A Hausdorff Regression Paradigm for Interval Privacy
IEEE SIGNAL PROCESSING LETTERS(2024)
摘要
Data privacy has become a critical concern in today's data-driven world. Interval privacy emerges as a promising safeguard, representing private values as intervals. Traditional interval analysis methods, however, often rely on critical assumptions that are questionable in practice. To address this gap, we propose a novel paradigm for analyzing interval-valued data generated by the interval privacy mechanism. Our contributions are two-fold: First, we innovatively model intervals as random objects in a metric space and use the Hausdorff distance to quantify their dissimilarity without imposing restrictive assumptions. Second, as an application of our paradigm, we develop an interval-to-interval regression method named Hausdorff distance-based regression (HDBR), extending multivariate linear regression to metric spaces. The HDBR method estimates regression coefficients by minimizing the Hausdorff distance between the observed and estimated intervals. Simulation studies demonstrate the effectiveness and robustness of our proposed approach compared to mainstream competitors. We also provide a real data example to illustrate how to perform regression analysis within the interval privacy framework, and the results further validate the superiority of the HDBR method.
更多查看译文
关键词
Data privacy,Linear regression,Data models,Extraterrestrial measurements,Upper bound,Synthetic data,Predictive models,Hausdorff distance,interval data,interval privacy,linear regression model,metric space
AI 理解论文
溯源树
样例
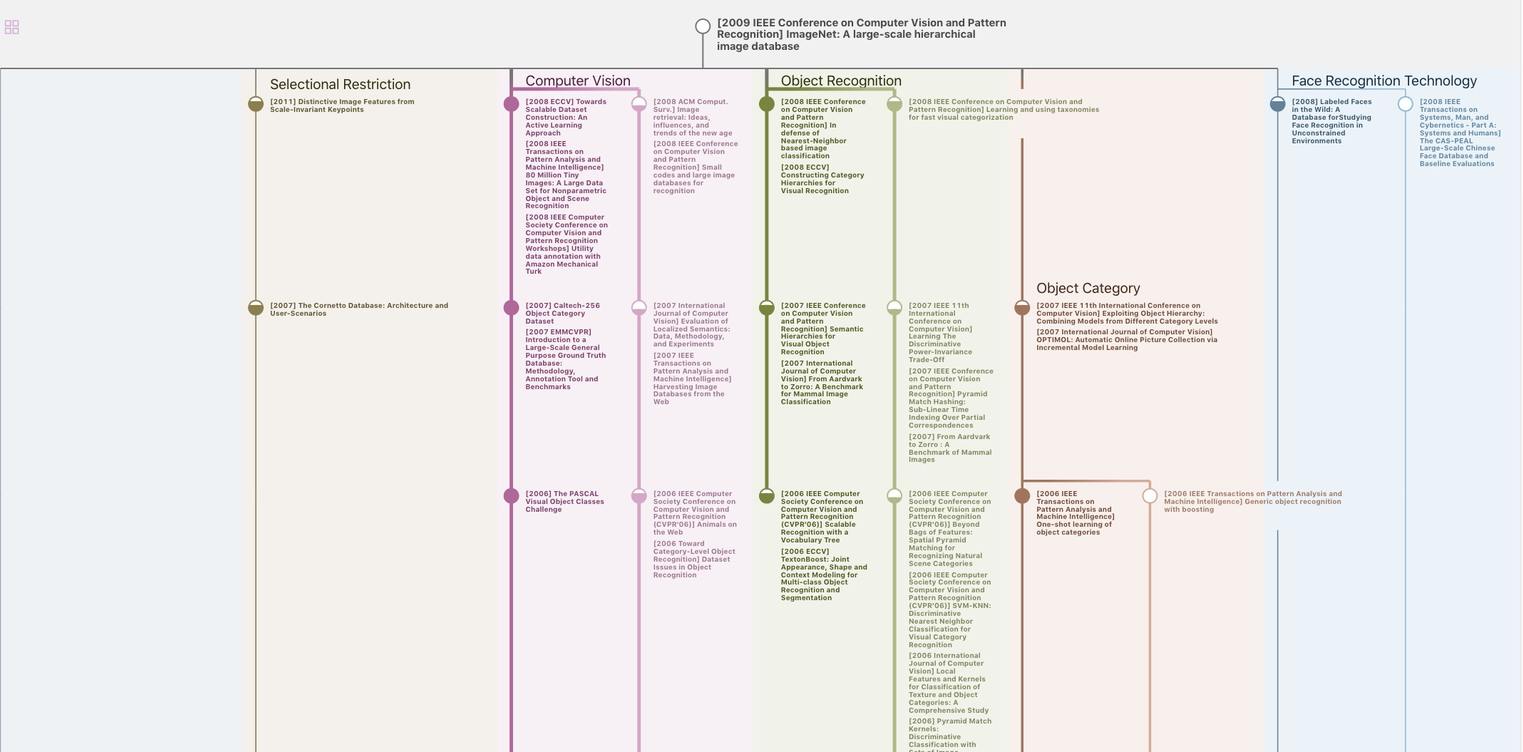
生成溯源树,研究论文发展脉络
Chat Paper
正在生成论文摘要