A Joint Saliency Temporal–Spatial–Spectral Information Network for Hyperspectral Image Change Detection
IEEE Transactions on Geoscience and Remote Sensing(2023)CCF BSCI 2区
Wuhan Univ Technol | Xidian Univ | Fuzhou Univ
Abstract
Hyperspectral image change detection (HSI-CD) is a fundamental task in the field of remote sensing (RS) observation, which utilizes the rich spectral and spatial information in bitemporal HSIs to detect subtle changes on the Earth's surface. However, modern deep learning (DL)-based HSI-CD methods mostly rely on patch-based methods, which leads to spectral band redundancy and spatial information noise in limited receiving domains, thus ignoring the extraction and utilization of saliency information and limiting the improvement of CD performance. To address these issues, this article proposes a joint saliency temporal-spatial-spectral information network (STSS-Net) for HSI-CD. The principal contributions of this article can be summarized: 1) we have designed a spatial saliency information extraction (SSIE) module for denoising based on distance from center pixels and spectral similarity of the substance, which increases the attention to spatial differences between similar spectral substances and different spectral substances; 2) we have designed a compact high-level spectral information tokenizer (CHLSIT) for spectral saliency information, where the high-level conceptual information of changes in spectral interest can be represented by nonlinear combinations of spectral bands, and redundancy can be removed by extracting high-level spectral conceptual features; and 3) utilizing the advantages of CNN and transformer architectures to combine temporal-spatial-spectral information. The experimental results on three real HSI-CD datasets show that STSS-Net can improve the accuracy of CD and has a certain improvement in the detection of edge information and complex information.
MoreTranslated text
Key words
Attention,change detection,convolutional neural networks (CNNs),hyperspectral image (HSI),saliency information,transformer
求助PDF
上传PDF
View via Publisher
AI Read Science
AI Summary
AI Summary is the key point extracted automatically understanding the full text of the paper, including the background, methods, results, conclusions, icons and other key content, so that you can get the outline of the paper at a glance.
Example
Background
Key content
Introduction
Methods
Results
Related work
Fund
Key content
- Pretraining has recently greatly promoted the development of natural language processing (NLP)
- We show that M6 outperforms the baselines in multimodal downstream tasks, and the large M6 with 10 parameters can reach a better performance
- We propose a method called M6 that is able to process information of multiple modalities and perform both single-modal and cross-modal understanding and generation
- The model is scaled to large model with 10 billion parameters with sophisticated deployment, and the 10 -parameter M6-large is the largest pretrained model in Chinese
- Experimental results show that our proposed M6 outperforms the baseline in a number of downstream tasks concerning both single modality and multiple modalities We will continue the pretraining of extremely large models by increasing data to explore the limit of its performance
Upload PDF to Generate Summary
Must-Reading Tree
Example
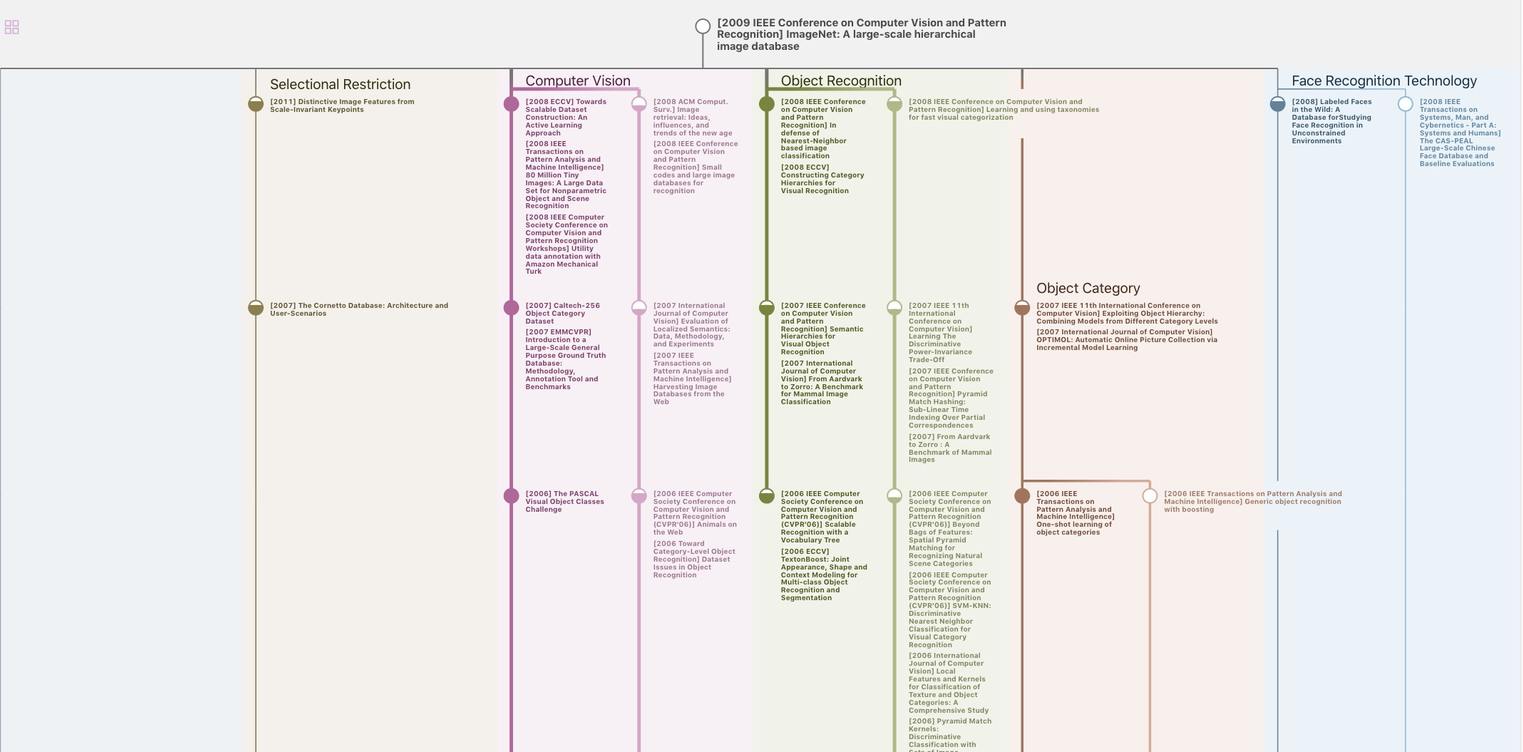
Generate MRT to find the research sequence of this paper
Related Papers
arXiv (Cornell University) 2024
被引用0
Data Disclaimer
The page data are from open Internet sources, cooperative publishers and automatic analysis results through AI technology. We do not make any commitments and guarantees for the validity, accuracy, correctness, reliability, completeness and timeliness of the page data. If you have any questions, please contact us by email: report@aminer.cn
Chat Paper
GPU is busy, summary generation fails
Rerequest