SSRL: Self-Supervised Spatial-Temporal Representation Learning for 3D Action Recognition
IEEE TRANSACTIONS ON CIRCUITS AND SYSTEMS FOR VIDEO TECHNOLOGY(2024)
摘要
For 3D action recognition, the main challenge is to extract long-range semantic information in both temporal and spatial dimensions. In this paper, in order to better excavate long-range semantic information from large number of unlabelled skeleton sequences, we propose Self-supervised Spatial-temporal Representation Learning (SSRL), a contrastive learning framework to learn skeleton representation. SSRL consists of two novel inference tasks that enable the network to learn global semantic information in the temporal and spatial dimensions, respectively. The temporal inference task learns the temporal persistence of human actions through temporally incomplete skeleton sequences. And the spatial inference task learns the spatially coordinated nature of human action through spatially partially skeleton sequence. We design two transformation modules to efficiently realize these two tasks while fitting the encoder network. To avoid the difficulty of constructing and maintaining high-quality negative samples, our proposed framework learns by maintaining consistency among positive samples without the need of any negative sample. Experiments demonstrate that our proposed method can achieve better results in comparison with state-of-the-art methods under a variety of evaluation protocols on NTU RGB+D 60, PKU-MMD and NTU RGB+D 120 datasets.
更多查看译文
关键词
Self-supervised learning,contrastive learning,skeleton action recognition
AI 理解论文
溯源树
样例
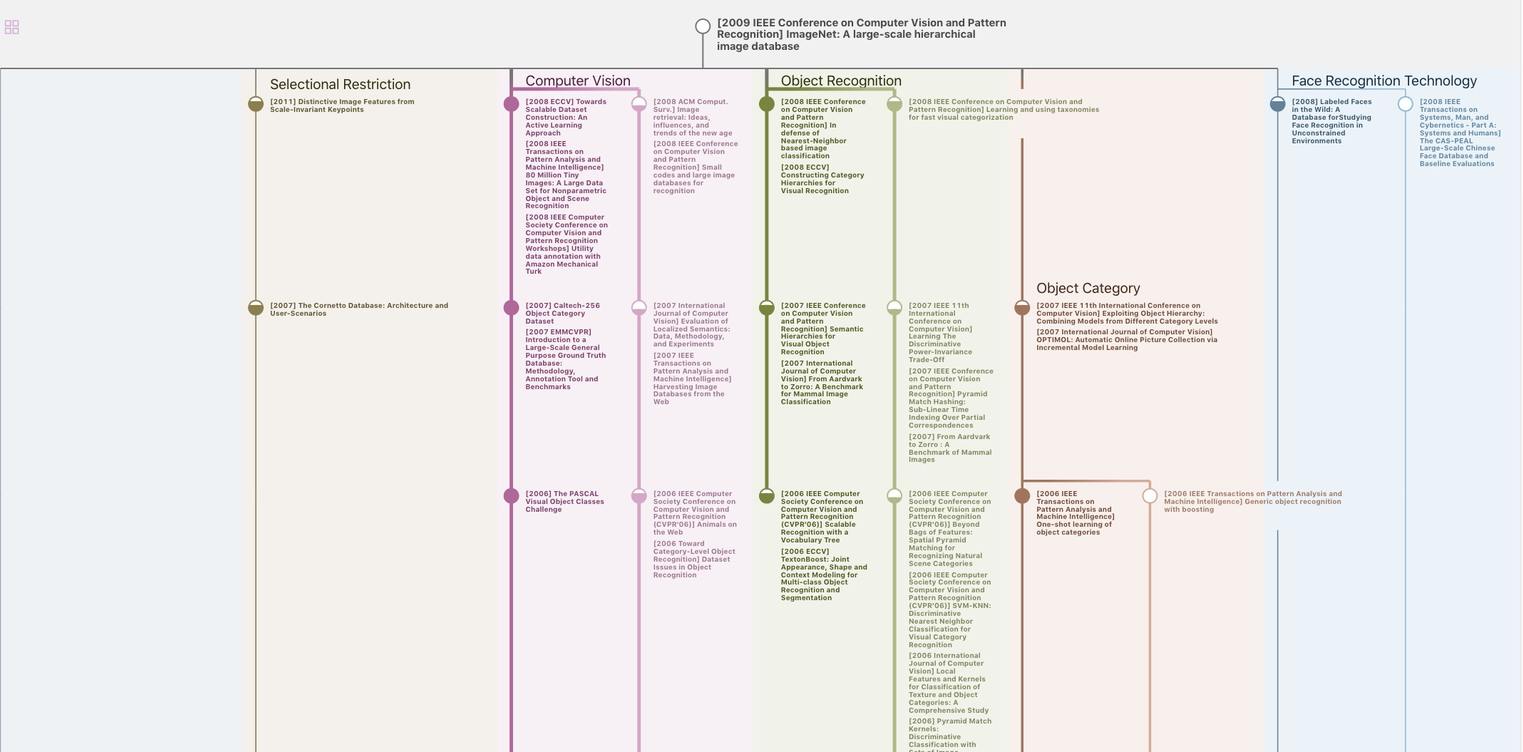
生成溯源树,研究论文发展脉络
Chat Paper
正在生成论文摘要