An efficient urban flood mapping framework towards disaster response driven by weakly supervised semantic segmentation with decoupled training samples
ISPRS JOURNAL OF PHOTOGRAMMETRY AND REMOTE SENSING(2024)
摘要
Despite the proven effectiveness of data-driven deep learning techniques in urban flood mapping, the availability of annotation data remains a critical factor impeding their timeliness in real applications. Recent progress in weakly supervised semantic segmentation (WSSS) presents promising solutions for addressing this limitation. To accomplish prompt and accurate flood mapping in complex urban areas from high-resolution remote sensing images to support disaster management and response, this study makes contributions in three key aspects: weak training data generation, the improvement of WSSS algorithm, and the construction of benchmark datasets. Firstly, we present a novel yet efficient weak training data generation strategy by decoupling the acquisition of positive and negative samples. This strategy enables the rapid generation of block-level weak annotations assisted by pre-flood river data or the segment anything model (SAM) for zero-shot segmentation, thereby alleviating the burden of weak data labeling. Secondly, to enhance the flood detection results in complex urban landscapes based on low-cost weak labels, we design an end-to-end WSSS framework incorporating tree filteringbased structure constraints and a perturbed dual-branch cross self-distillation mechanism. Lastly, to evaluate the performance of the proposed approach, we constructed two large aerial imagery datasets, namely Calgary-Flood and Huston-Flood. These datasets encompass diverse urban land covers and include challenging scenarios with extensive shadows, providing a robust benchmark for evaluating our method against various urban environments. Experimental results demonstrate that our weak data annotation strategy substantially enhances efficiency. Additionally, the proposed WSSS framework exhibits superior performance in comparison to existing state-of-the-art methods, particularly in terms of edge delineation and algorithmic stability. The advancements in weak data annotation strategy, end-to-end model architecture, and benchmark dataset development in this study collectively exploit the potential of the weakly supervised paradigm for rapid flood mapping in urgent situations. The datasets and code will be publicly available at (https://github.com/YJ-He/Flood_Mapp ing_WSSS).
更多查看译文
关键词
Rapid flood mapping,Weakly supervised semantic segmentation,Sparse label,Tree filtering,Self-distillation,Segment anything model
AI 理解论文
溯源树
样例
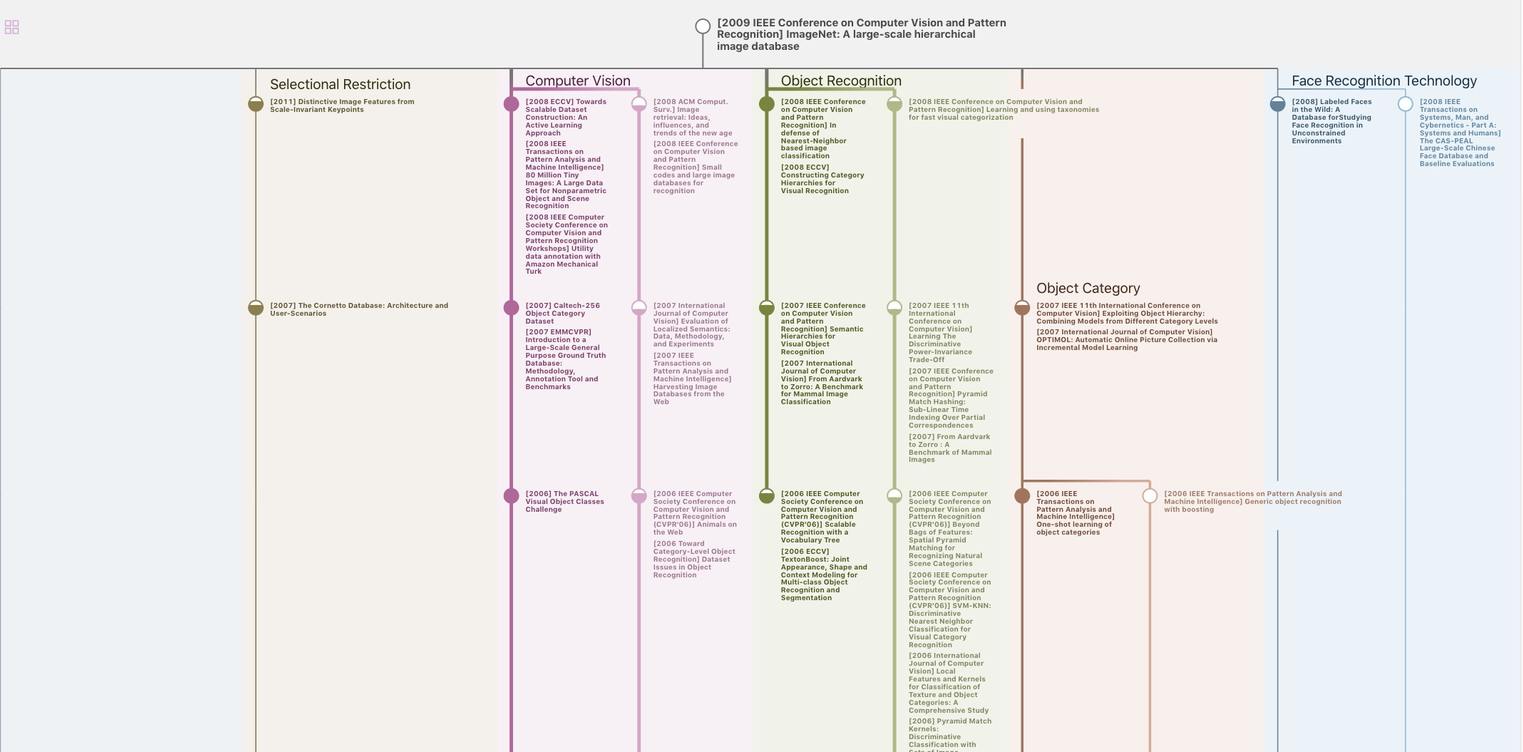
生成溯源树,研究论文发展脉络
Chat Paper
正在生成论文摘要