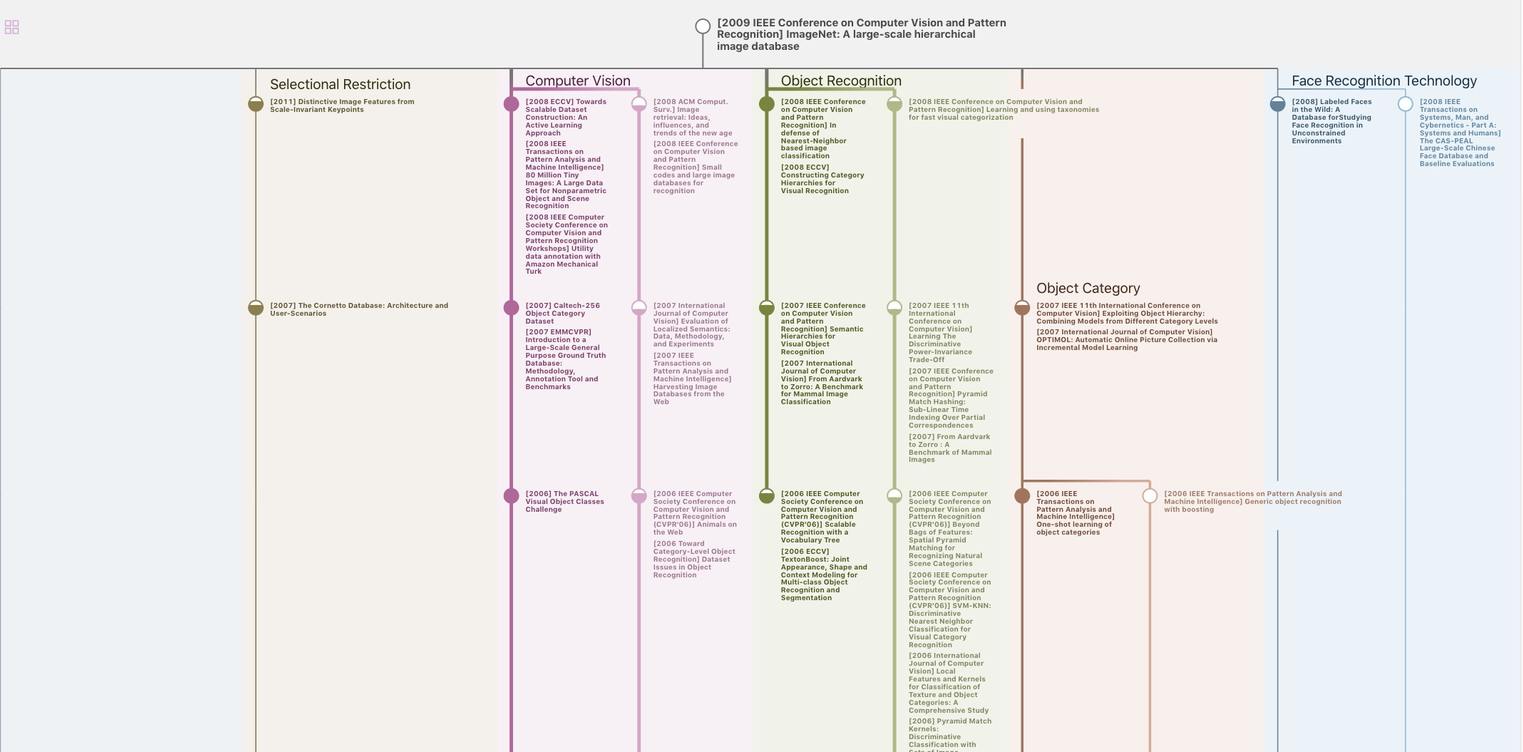
生成溯源树,研究论文发展脉络
Chat Paper
正在生成论文摘要