Assessing the influence of sugarcane bagasse ash for the production of eco-friendly concrete: Experimental and machine learning approaches
CASE STUDIES IN CONSTRUCTION MATERIALS(2024)
摘要
The innovative utilization of sugarcane bagasse ash (SCBA) in producing lightweight concrete (LWC) needs to be explored experimentally and quantification with machine learning approaches. Therefore, this study aims to investigate fresh, mechanical and microstructural characteristics and machine learning modeling of incorporating SCBA as a partial substitute of cement. In this investigation, OPC was replaced partially by the weight of SCBA in the proportion of 0 %, 5 %, 10 %, 15 %, and 20 %. The influences of SCBA on the fresh characteristics of concrete were investigated through tests of the slump, compacting factor, Kelly ball, k-slump, density, and air content. In addition, for hardened properties compressive, splitting tensile, flexural strength, and static modulus of elasticity were tested at 7 and 28 days. Furthermore, a microstructural characteristic was carried out for varying content of SCBA. Different machine learning (ML) predictive models were performed utilizing artificial neural network (ANN) and random forest (RF), to predict the fresh and mechanical attributes of the LWC. It was noticed that the workability value of fresh concrete was enhanced almost by 40 % to 50 % with the incorporation of SCBA content. The hardened test results revealed that a mixture having SCBA up to 10 % content SCBA resulted in nearly 10 % to 30 % better performance to satisfy the requirement of LWC. Regarding the sustainability assessment, the less embodied CO2 (eCO2) is produced in SCBA mixes, whereas it is also economically beneficial to produce SCBA mixes. Models' efficacy was evaluated including mean absolute error (MAE), root mean squared error (RMSE), and coefficient of determination (R2). The maximum R2 and minimum RMSE came across for RF (0.989 and 1.393, respectively) which provides the well estimation of the model.
更多查看译文
关键词
Fresh properties,Hardened properties,Lightweight concrete,Machine learning,Sugarcane bagasse ash,Sustainability
AI 理解论文
溯源树
样例
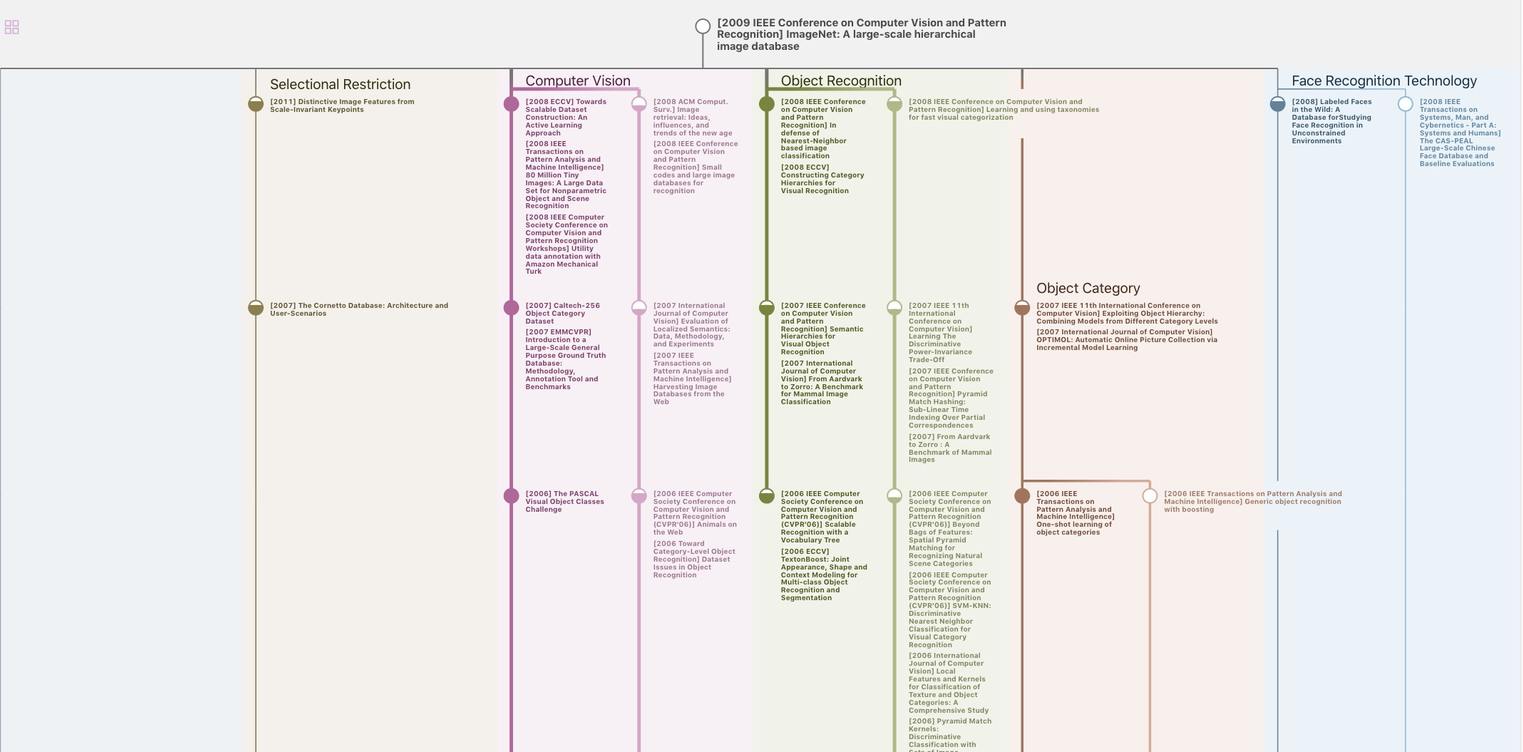
生成溯源树,研究论文发展脉络
Chat Paper
正在生成论文摘要