Large-Scale Traffic Signal Control Based on Multi-Agent Q-Learning and Pressure
IEEE ACCESS(2024)
摘要
Traffic congestion has become an increasingly severe problem and needs to be solved urgently. Reinforcement learning (RL) is an advanced data-driven approach for large-scale adaptive traffic signal control (ATSC) in the complex urban traffic network. The decentralized multi-agent RL (MARL) framework is feasible for multi-intersection scenarios. However, the environment becomes partially observable and nonstationary without effective communication among agents from the perspective of each local agent. Previous studies solve this problem by improving the observation of each local agent and using a spatial discount factor. In this paper, we are motivated by achieving coordination among intersections in the RL settings and better performance in ATSC and solve the problem of traffic signal control at multiple intersections in large-scale networks based on RL techniques. Pressure is used as an indicator to represent the traffic conditions of an intersection considering its neighbor intersections. A new reward function with pressure and vehicle waiting time is designed to achieve coordination among intersections. The above three control policies are innovatively applied to a multi-agent Q-learning method for ATSC at multiple intersections. Experiments are carried out on a large synthetic traffic grid under simulated peak-hour traffic dynamics. Results demonstrate that our approach outperforms the state-of-the-art decentralized MARL algorithms in average queue length and intersection delay.
更多查看译文
关键词
Q-learning,Training,Vehicle dynamics,Traffic congestion,Heuristic algorithms,Delays,Behavioral sciences,Adaptive traffic signal control,multi-agent reinforcement learning,max pressure control
AI 理解论文
溯源树
样例
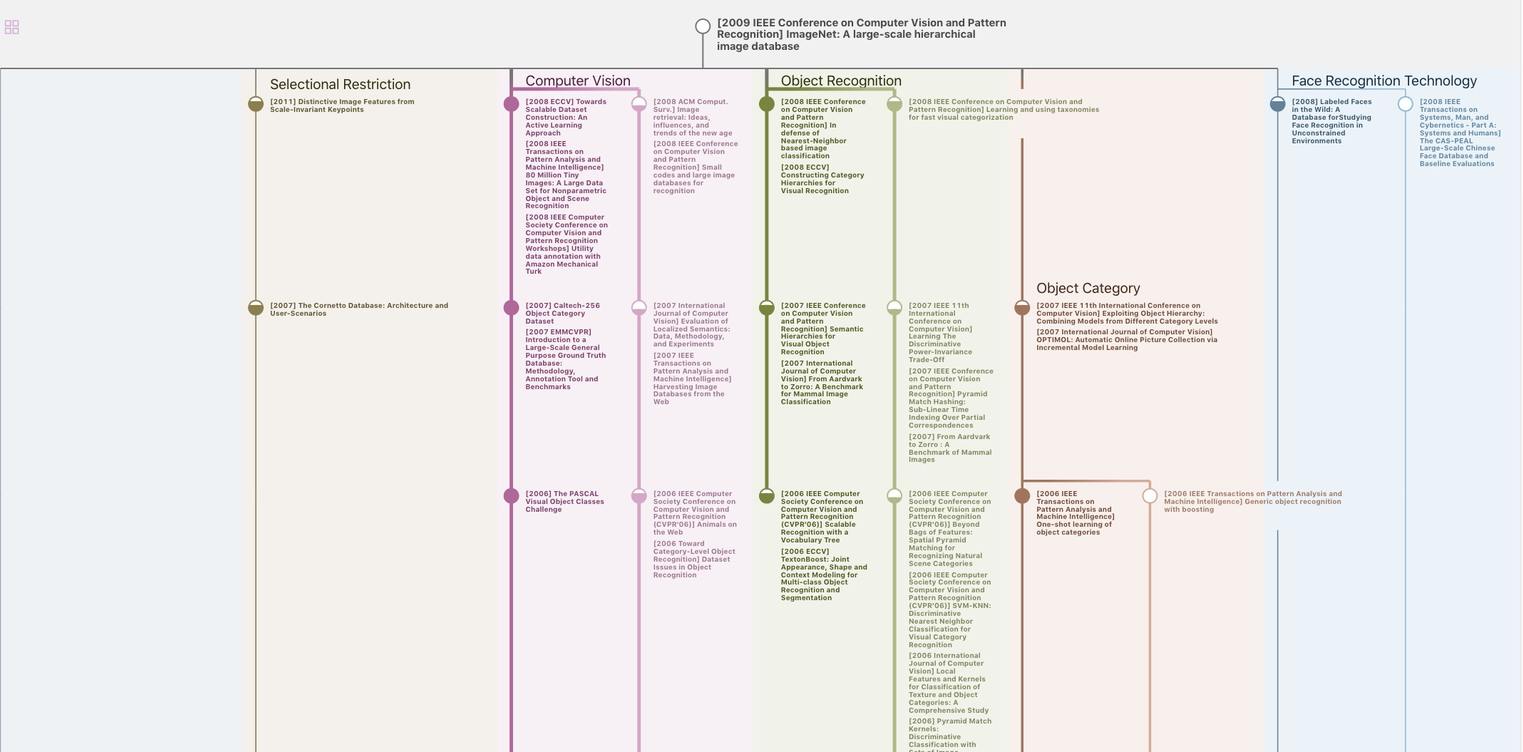
生成溯源树,研究论文发展脉络
Chat Paper
正在生成论文摘要