Targeted Transferable Attack against Deep Hashing Retrieval.
ACM Multimedia Asia(2023)
摘要
With the extensive utilization of deep hashing, there exists a surging interest in studying adversarial attacks against it. Previous methods have demonstrated the superior white-box attack performance against deep hashing. However, the more challenging and realistic targeted black-box attack has not yet been explored sufficiently, which will result in an over-estimation on model robustness. In this paper, we focus on targeted black-box attack based on transferability, and propose a novel Targeted Transferable Attack method against deep hashing with Generative Adversarial Network (TTA-GAN). Specifically, we first propose a new Iterative Anchor code Optimization (IAO) method to generate anchor code with superior representative semantics of target label, which can improve both targeted white-box and black-box performances. Then, we propose a generation-based method to directly generate targeted transferable adversarial example by training a conditional generator and a discriminator. Moreover, to further promote the targeted transferability, we conduct multiple input transformations on the generated adversarial example to alleviate the overfitting phenomenon on source model. Finally, we extend our method to a novel model ensemble attack method TTA-GANens to preserve the representative semantics on multiple models, specialized for deep hashing. Extensive experiments demonstrate the superior targeted black-box attack performance than the state-of-the-art methods.
更多查看译文
AI 理解论文
溯源树
样例
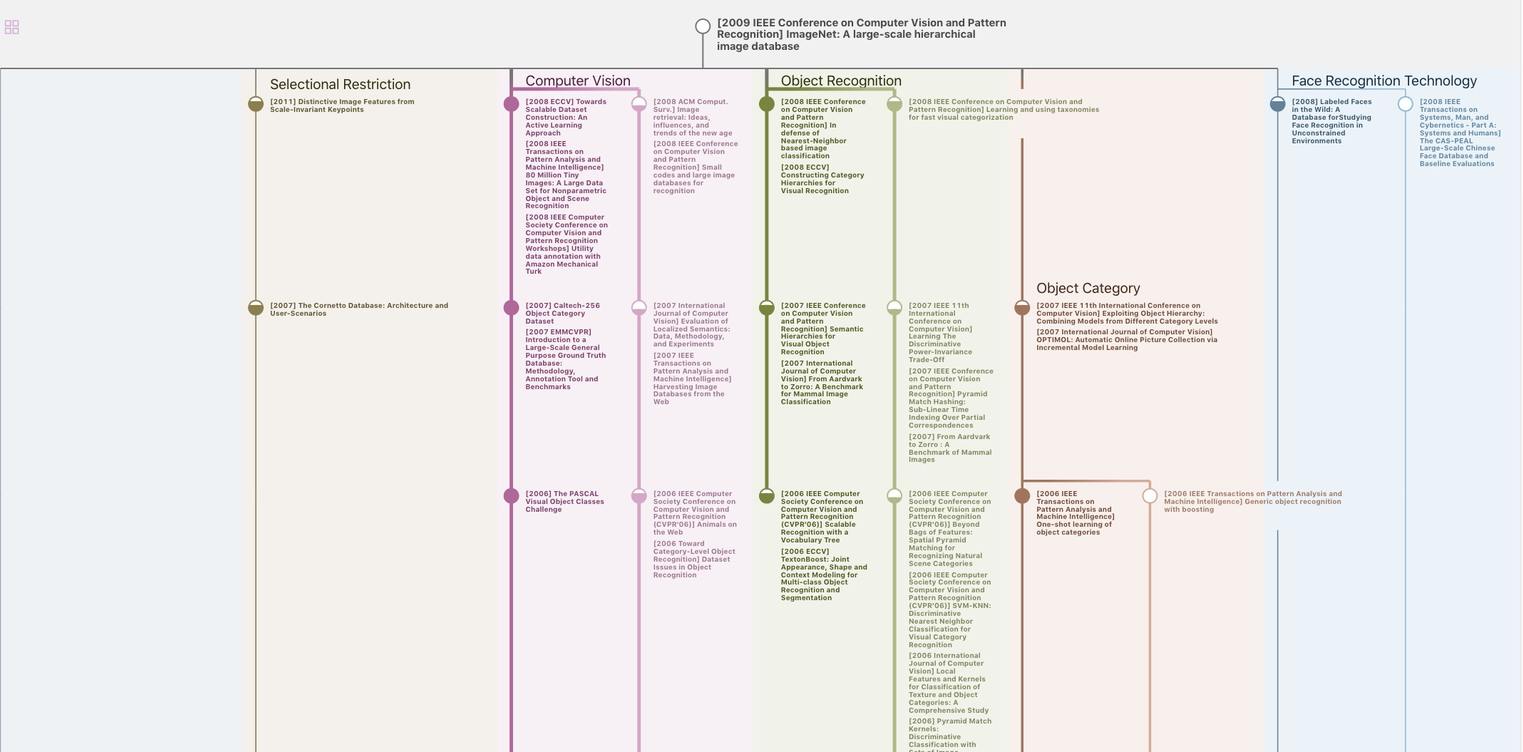
生成溯源树,研究论文发展脉络
Chat Paper
正在生成论文摘要