Deep Learning Algorithm of the SPARCC Scoring System in SI Joint MRI.
Journal of magnetic resonance imaging(2024)
摘要
Background: The Spondyloarthritis Research Consortium of Canada (SPARCC) scoring system is a sacroiliitis grading system.Purpose: To develop a deep learning-based pipeline for grading sacroiliitis using the SPARCC scoring system.Study Type: Prospective.Population: The study included 389 participants (42.2-year-old, 44.6% female, 317/35/37 for training/validation/testing). A pretrained algorithm was used to differentiate image with/without sacroiliitis.Field Strength/Sequence: 3-T, short tau inversion recovery (STIR) sequence, fast spine echo.Assessment: The regions of interest as ground truth for models' training were identified by a rheumatologist (HYC, 10-year-experience) and a radiologist (KHL, 6-year-experience) using the Assessment of Spondyloarthritis International Society definition of MRI sacroiliitis independently. Another radiologist (YYL, 4.5-year-experience) solved the discrepancies. The bone marrow edema (BME) and sacroiliac region models were for segmentation. Frangi-filter detected vessels used as intense reference. Deep learning pipeline scored using SPARCC scoring system evaluating presence and features of BMEs. A rheumatologist (SCWC, 6-year-experience) and a radiologist (VWHL, 14-year-experience) scored using the SPARCC scoring system once. The radiologist (YYL) scored twice with 5-day interval.Statistical Tests: Independent samples t-tests and Chi-squared tests were used. Interobserver and intraobserver reliability by intraclass correlation coefficient (ICC) and Pearson coefficient evaluated consistency between readers and the deep learning pipeline. We evaluated the performance using sensitivity, accuracy, positive predictive value, and Dice coefficient. A P-value <0.05 was considered statistically significant.Results: The ICC and the Pearson coefficient between the SPARCC scores from three readers and the deep learning pipeline were 0.83 and 0.86, respectively. The sensitivity in identifying BME and accuracy of identifying SI joints and blood vessels was 0.83, 0.90, and 0.88, respectively. The dice coefficients were 0.82 (sacrum) and 0.80 (ilium).Data Conclusion: The high consistency with human readers indicated that deep learning pipeline may provide a SPARCC-informed deep learning approach for scoring of STIR images in spondyloarthritis.
更多查看译文
关键词
deep learning,sacroiliitis,STIR-MRI,SPARCC scoring system
AI 理解论文
溯源树
样例
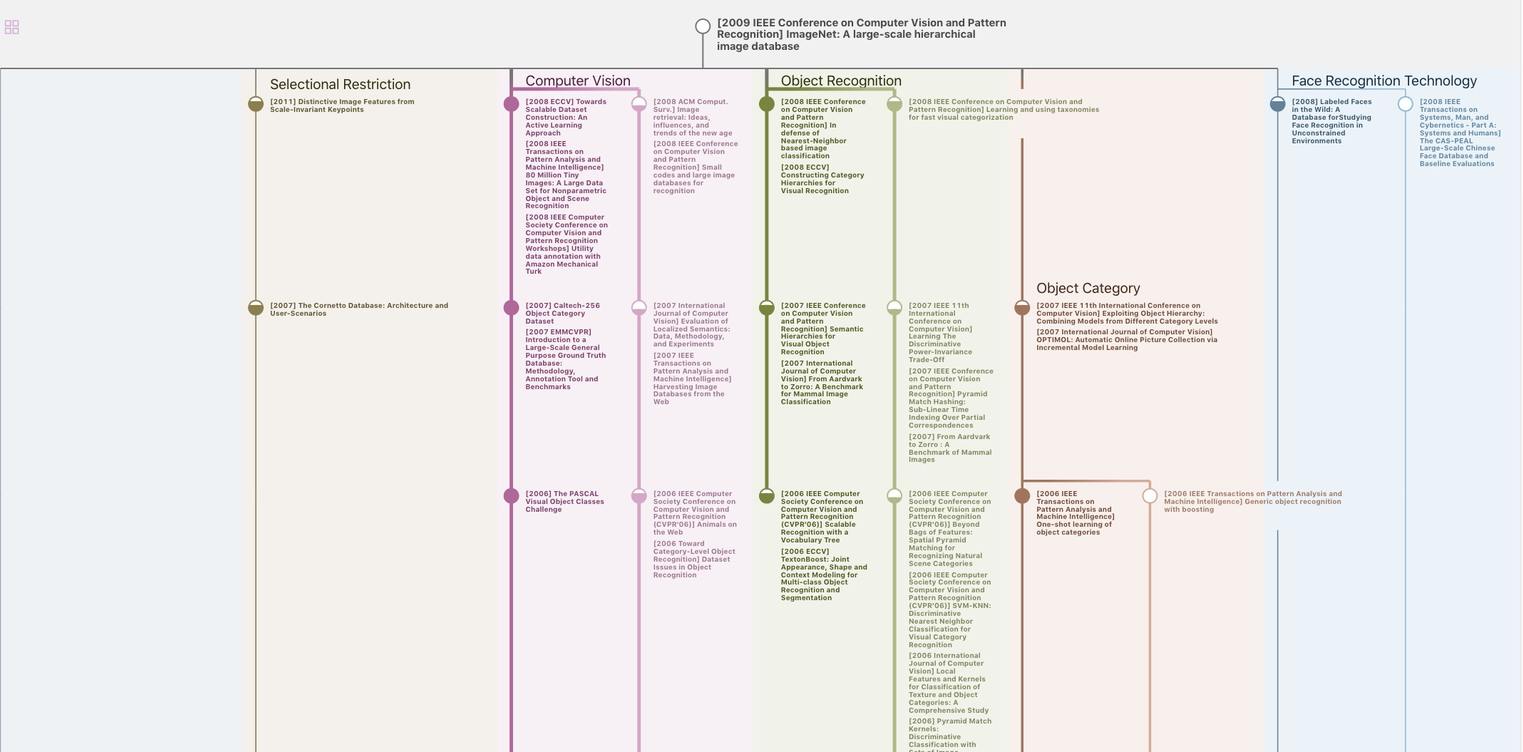
生成溯源树,研究论文发展脉络
Chat Paper
正在生成论文摘要