Bayesian Inversion of Frequency-Domain Airborne EM Data With Spatial Correlation Prior Information
IEEE TRANSACTIONS ON GEOSCIENCE AND REMOTE SENSING(2024)
摘要
The Bayesian inversion of electromagnetic data can obtain key information on the uncertainty of subsurface resistivity. However, due to its high computational cost, Bayesian inversion is largely limited to 1-D resistivity models. In this study, a fast Bayesian inversion method is implemented by introducing the spatial correlation as prior information. The contributions of this article mainly include: 1) explicitly introduce the expression of spatial correlation prior information and provide a method to determine the parameters in the expression through the variogram theory. The influence of parameters in the spatial correlation prior information on the inversion results is systematically analyzed with the 1-D synthetic model. 2) The information entropy theory of continuous functions is introduced to quantify the degrees of freedom (DOF) of the parameters of the spatial correlation prior model. The analysis shows that the DOF of model parameters are significantly smaller than the number of model parameters when spatial correlation prior information is introduced, which is the main reason for the rapid Bayesian inversion. 3) Introducing the Sengpiel fast imaging algorithm, combined with the variogram theory, realized the direct acquisition of spatial correlation prior information from the observation data, minimizing the dependence on other information. The inversion results of 1-D and 2-D synthetic models and field datasets show that considering the spatial correlation prior information, hundreds of thousands of Markov chain Monte Carlo sampling steps are needed to enable the inversion of up to thousands of model parameters. This result provides a possible idea for future Bayesian inversion of complex 3-D models.
更多查看译文
关键词
Correlation,Bayes methods,Conductivity,Data models,Solid modeling,Computational modeling,Geology,Airborne electromagnetic (AEM),Bayesian inversion,Markov chain Monte Carlo (MCMC),statistical methods
AI 理解论文
溯源树
样例
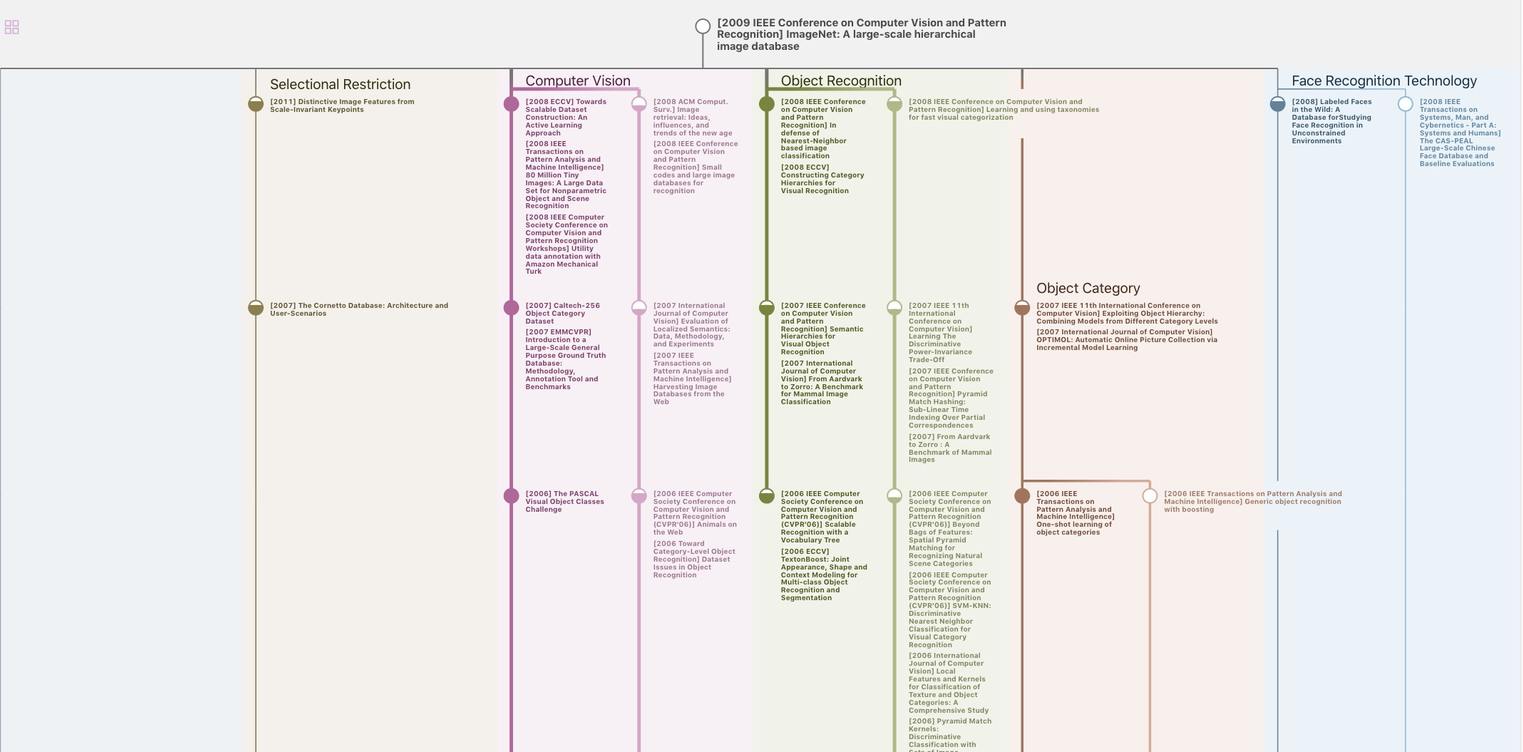
生成溯源树,研究论文发展脉络
Chat Paper
正在生成论文摘要