Unbiased construction of constitutive relations for soft materials from experiments via rheology-informed neural networks
PROCEEDINGS OF THE NATIONAL ACADEMY OF SCIENCES OF THE UNITED STATES OF AMERICA(2024)
摘要
The ability to concisely describe the dynamical behavior of soft materials through closed-form constitutive relations holds the key to accelerated and informed design materials and processes. The conventional approach is to construct constitutive relations through simplifying assumptions and approximating the time-and rate dependent stress response of a complex fluid to an imposed deformation. While traditional frameworks have been foundational to our current understanding of soft materials, they often face a twofold existential limitation: i) Constructed on ideal and generalized assumptions, precise recovery of material-specific details is usually serendipitous, if possible, and ii) inherent biases that are involved by making those assumptions commonly come at the cost of new physical insight. This work introduces approach by leveraging recent advances in scientific machine learning methodologies discover the governing constitutive equation from experimental data for complex fluids. Our rheology-informed neural network framework is found capable of learning hidden rheology of a complex fluid through a limited number of experiments. This followed by construction of an unbiased material-specific constitutive relation that accurately describes a wide range of bulk dynamical behavior of the material. While extremely efficient in closed-form model discovery for a real-world complex system, model also provides insight into the underpinning physics of the material.
更多查看译文
关键词
data-driven physics discovery,rheology-informed neural networks,soft matter modeling
AI 理解论文
溯源树
样例
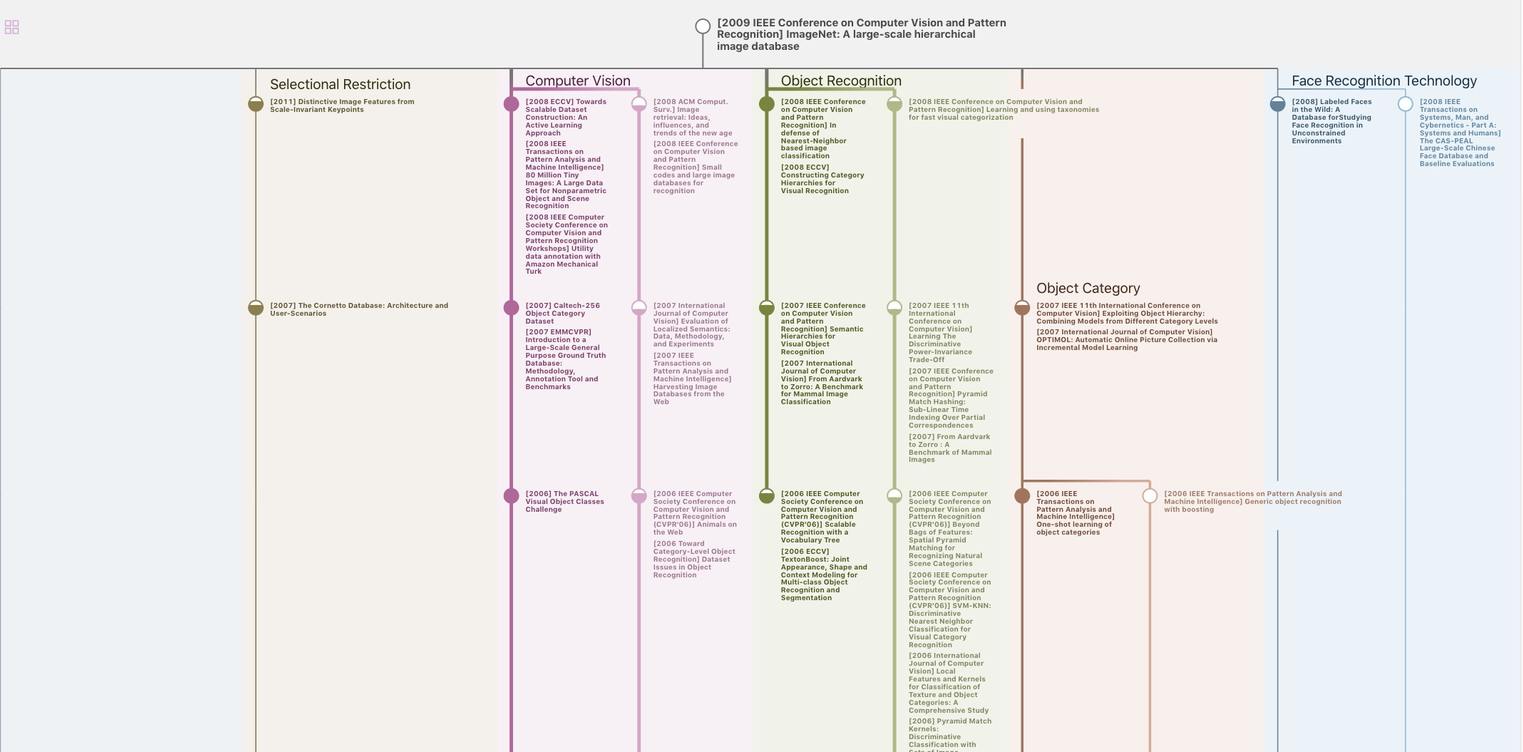
生成溯源树,研究论文发展脉络
Chat Paper
正在生成论文摘要