An adaptive evolutionary multi-objective clustering based on the data properties of the base partitions
EXPERT SYSTEMS WITH APPLICATIONS(2024)
摘要
Evolutionary multi -objective clustering (EMOC) is a modern clustering technique in which the general concepts of evolutionary multi -objective optimization are applied to the clustering problem. The design and definition of clustering are difficult problems, and the choice of the objective functions and parameter setting of the algorithms are still challenges. In this paper, we propose the adaptive evolutionary multi -objective clustering approach based on data properties (AEMOC). AEMOC considers a new metric to evaluate the base partitions (candidate solutions used in the initial population) generated by minimum spanning tree clustering. This metric is applied to: (1) determine properties of the data, such as separation and overlap; and (2) define an offline selection of objective functions and parameter settings of the multi -objective algorithm. The information regarding the data properties allows AEMOC to avoid unnecessary data processing in datasets in which the initialization provides optimal solutions and optimization is not required. AEMOC presented promising results considering a diverse set of artificial and real -life datasets, based on two aspects: (1) it succeeded in the determination of the data properties of the base partitions and verifying the potential clustering quality, presenting a correlation of 0.8 with a reference metric, and (2) it provided better clustering results than reference EMOC approaches, achieving a general gain in the clustering performance of 3% in real -life datasets and 7% in artificial datasets.
更多查看译文
关键词
Clustering criteria,Multi-objective clustering,Evolutionary multi-objective optimization,Clustering analysis
AI 理解论文
溯源树
样例
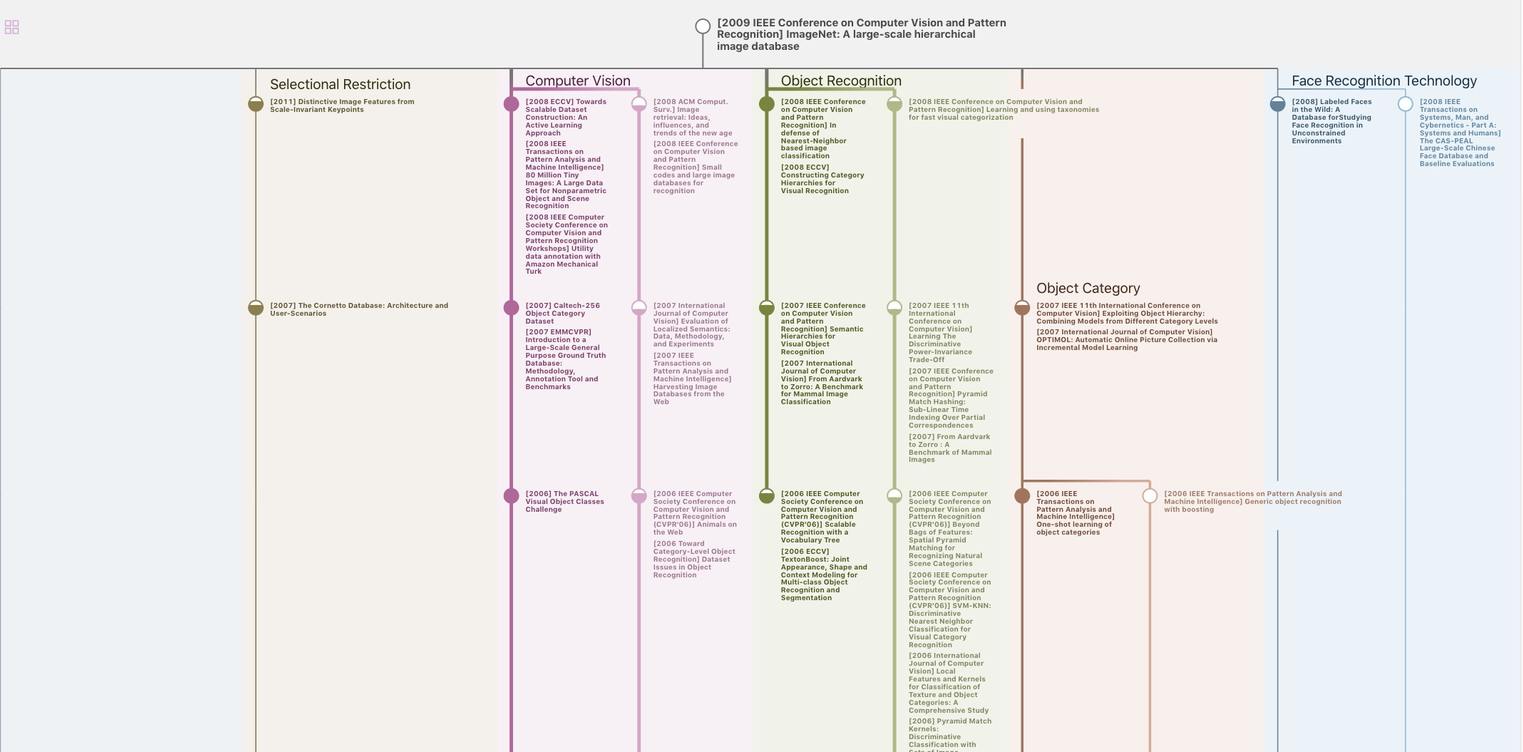
生成溯源树,研究论文发展脉络
Chat Paper
正在生成论文摘要