Thermo-electro-rheological properties of graphene oxide and MXene hybrid nanofluid for vanadium redox flow battery: Application of explainable ensemble machine learning with hyperparameter optimization
FLATCHEM(2024)
摘要
Recent research has extensively focused on 2D materials such as graphene oxide (GO) and MXene due to their intriguing properties, significantly advancing nanotechnology and materials research. This experimental study explores the use of a vanadium electrolyte-based hybrid nanofluid (HNF) composed of GO and MXene (90:10) to enhance vanadium redox flow batteries (VRFBs). The synthesis and characterization of GO and Mxene nanoparticles (NPs) were conducted using various techniques. The HNF, produced at different weight concentrations, underwent analysis for stability, rheology, thermal conductivity (TC), and electrical conductivity (EC) within a temperature range of 10-45 degrees C. The results indicate that the HNF exhibits favorable stability and Newtonian behavior in the specified temperature range. At 45 degrees C, the HNF achieves a maximum enhancement of 20.5 % in EC and 6.81 % in TC for 0.1 wt% compared to the vanadium electrolyte. Subsequently, a prognostic model was developed using an explainable ensemble LSBoost-based machine learning approach, employing a test dataset and applying 5-fold cross-validation to prevent overfitting. Hyperparameter optimization was achieved using the Bayesian technique. The LSBoost-based prognostic models created for TC, EC, and viscosity (VST) demonstrated high effectiveness, with R(2 )values of 0.9981, 0.99, and 0.9954, respectively. The prediction errors were minimal, with RMSE values of 0.00089255, 5.553, and 0.09391 for the TC, EC, and VST models, respectively. Similarly, the MAE values were low, at 0.00068948, 4.0919, and 0.06129.
更多查看译文
关键词
Electrical conductivity,Graphene oxide,Explainable Machine learning,Mxene,Redox flow battery,Vanadium,Renewable energy
AI 理解论文
溯源树
样例
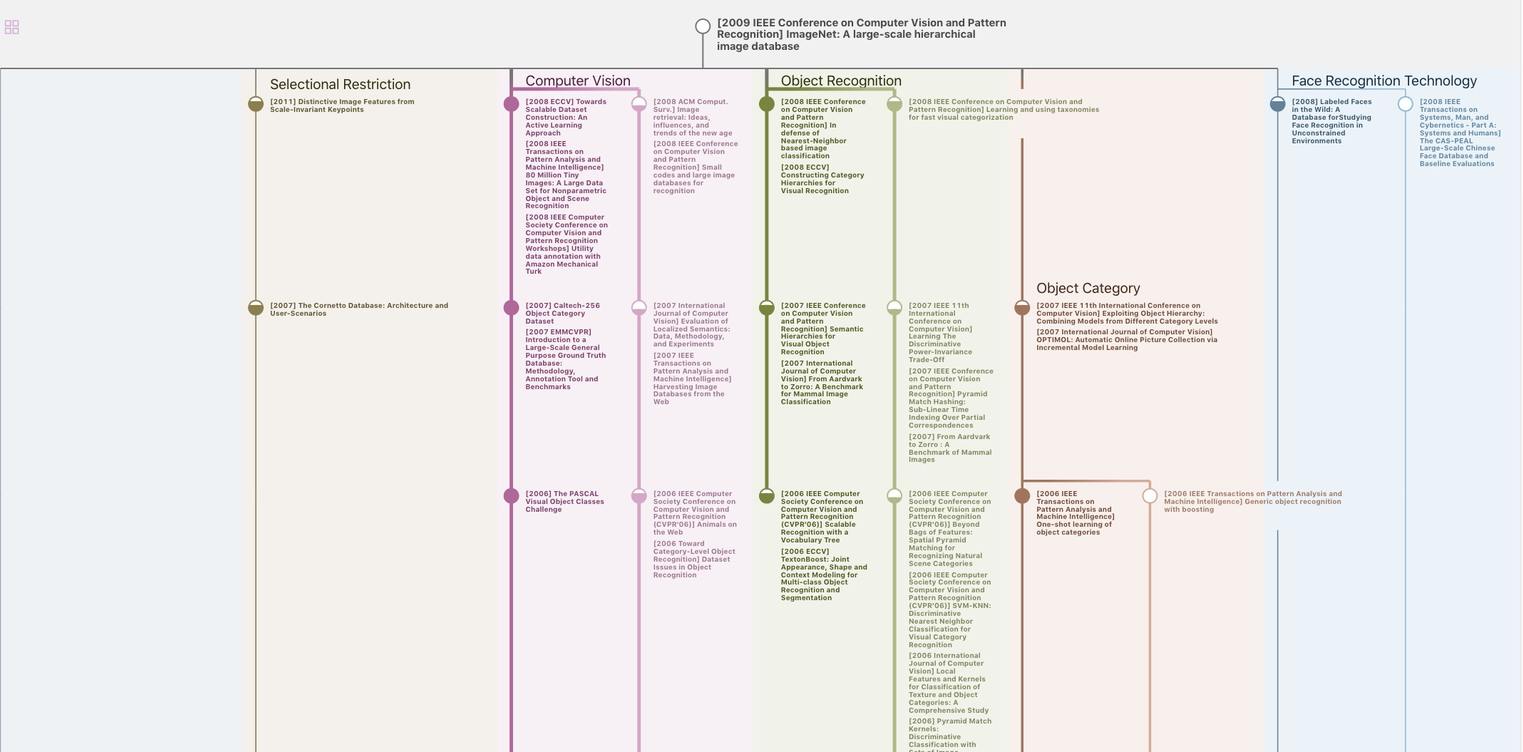
生成溯源树,研究论文发展脉络
Chat Paper
正在生成论文摘要