Superpixels Meet Essential Spectra for Fast Raman Hyperspectral Microimaging.
OPTICS EXPRESS(2024)
Abstract
In the context of spectral unmixing, essential information corresponds to the most linearly dissimilar rows and/or columns of a two-way data matrix which are indispensable to reproduce the full data matrix in a convex linear way. Essential information has recently been shown accessible on-the-fly via a decomposition of the measured spectra in the Fourier domain and has opened new perspectives for fast Raman hyperspectral microimaging. In addition, when some spatial prior is available about the sample, such as the existence of homogeneous objects in the image, further acceleration for the data acquisition procedure can be achieved by using superpixels. The expected gain in acquisition time is shown to be around three order of magnitude on simulated and real data with very limited distortions of the estimated spectrum of each object composing the images.
MoreTranslated text
Key words
Hyperspectral Imaging,In Vivo Imaging,Infrared Spectroscopy,High-Resolution Imaging,Near-Infrared Spectroscopy
求助PDF
上传PDF
View via Publisher
AI Read Science
Must-Reading Tree
Example
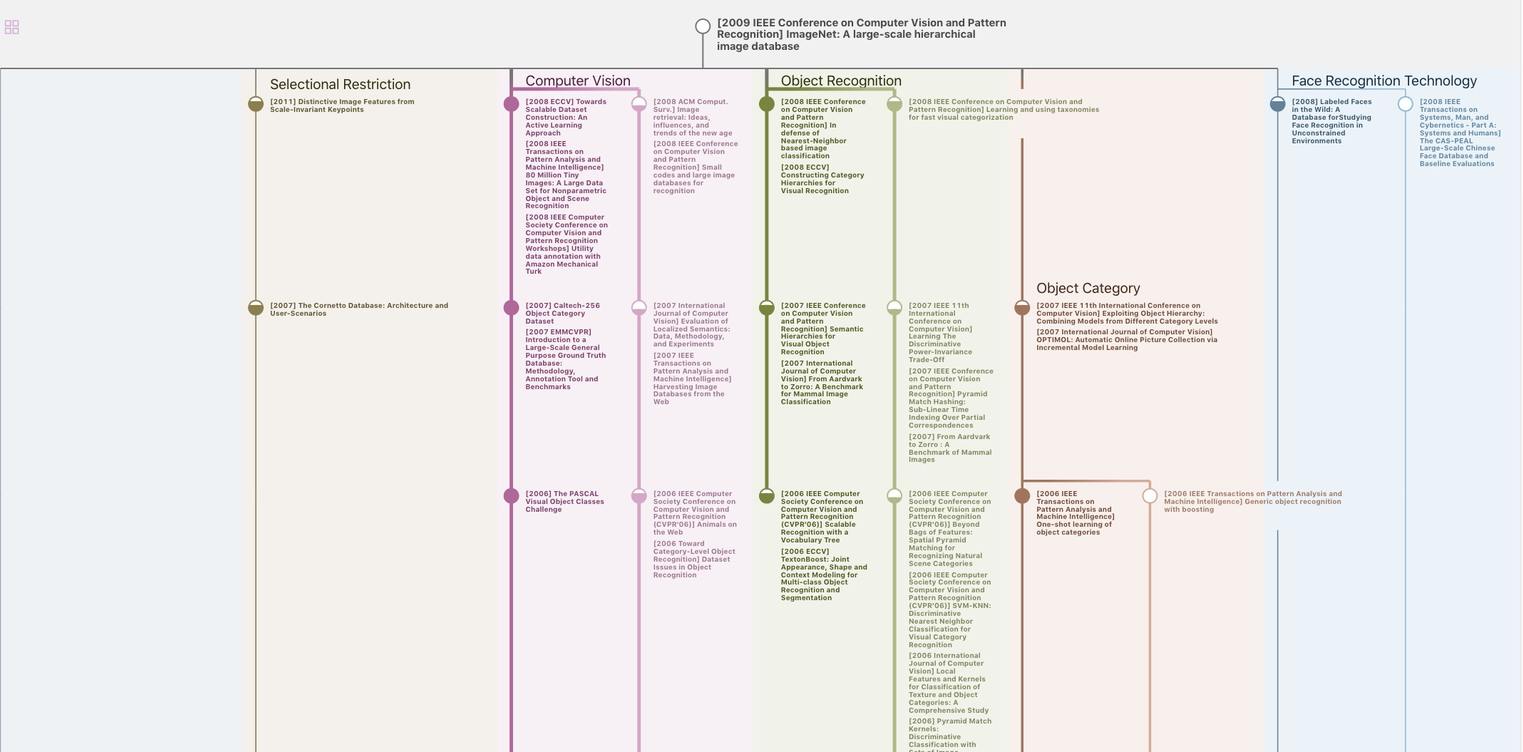
Generate MRT to find the research sequence of this paper
Data Disclaimer
The page data are from open Internet sources, cooperative publishers and automatic analysis results through AI technology. We do not make any commitments and guarantees for the validity, accuracy, correctness, reliability, completeness and timeliness of the page data. If you have any questions, please contact us by email: report@aminer.cn
Chat Paper
Summary is being generated by the instructions you defined