ML-TCAD: Accelerating FeFET Reliability Analysis Using Machine Learning
IEEE TRANSACTIONS ON ELECTRON DEVICES(2024)
摘要
Physics-based simulations using technology computer aided design (TCAD) offer high accuracy while suffering from exceedingly slow computations and significant license costs (especially when high parallelism is inevitable for Monte Carlo analysis), rendering large-scale design-space explorations infeasible. Therefore, we employ machine learning (ML) algorithms, trained on accurate datasets produced by TCAD, in order to massively accelerate multidomain ferroelectric FET (FeFET) simulations and take TCAD out of our framework's loop. Part of this study explores approaches to predicting I-V characteristics using pure ML means or augmenting it with simple physics models. The huge speedup (13 600x) obtained through our ML-based modeling enabled unprecedented analysis of device-to-device and cycle-to-cycle variability for FeFET technology. Furthermore, we demonstrate how computationally infeasible analysis that would take years using TCAD (e.g., read disturbance pulses with 200 million time steps) became feasible for the first time.
更多查看译文
关键词
Ferroelectric (FE),ferroelectric FET (FeFET),machine learning (ML),multidomain,reliability,technology computer aided design (TCAD),variation
AI 理解论文
溯源树
样例
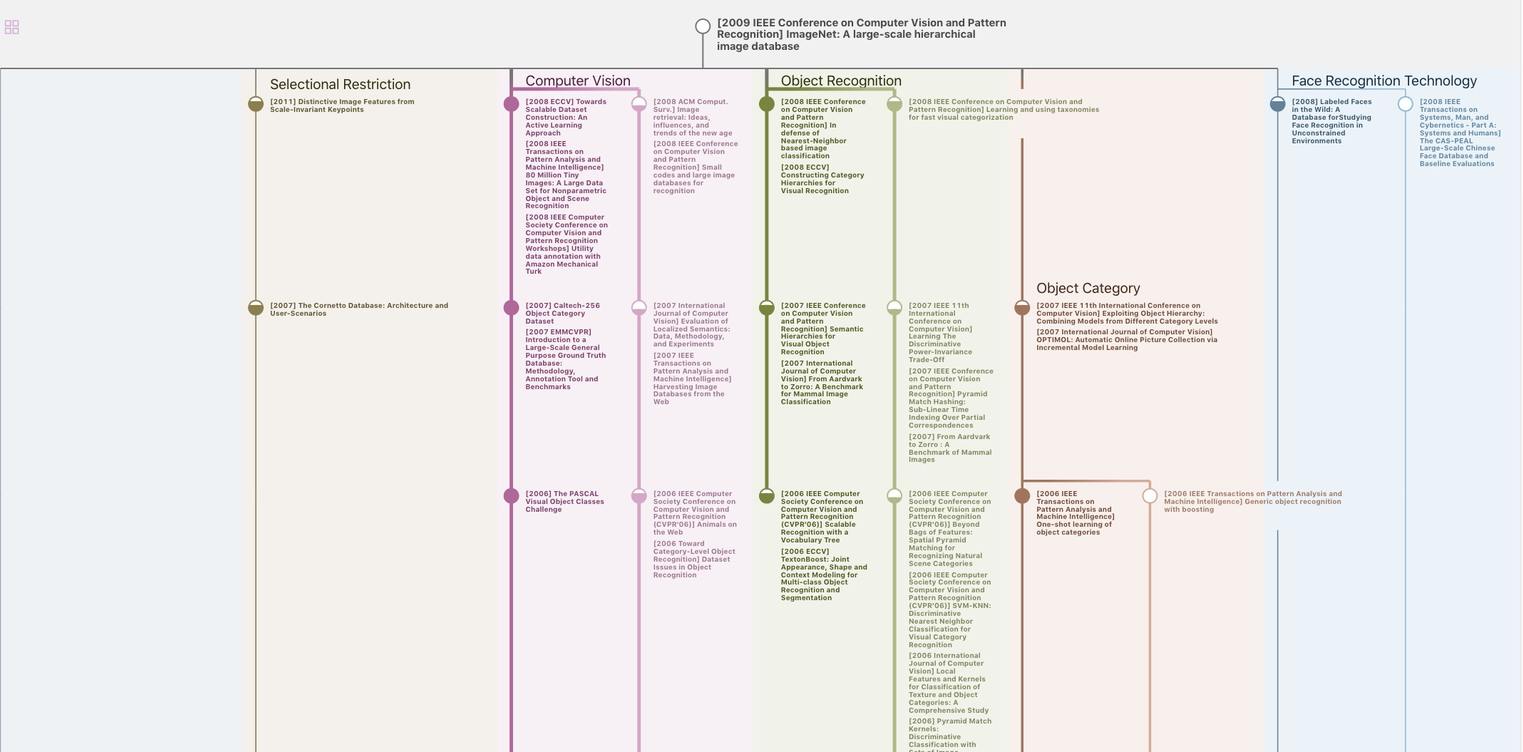
生成溯源树,研究论文发展脉络
Chat Paper
正在生成论文摘要