A deep transfer learning approach to construct the allowable load space of notched composite laminates
COMPOSITES SCIENCE AND TECHNOLOGY(2024)
摘要
Allowable load is one of the core parameters for the design and service of composite structures (CSs). However, due to the large design parameters, cumbersome design procedure and complicated simulation model of CSs, considerable amounts of experiments and simulations are needed to determine the allowable load of CSs, which is costly and inefficient, hindering the wide application of CSs in industry. In this work, we present a general deep transfer learning approach to construct the allowable load space (ALS) of notched laminates by considering various design parameters such as geometries, materials, stacking angles, ply numbers, laminate types and load types. Initially, an ensemble deep neural network (Ensemble) is trained, which can predict the allowable loads of notched laminate with known design parameters well. Here the Ensemble guided by ensemble learning is trained to reduce the errors generated by manually setting. Then, the pre-trained Ensemble is transferred to new design parameters by fine-tuning it with scarce new samples. Finally, the ALS of notch laminates is constructed by integrating the pre-trained and fine-tuned models, covering both known and diverse new design parameters. Our approach can easily be extended to other CSs.
更多查看译文
关键词
Composite structures,Allowable load space,Deep transfer learning,Double-double laminate,Legacy quad laminate
AI 理解论文
溯源树
样例
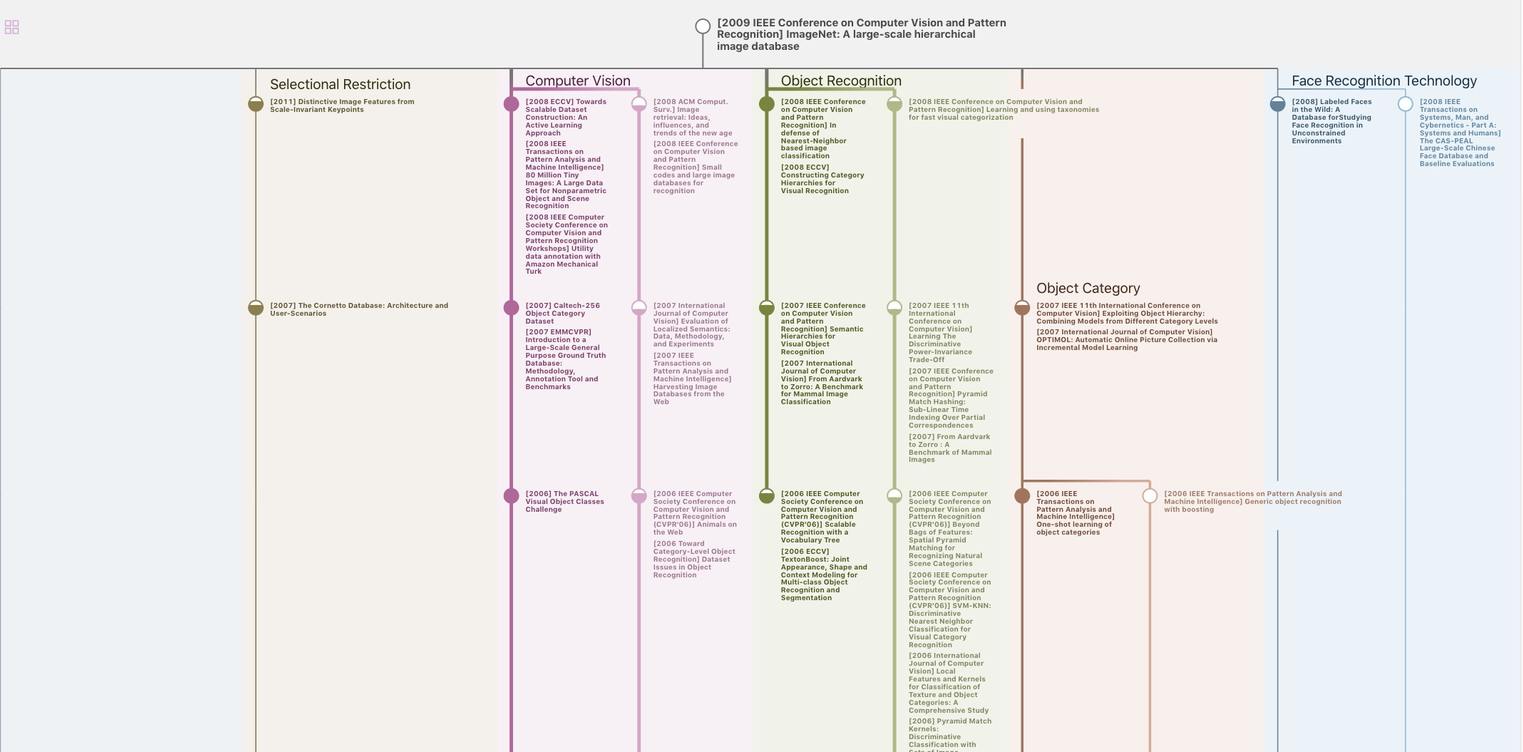
生成溯源树,研究论文发展脉络
Chat Paper
正在生成论文摘要