NuclSeg: nuclei segmentation using semi-supervised stain deconvolution.
ACM Multimedia Asia(2023)
摘要
Recently, deep learning-inferred stain deconvolution/separation-based nuclei segmentation works demonstrated significant results by translating low-cost and prevalent immunohistochemical (IHC) slides to more expensive-yet-informative multiplex immunofluorescence (mpIF) images. However, when the input stain style is changed to Hematoxylin and Eosin stain (H&E), which is one of the principal tissue stains used in histology, the stain deconvolution/separation based works can not achieve satisfactory performance because the features of the input stain image are greatly changed. To solve this problem, we integrate stain transfer (H&E->IHC) before stain deconvolution (IHC->mpIF) to revise the image style. Moreover, a new semi-supervised learning strategy collaborating supervised and unsupervised learning processes are employed to diversify training data content for stain deconvolution.Firstly, in the supervised learning process, generative adversarial network (GAN) based image-to-image mapping (stain deconvolution) and inverse mapping models are trained on the dataset of co-registered IHC staining and mpIF staining of the same slides to respectively convert IHC to mpIF (I2m), and mpIF to IHC (m2I). After that, in the unsupervised learning process, high-quality IHC images from m2I model are selected according to the Discriminator score on another unpaired dataset, and then, IHC images are paired with input mpIF as the unsupervised training data for further improving the I2m model. This semi-supervised scheme balances two supervised and unsupervised errors while optimizing to limit the effect of imperfect pseudo inputs but still enhance stain deconvolution. Furthermore, image enhancement is applied after the stain deconvolution model to obtain high-quality segmentation masks. We thoroughly evaluate our method on publicly available benchmark datasets. The results show the proposed model obtains significant improvement compared to the SOTAs.
更多查看译文
AI 理解论文
溯源树
样例
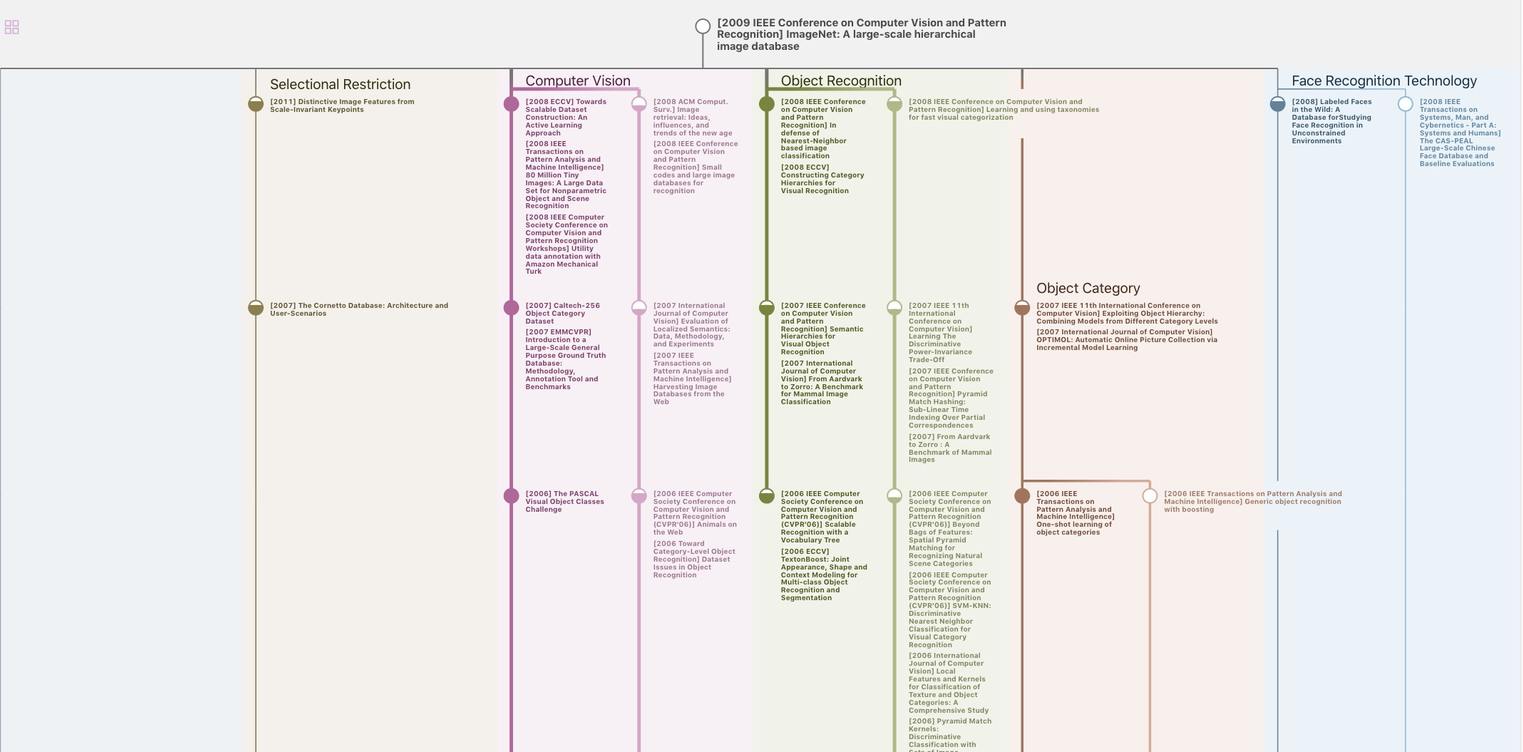
生成溯源树,研究论文发展脉络
Chat Paper
正在生成论文摘要