Alleviating repetitive tokens in non-autoregressive machine translation with unlikelihood training
Soft Computing(2024)
摘要
In recent years, significant progress has been made in the field of non-autoregressive machine translations. However, the accuracy of non-autoregressive models still lags behind their autoregressive counterparts. This discrepancy can be attributed to the abundance of repetitive tokens in the target sequences generated by non-autoregressive models. In this study, we delve into this phenomenon and propose a novel approach to train a non-autoregressive model using unlikelihood loss. We evaluate our method on three widely used benchmark tasks. The experimental results demonstrating that our proposed approach significantly reduces the number of repetitive tokens while improving the overall performance of non-autoregressive machine translations. Compared to the baseline model ”Mask-Predict”, the average number of repetitions on IWSLT 14 DE → EN valid set is reduced from 0.48 to 0.17, resulting in a remarkable 62
更多查看译文
关键词
Machine translation,Non-autoregressive,Repetitive tokens,Unlikelihood training
AI 理解论文
溯源树
样例
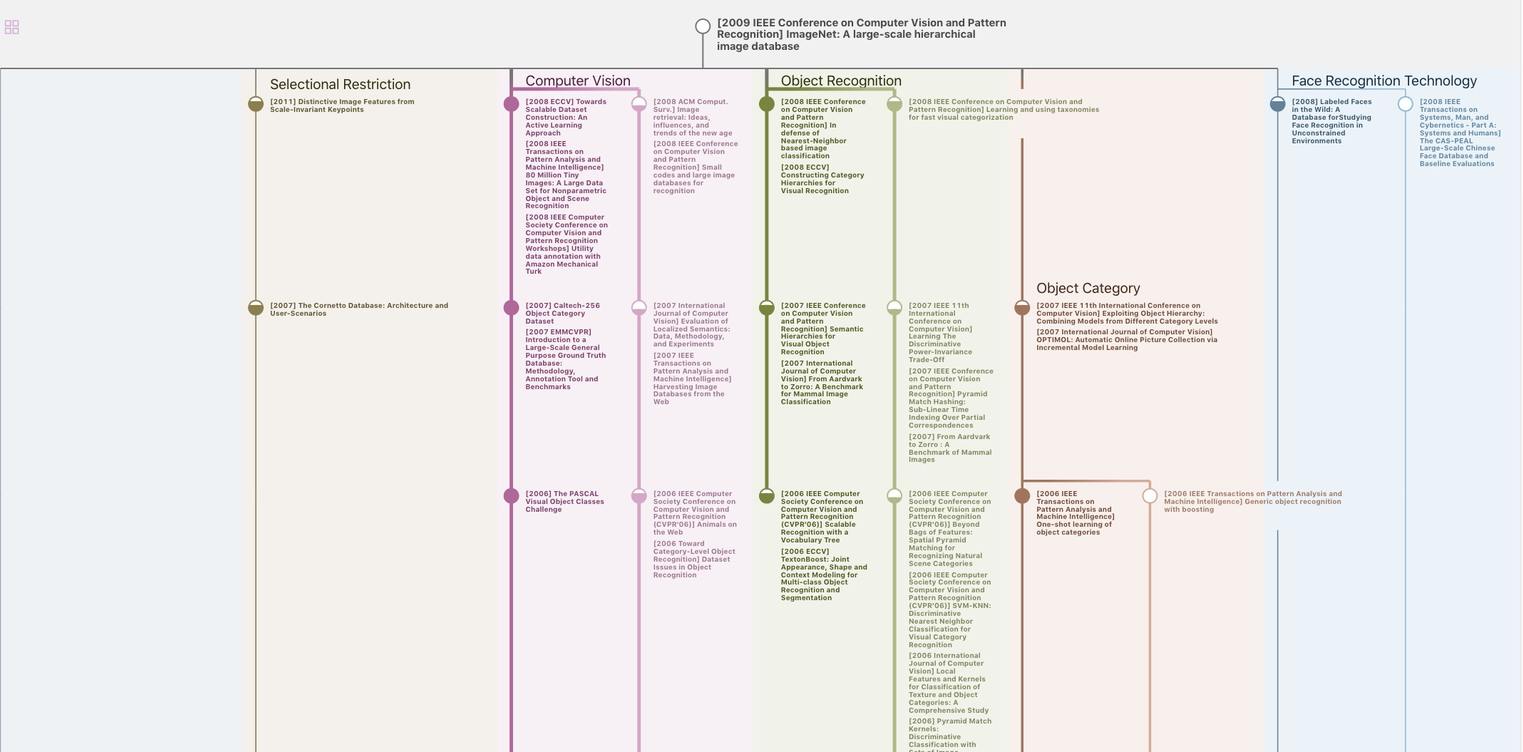
生成溯源树,研究论文发展脉络
Chat Paper
正在生成论文摘要