RL-NMT: Reinforcement Learning Fine-tuning for Improved Neural Machine Translation of Burmese Dialects.
ACM Multimedia Asia(2023)
摘要
In this study, we investigate the use of Reinforcement Learning (RL) for fine-tuning neural machine translation models for Burmese dialects. We perform experiments using two extremely low-resource Burmese dialect datasets: Burmese-Beik and Burmese-Rakhine, employing two different deep learning modeling techniques: bi-LSTM (Seq2Seq) and the Transformer. Our training procedure involved initially training models over varying numbers of epochs: 30, 40, 50, 60, and 70 epochs, and then fine-tuning them with RL for additional epochs, such that the total number of epochs for each model equaled 100. For instance, a model initially trained for 30 epochs was fine-tuned for an additional 70 epochs using RL. The results show that better quality machine translation was attained with RL over all initial models. Moreover, RL yields a significant improvement of Bilingual Evaluation Understudy (BLEU) scores (+4.73 BLEU for Burmese-to-Beik translation with Seq2Seq RL, +2.58 for Rakhine-to-Burmese translation with Transformer RL) over some of the baselines not utilizing RL training.
更多查看译文
AI 理解论文
溯源树
样例
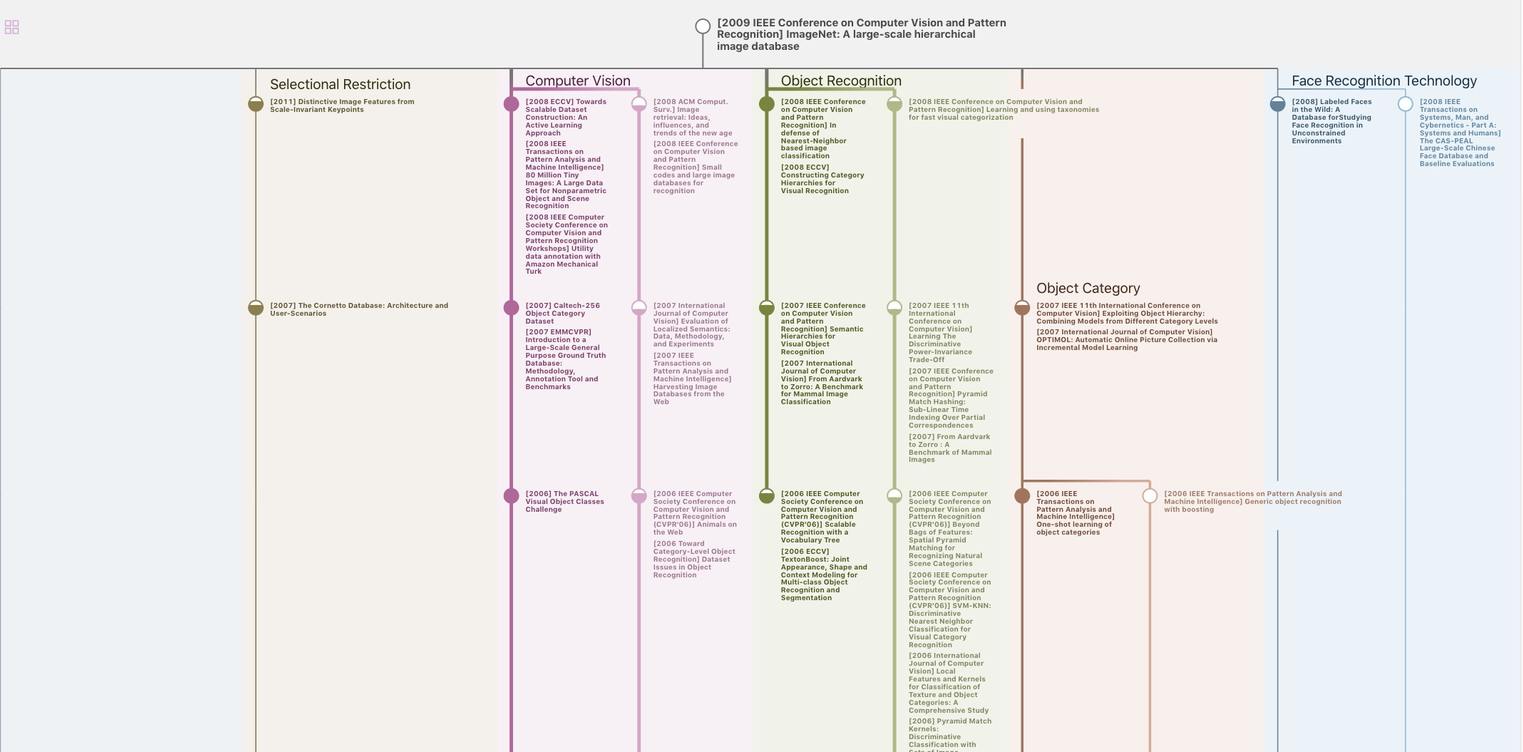
生成溯源树,研究论文发展脉络
Chat Paper
正在生成论文摘要