A hybrid Machine Learning unmixing method for automatic analysis of γ-spectra with spectral variability
Nuclear Instruments and Methods in Physics Research Section A: Accelerators, Spectrometers, Detectors and Associated Equipment(2024)
摘要
Automatic identification and quantification of γ-emitting radionuclides, taking into account spectral deformations due to γ-interactions in radioactive source surroundings, is a challenging task in the nuclear field. In that context, this paper presents a Machine Learning approach based on autoencoder that can learn a model for the spectral signatures of γ-emitters with variability. Training and test datasets were obtained by means of simulated γ-spectra computed with the Geant4 simulation code according to increasing material thicknesses (steel, lead). A novel hybrid unmixing algorithm combining a pretrained autoencoder is studied for joint estimation of spectral signatures and counting in the case of mixtures of four radionuclides (57Co, 60Co, 133Ba, 137Cs). The investigations were carried out to account for spectral deformations due to attenuation, Compton scattering and fluorescence at high and low statistics. This study demonstrates the validity of this novel hybrid approach combining Machine Learning and Maximum Likelihood for the automatic full-spectrum analysis of γ-spectra.
更多查看译文
关键词
Gamma-ray spectrometry,Spectral variability,Hybrid algorithm,Machine learning,Interpolating autoencoder,Semi-blind unmixing
AI 理解论文
溯源树
样例
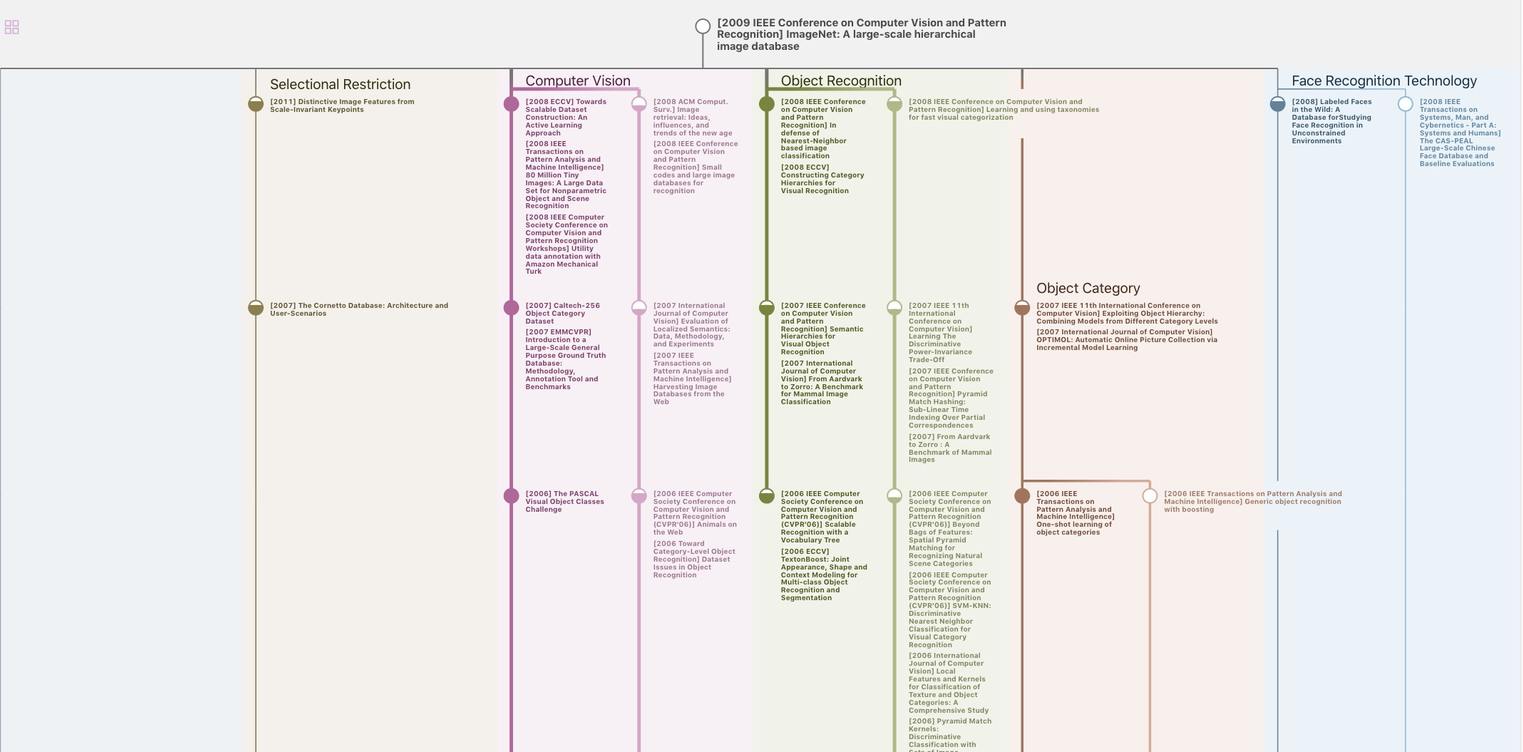
生成溯源树,研究论文发展脉络
Chat Paper
正在生成论文摘要