Enhanced autoencoder-based LiDAR localization in self-driving vehicles
APPLIED SOFT COMPUTING(2024)
摘要
The ability of self -driving vehicles to carry out navigation tasks successfully relies heavily on the implementation of a strong localization system. Global navigation satellite systems have been used to provide such information to the vehicle. However, this faces challenges related to the position computation in certain environments, such as in tunnels, under bridges, or in urban canyons. This paper proposes an autoencoder based method to predict a vehicle's position based on a LiDAR point cloud (converted into a reference system) without using additional sensors. The proposed model modifies the objective function of the autoencoder to include an additional objective responsible for adapting the output of the encoder layer (the latent space) to predict the position. Moreover, a penalty parameter was used in the objective function to emphasize the learning in the latent space. The convergence of the proposed method was proven by showing that it is a stochastic -local -quasi -convex function, which ensures the effectiveness of the Stochastic Normalized Gradient Descent algorithm in achieving convergence. The NCLT dataset was used to train our model in PyTorch. In comparison to various advanced registration techniques, our method achieves excellent results for the majority of the Kitti dataset sequences as well. The method achieved a mean absolute error of about 0.5, which is desirable for autonomous -driving vehicles.
更多查看译文
关键词
Self-driving vehicles,Localization,Mapping,Optimization,Autoencoder,Latent space
AI 理解论文
溯源树
样例
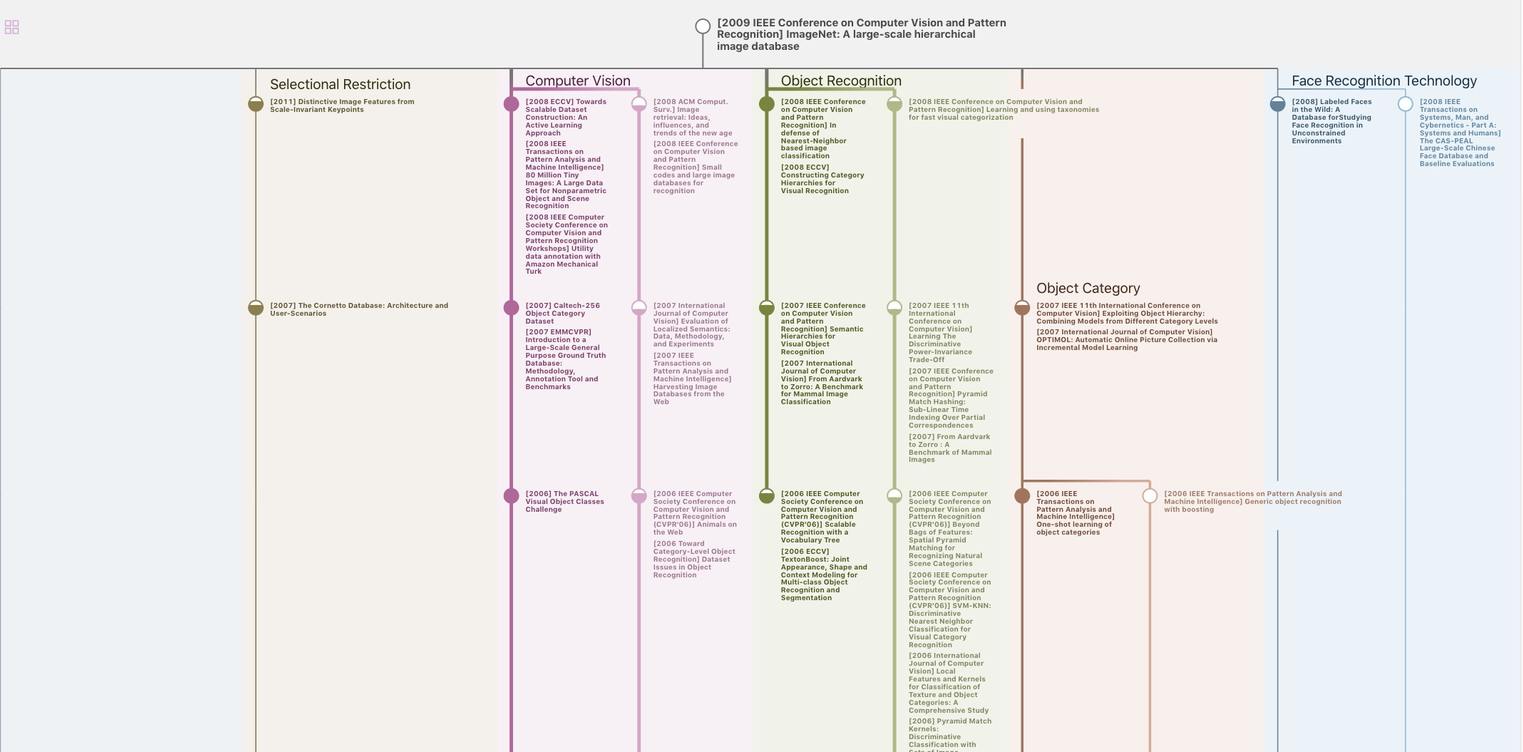
生成溯源树,研究论文发展脉络
Chat Paper
正在生成论文摘要