WIP: Using Generative AI to Assist in Individual Performance Feedback for Engineering Student Teams.
2023 IEEE Frontiers in Education Conference (FIE)(2023)
摘要
Teamwork is a critical component of engineering education, established as both an ABET competency and recognized by engineers in the workforce as one of the essential skills to their work. As a result, team-based learning throughout engineering education has become increasingly popular, especially in first-year programs. Tools such as CATME (Comprehensive Assessment of Team Member Effectiveness), a web-based program used by instructors, help instructors form student teams based on predefined criteria, facilitate peer evaluations to assess individual contributions and performance, and gather general feedback on student teamwork experiences. Other instructors may collect peer feedback through other methods, such as submitted assignments. Theories in organizational psychology highlight the relationship between performance feedback, psychological safety, and conflict management. To purposefully help students develop essential teamwork skills, such as how to manage conflict and build inclusive, psychologically safe teams effectively, it is important that they receive individual performance feedback. However, negative, unfiltered feedback from peers may also escalate unhealthy team dynamics. Consequently, instructors can be hesitant to share peer evaluation scores and comments with students because students may not be comfortable sharing honest, authentic feedback with their peers. However, withholding this valuable formative feedback to students may come at the expense of their individual professional development, especially in devel-oping teamwork skills. Additionally, these project-based learning (PBL) courses tend to be large, and summarizing formative feedback for individual students based on peer evaluations can be time-consuming and perhaps unrealistic with increasing class sizes. To address this need, we present the use of a generative artificial intelligence (AI) system to create individual performance summaries based on student peer responses. The authors present preliminary work from a pilot study that uses generative AI to produce individual performance summaries using CATME data from a first-year engineering course at a large public university in the mid-Atlantic region of the US. We share a sample output performance summary using a generative AI tool, GPT-4 specifically. Our initial findings indicate AI tools, such as GPT-4, can serve as a solution to provide more confidentiality in peer comments by effectively summarizing peer comments and producing individual performance feedback reviews. This study provides promising initial findings and recommendations on how AI can be leveraged to automate a student feedback process for instructors, allowing students to receive individual performance feedback while also providing an additional layer of confidentiality to support team dynamics and not compromise honest individual assessments.
更多查看译文
关键词
AI,feedback,peer comments,teamwork
AI 理解论文
溯源树
样例
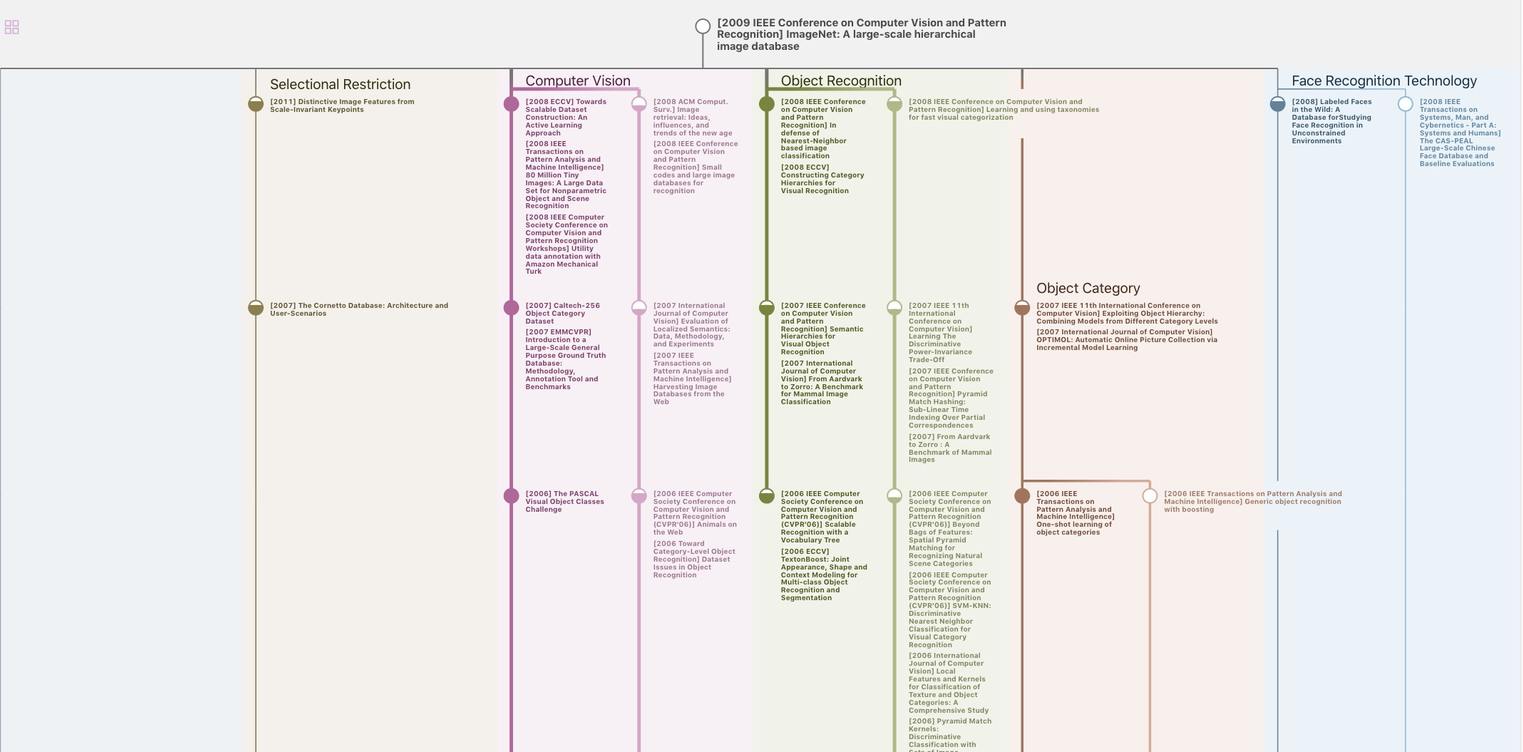
生成溯源树,研究论文发展脉络
Chat Paper
正在生成论文摘要