A portfolio trading system using a novel pixel graph network for stock selection and a mean-CDaR optimization for portfolio rebalancing
APPLIED SOFT COMPUTING(2024)
摘要
Due to the complexity and dynamic nature of financial markets, portfolio management tasks require continuous adaptation with market intelligence. Furthermore, to make profitable trading decisions, it is essential to predict the future performance of the market. To that end, this study proposes a dynamic portfolio trading system in two stages: stock trend prediction and portfolio optimization. In the first stage, although many researchers proposed powerful classification models to predict BUY/SELL trading signals, some scholars only focused on converting time series' features into multichannel images without considering any spatial relationships between features. Considering the relational capacity of graphs, this study proposes a novel image-based classification model, called Pixel Graph Network (PGN), to analyze the constructed images with propagating information from all neighboring pixels, build up a representation of the images as special graphs, and get advantages of a graphbased learning algorithm. In the second stage, stocks with predicted BUY trading signals in the following days, are fed into the Mean-Conditional Drawdown at Risk (M-CDaR) optimization model to prevent any significant drawdown of the investment portfolio. Moreover, a hierarchical feature selection algorithm has been proposed, combined with a continuous trend labeling method to improve the efficiency of the training process. To demonstrate the superiority of our proposed PGN model in comparison with benchmarks, 18 stocks from the New York Stock Exchange (NYSE) are used for numerical experiments. The obtained results show the improvement and effectiveness of the proposed model in both stock trend prediction and portfolio optimization. In terms of financial performance, the proposed PGN+M-CDaR portfolio-based trading system achieves an average annual return of 112%, an average annual Sortino ratio of 10.78, and an average annual Sharpe ratio of 2.79 which are promising to achieve substantial profits while mitigating downside risk.
更多查看译文
关键词
Financial forecasting,Graph neural network,Image classification,Conditional drawdown at risk,Portfolio optimization
AI 理解论文
溯源树
样例
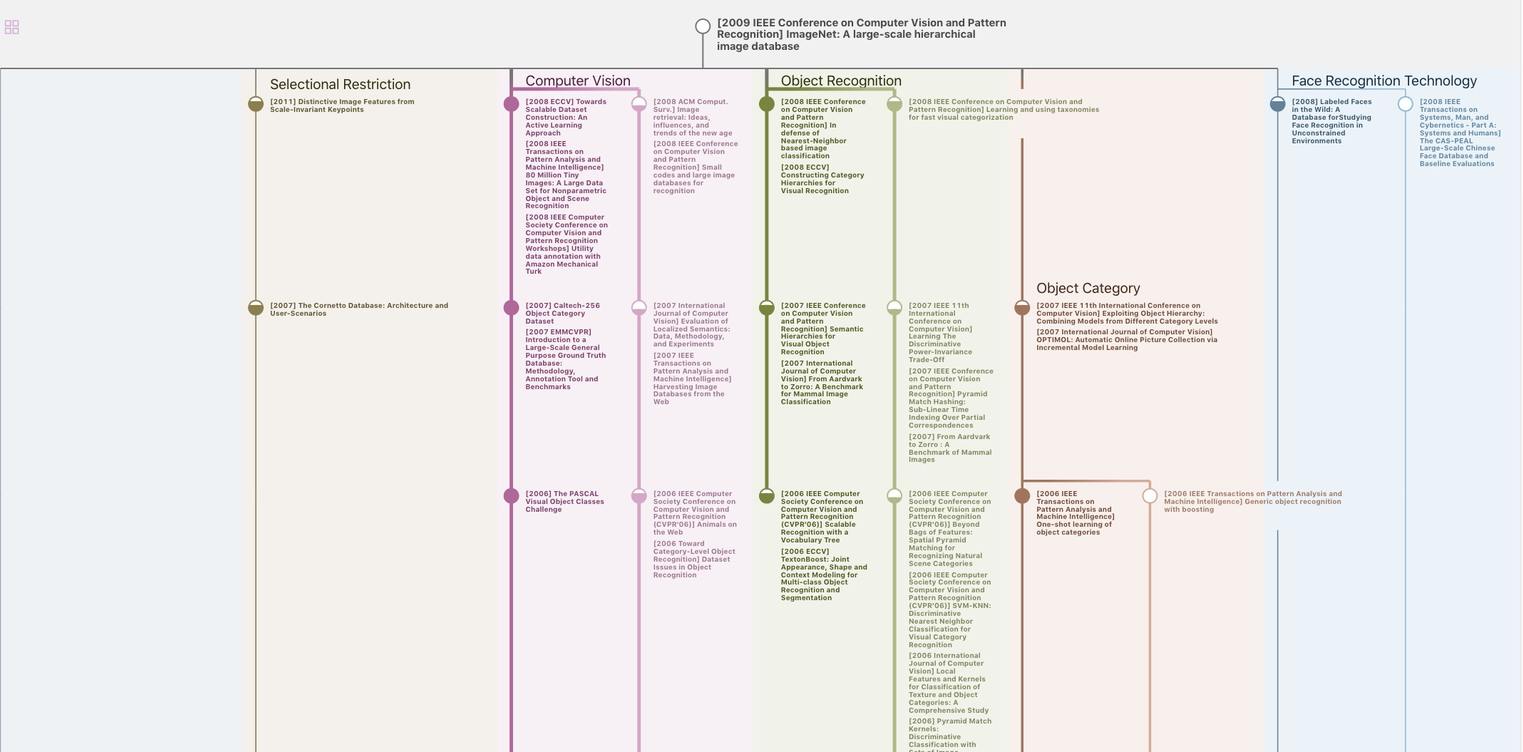
生成溯源树,研究论文发展脉络
Chat Paper
正在生成论文摘要