Joint Multi-Facts Reasoning Network For Complex Temporal Question Answering Over Knowledge Graph
CoRR(2024)
摘要
Temporal Knowledge Graph (TKG) is an extension of regular knowledge graph by
attaching the time scope. Existing temporal knowledge graph question answering
(TKGQA) models solely approach simple questions, owing to the prior assumption
that each question only contains a single temporal fact with explicit/implicit
temporal constraints. Hence, they perform poorly on questions which own
multiple temporal facts. In this paper, we propose Joint
Multi Facts
Reasoning Network (JMFRN), to jointly
reasoning multiple temporal facts for accurately answering complex
temporal questions. Specifically, JMFRN first retrieves question-related
temporal facts from TKG for each entity of the given complex question. For
joint reasoning, we design two different attention (entity-aware and
time-aware) modules, which are suitable for universal settings, to aggregate
entities and timestamps information of retrieved facts. Moreover, to filter
incorrect type answers, we introduce an additional answer type discrimination
task. Extensive experiments demonstrate our proposed method significantly
outperforms the state-of-art on the well-known complex temporal question
benchmark TimeQuestions.
更多查看译文
关键词
Temporal knowledge graph question answering,knowledge graph,neural language processing
AI 理解论文
溯源树
样例
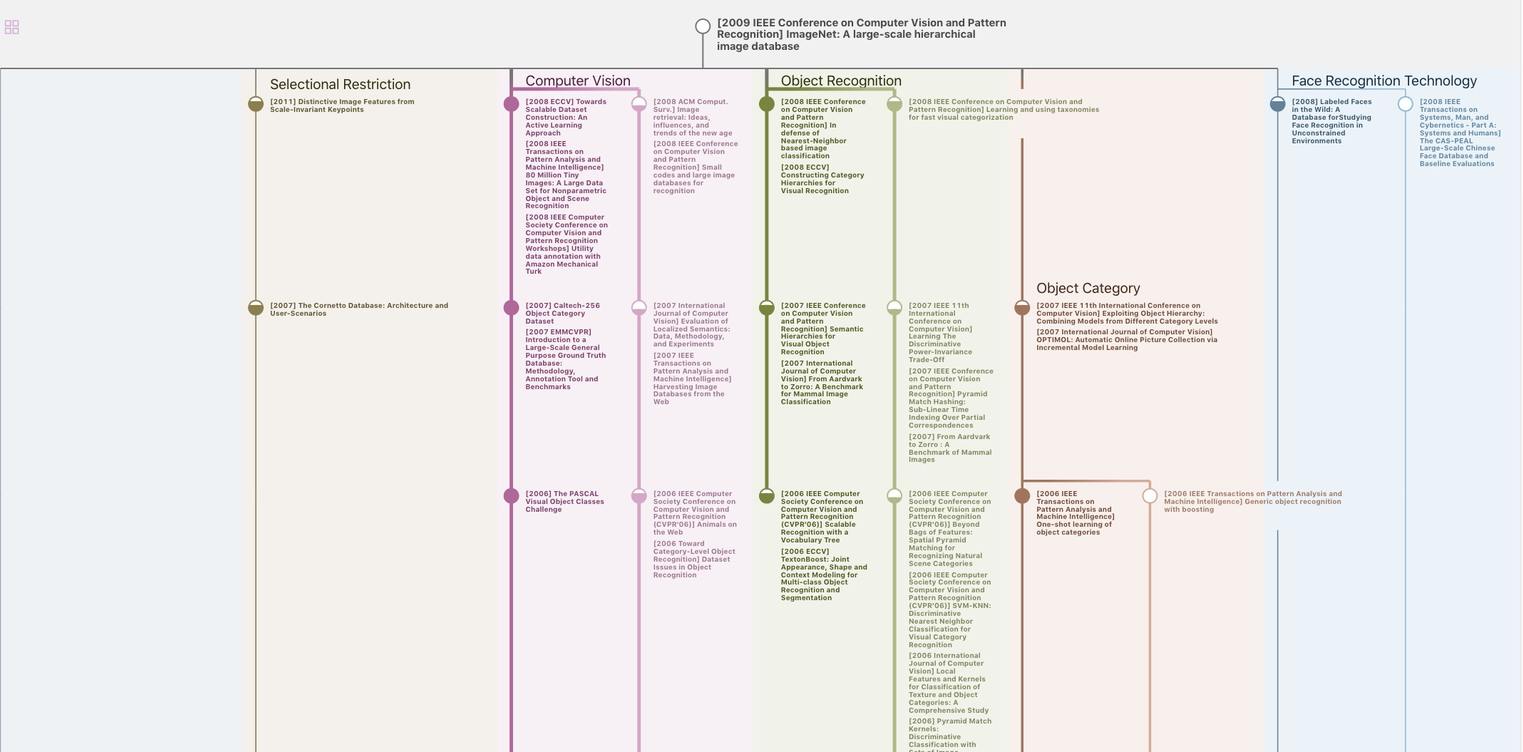
生成溯源树,研究论文发展脉络
Chat Paper
正在生成论文摘要