Relevance and Irrelevance Considered Subspace Mapping Neural Networks for Remote Sensing Text-Image Retrieval.
ACM Multimedia Asia(2023)
摘要
Remote sensing cross-modal image-text retrieval has attracted increasing attention due to its important roles in multiple domains. Existing methods perform salient modeling for the feature that has high relevance between different modalities. However, most works consider the relevance between different modalities but ignore the irrelevance between different modalities, resulting in incomplete modeling of the relevance and irrelevance between different modalities. In this paper, we propose a Relevance and Irrelevance Considered Subspace Mapping Neural Networks (RIR-SMNNs) to simultaneously consider the relevance and irrelevance between different modalities. Specifically, we first utilize Multiscale Image Feature Extraction (MIFE) and Multiscale Text Feature Extraction (MTFE) to extract the multiscale feature of image and text. Then, we perform Local Space Building Module (LSB), which constructs local space that realizes scale alignment. Finally, we perform the Relevance and Irrelevance Local Space Mapping (RIRLSM) to consider the relevance and irrelevance of different modalities in multiple spaces. Experimental results on several remote sensing datasets demonstrate our model outperforms the state-of-the-art approaches.
更多查看译文
AI 理解论文
溯源树
样例
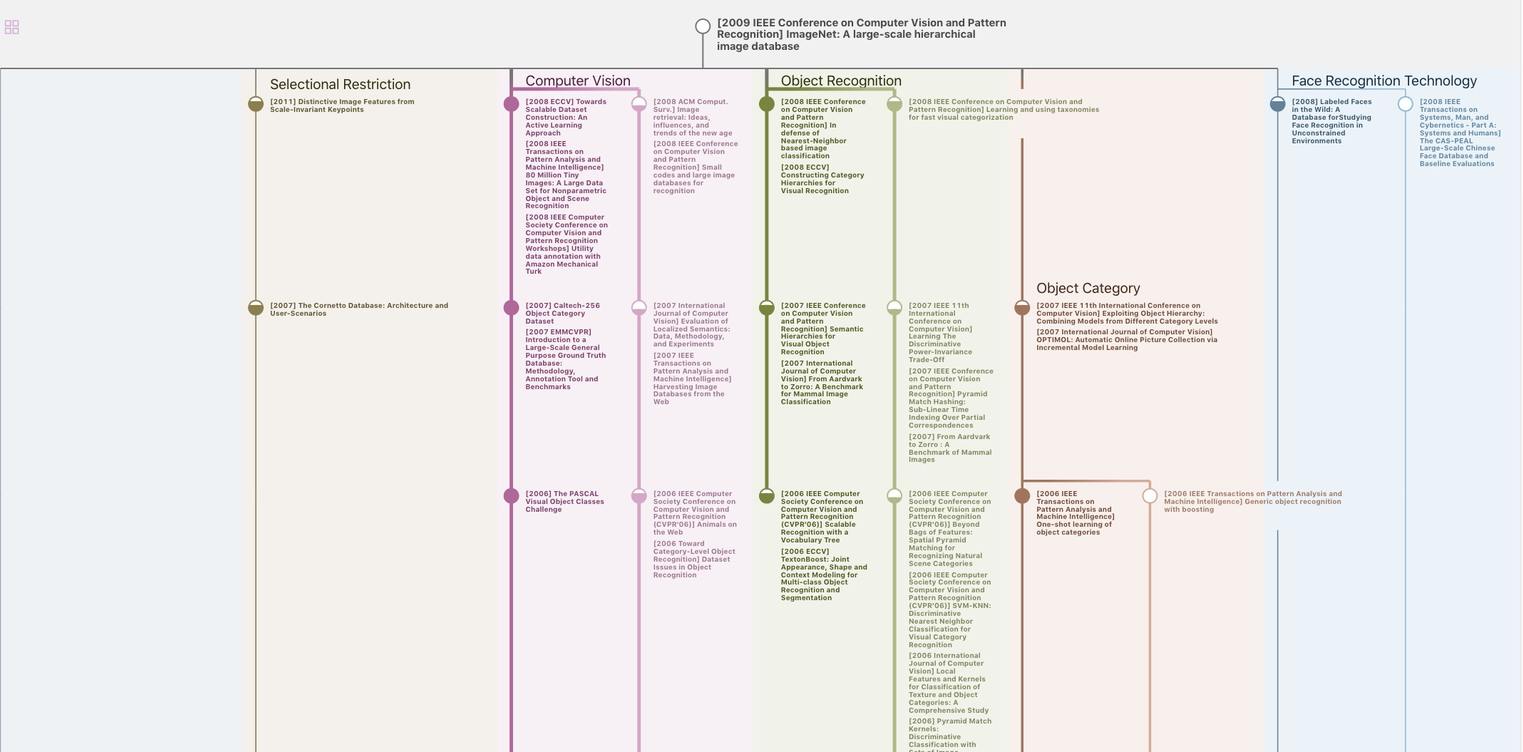
生成溯源树,研究论文发展脉络
Chat Paper
正在生成论文摘要