A Novel Feature Extraction Approach for the Clustering and Classification of Genome Sequences.
2023 IEEE Symposium Series on Computational Intelligence (SSCI)(2023)
摘要
Feature extraction is essential in bioinformatics because it transforms genome sequences into the feature vectors required for data mining activities such as classification and clustering. The data mining activities enable us to classify or cluster the newly sequenced genome to the known families. Nowadays, a variety of feature extraction strategies are available for genome data. Nevertheless, several existing algorithms do not extract context-sensitive key properties, also some approaches extract features, which are unable to distinguish between two non-similar sequences. In addition, the efficacy of existing feature extraction techniques is evaluated on either supervised or unsupervised learning models, but not on both. Thus, an efficient feature extraction technique that extracts significantly relevant features from genome sequences is required. In this paper, a novel feature extraction method is proposed that extracts features based on the length of the sequence, the frequency of nucleotide bases, the modified positional sum of nucleotide bases, the distribution of nucleotide bases, and the entropy of the sequence to generate a 14-dimensional fixed-length numeric vector to describe each genome sequence uniquely. By applying extracted features to both supervised and unsupervised machine learning approaches, the performance of the proposed feature extraction method is assessed. The experimental results show that the proposed strategy for clustering and classifying novel genome sequences into recognized genome classes is highly effective and efficient. The same is proven by comparing the proposed method to the standard state-of-the-art method.
更多查看译文
关键词
Feature extraction,Genome sequences,Clustering,Classification,Single nucleotide polymorphism
AI 理解论文
溯源树
样例
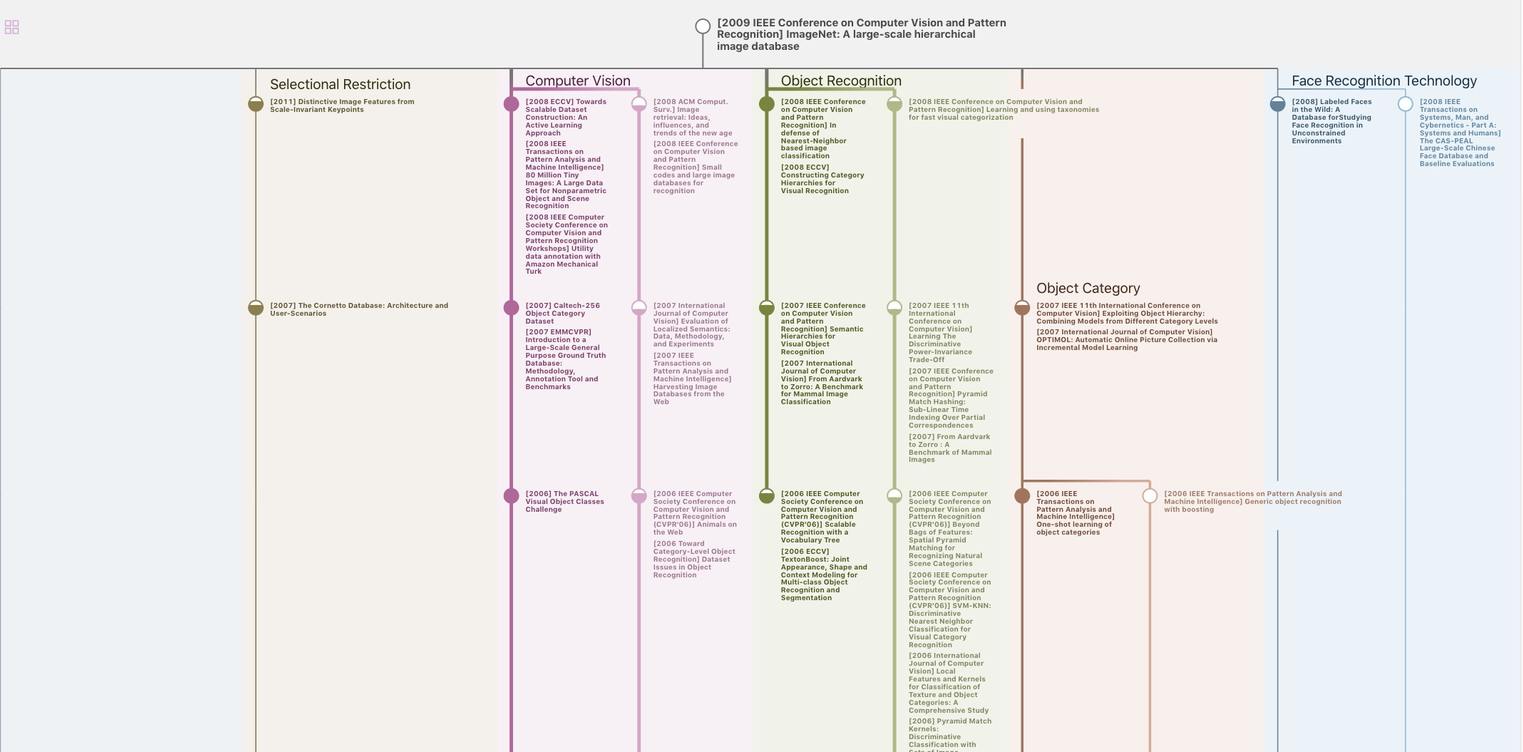
生成溯源树,研究论文发展脉络
Chat Paper
正在生成论文摘要