Approximation Algorithms for the Weighted Nash Social Welfare via Convex and Non-Convex Programs
CoRR(2024)
摘要
In an instance of the weighted Nash Social Welfare problem, we are given a
set of m indivisible items, 𝒢, and n agents, 𝒜,
where each agent i ∈𝒜 has a valuation v_ij≥ 0 for each
item j∈𝒢. In addition, every agent i has a non-negative weight
w_i such that the weights collectively sum up to 1. The goal is to find an
assignment σ:𝒢→𝒜 that maximizes
∏_i∈𝒜(∑_j∈σ^-1(i)
v_ij)^w_i, the product of the weighted valuations of the players.
When all the weights equal 1/n, the problem reduces to the classical Nash
Social Welfare problem, which has recently received much attention. In this
work, we present a 5·exp(2· D_KL(𝐰 || 1⃗/n)) = 5·exp(2logn + 2∑_i=1^n
w_i logw_i)-approximation algorithm for the weighted Nash Social
Welfare problem, where D_KL(𝐰 || 1⃗/n) denotes the KL-divergence between the distribution
induced by 𝐰 and the uniform distribution on [n].
We show a novel connection between the convex programming relaxations for the
unweighted variant of Nash Social Welfare presented in , and generalize the programs to two different mathematical
programs for the weighted case. The first program is convex and is necessary
for computational efficiency, while the second program is a non-convex
relaxation that can be rounded efficiently. The approximation factor derives
from the difference in the objective values of the convex and non-convex
relaxation.
更多查看译文
AI 理解论文
溯源树
样例
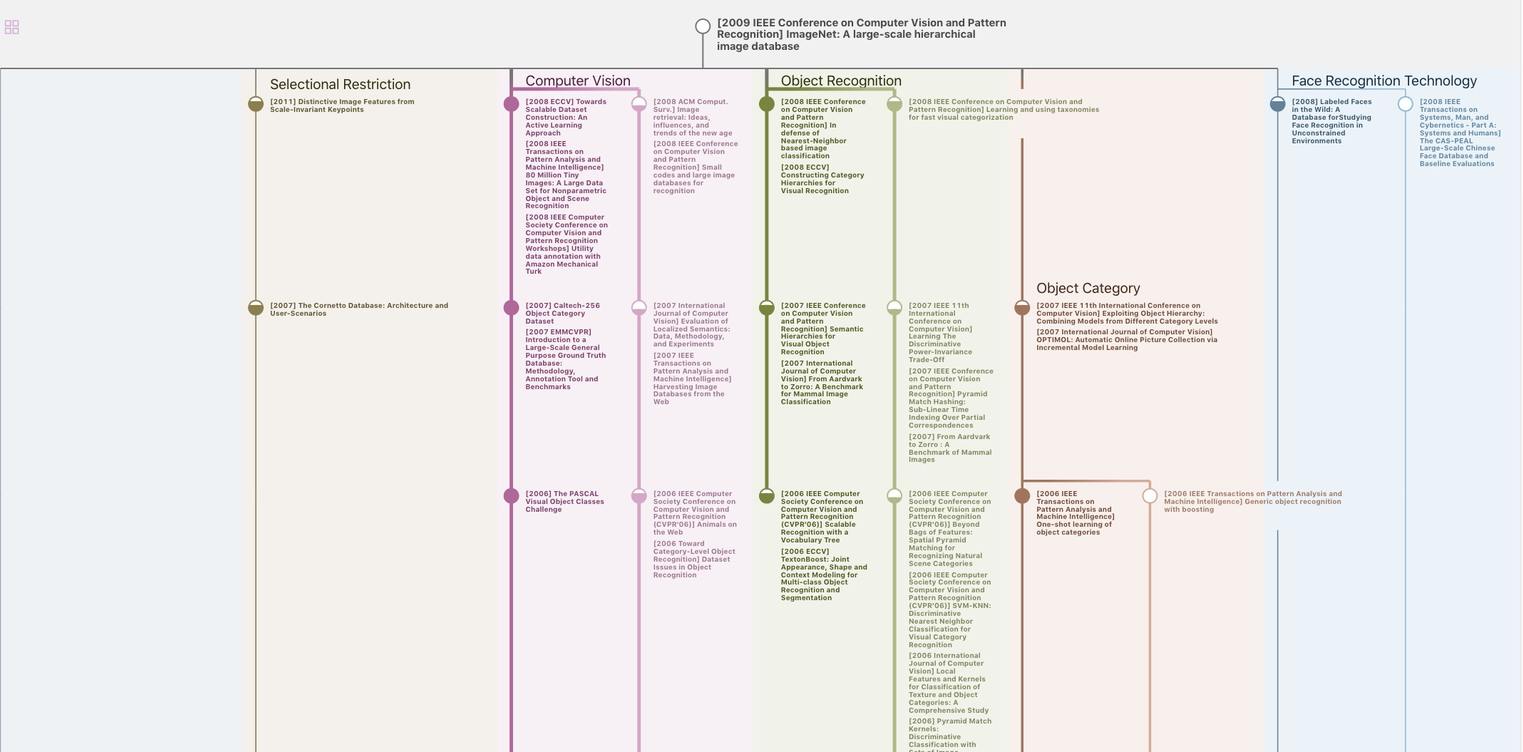
生成溯源树,研究论文发展脉络
Chat Paper
正在生成论文摘要