Local and soft feature selection for value function approximation in batch reinforcement learning for robot navigation
The Journal of Supercomputing(2024)
摘要
This paper proposes a novel method for robot navigation in high-dimensional environments that reduce the dimension of the state space using local and soft feature selection. The algorithm selects relevant features based on local correlations between states, avoiding duplicate inappropriate information and adjusting sensor values accordingly. By optimizing the value function approximation based on the local weighted features of states in the reinforcement learning process, the method shows improvements in the robot’s motion flexibility, learning time, the distance traveled to reach its goal, and the minimization of collisions with obstacles. This approach was tested on an E-puck robot using the Webots robot simulator in different test environments.
更多查看译文
关键词
Reinforcement learning,Value function approximation,Local relevance feature selection,Robot navigation
AI 理解论文
溯源树
样例
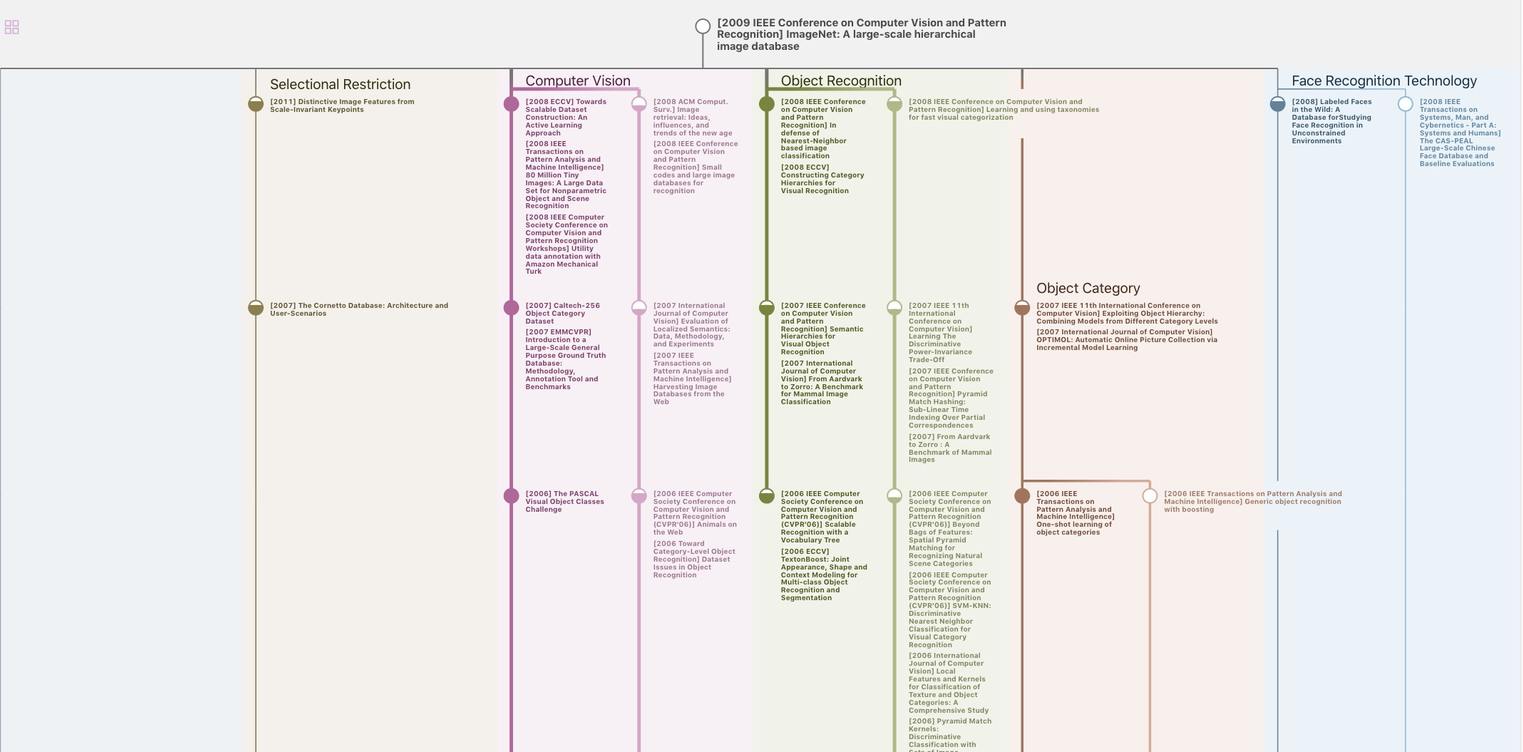
生成溯源树,研究论文发展脉络
Chat Paper
正在生成论文摘要