Efficient Parameter Optimisation for Quantum Kernel Alignment: A Sub-sampling Approach in Variational Training
CoRR(2024)
摘要
Quantum machine learning with quantum kernels for classification problems is
a growing area of research. Recently, quantum kernel alignment techniques that
parameterise the kernel have been developed, allowing the kernel to be trained
and therefore aligned with a specific dataset. While quantum kernel alignment
is a promising technique, it has been hampered by considerable training costs
because the full kernel matrix must be constructed at every training iteration.
Addressing this challenge, we introduce a novel method that seeks to balance
efficiency and performance. We present a sub-sampling training approach that
uses a subset of the kernel matrix at each training step, thereby reducing the
overall computational cost of the training. In this work, we apply the
sub-sampling method to synthetic datasets and a real-world breast cancer
dataset and demonstrate considerable reductions in the number of circuits
required to train the quantum kernel while maintaining classification accuracy.
更多查看译文
AI 理解论文
溯源树
样例
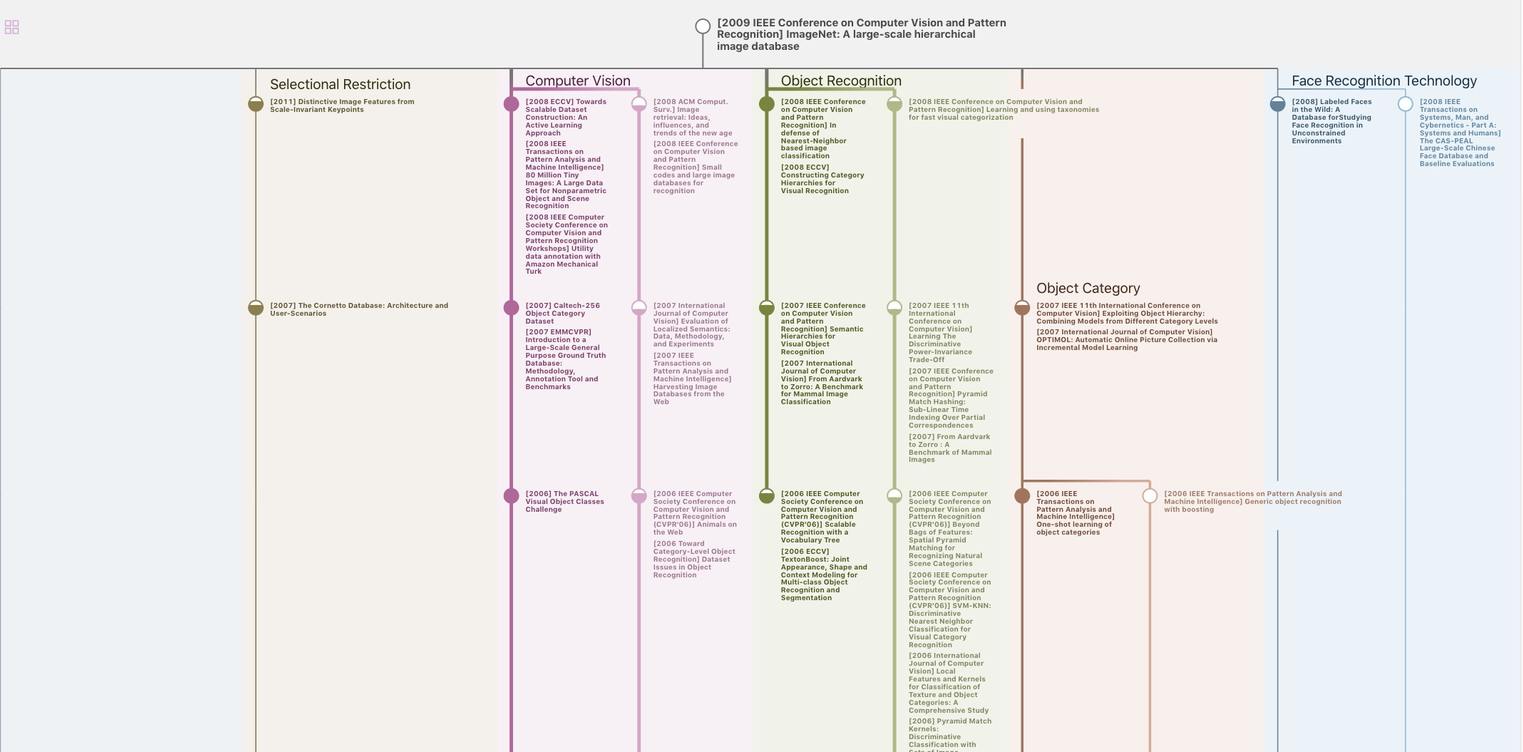
生成溯源树,研究论文发展脉络
Chat Paper
正在生成论文摘要