Multi-label Feature selection with adaptive graph learning and label information enhancement
KNOWLEDGE-BASED SYSTEMS(2024)
摘要
The high dimensionality and complexity of multi -label data make obtaining accurate label sets in practical applications difficult. Noisy data in the labels will affect the model's classification performance. Existing methods only reconstruct logical labels into semantic labels to mitigate the effect of inherent noise and do not specifically address the noise. In order to solve this problem and eliminate the effect of noise, this paper proposes a multi -label feature selection method with adaptive graph learning and label information enhancement (AGLE). First, each label is represented as a combination of other labels using global label correlation, which can reconstruct the original label matrix to obtain the self-expression label matrix. Based on this, similar labels in two matrices can be considered reliable labels, and not similar may be noise. Therefore, constructing a predictive label matrix to weigh the learning of original and self-expression labels can enhance reliable information. Second, an adaptive graph is utilized to learn global label correlation to ensure that the self-expression label matrix can be continuously optimized. Then, the local label manifold structure is utilized to constrain the adaptive graph for more stable performance. Finally, the adaptive graph and t2,1 -norm are combined to guide learning the feature weight matrix. For the proposed method, an iterative algorithm based on alternating optimization is used to compute the weight matrix. Comparing experimental results with several state-of-the-art algorithms demonstrates the effectiveness of the AGLE method for the noise problem.
更多查看译文
关键词
Adaptive graph,Label information enhancement,Sparse learning,Label correlation
AI 理解论文
溯源树
样例
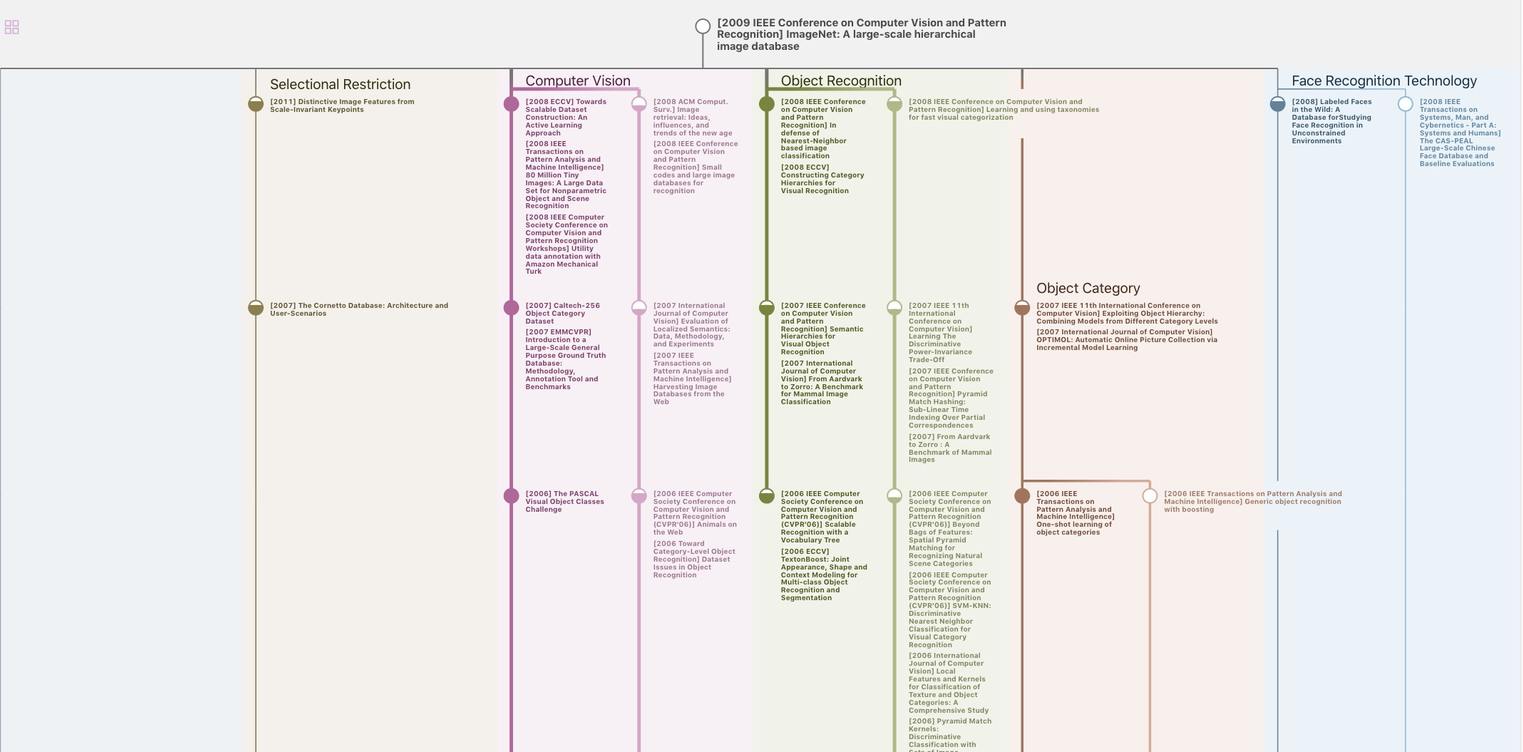
生成溯源树,研究论文发展脉络
Chat Paper
正在生成论文摘要