False Positive Reduction of Pulmonary Nodule on CT Image Using Attention-based Multiple Instance Learning.
20TH INTERNATIONAL CONFERENCE ON CONTENT-BASED MULTIMEDIA INDEXING, CBMI 2023(2023)
Univ Sci & Technol Hanoi | Univ Orleans
Abstract
Diagnosis and treatment of multiple pulmonary nodules are clinically essential but challenging. Pulmonary nodule detection is important in early lung cancer detection and diagnosis. False positive reduction (FPR) is a significant stage of pulmonary nodule detection systems. Prior investigations on nodule candidate classification use solitary-nodule approaches, which ignore the relations between nodules. In this study, we propose to use Attention-based Deep Multiple Instance Learning, a variation of supervised learning to recognize true pulmonary nodules among a large group of candidates proposed from the detection stage. By treating the multiple nodules from a different patient, critical relational information between solitary-nodule is extracted and empirically proves the benefit of learning the relations between multiple nodules. An attention layer trained with CNN to replace typical pooling-based aggregation in multiple instance learning (MIL). Experiments of lung nodule FPR on the public LUNA16 dataset validate the effectiveness of the proposed method. The proposed method achieved an accuracy of 99.6%, specificity of 100%, recall of 99.92%, and F1 score of 99.6%. The experimental results reveal that our method can achieve satisfactory performance in FPR.
MoreTranslated text
Key words
Multiple Instance Learning,false positive reduction,pulmonary nodules,CT images
求助PDF
上传PDF
View via Publisher
AI Read Science
AI Summary
AI Summary is the key point extracted automatically understanding the full text of the paper, including the background, methods, results, conclusions, icons and other key content, so that you can get the outline of the paper at a glance.
Example
Background
Key content
Introduction
Methods
Results
Related work
Fund
Key content
- Pretraining has recently greatly promoted the development of natural language processing (NLP)
- We show that M6 outperforms the baselines in multimodal downstream tasks, and the large M6 with 10 parameters can reach a better performance
- We propose a method called M6 that is able to process information of multiple modalities and perform both single-modal and cross-modal understanding and generation
- The model is scaled to large model with 10 billion parameters with sophisticated deployment, and the 10 -parameter M6-large is the largest pretrained model in Chinese
- Experimental results show that our proposed M6 outperforms the baseline in a number of downstream tasks concerning both single modality and multiple modalities We will continue the pretraining of extremely large models by increasing data to explore the limit of its performance
Upload PDF to Generate Summary
Must-Reading Tree
Example
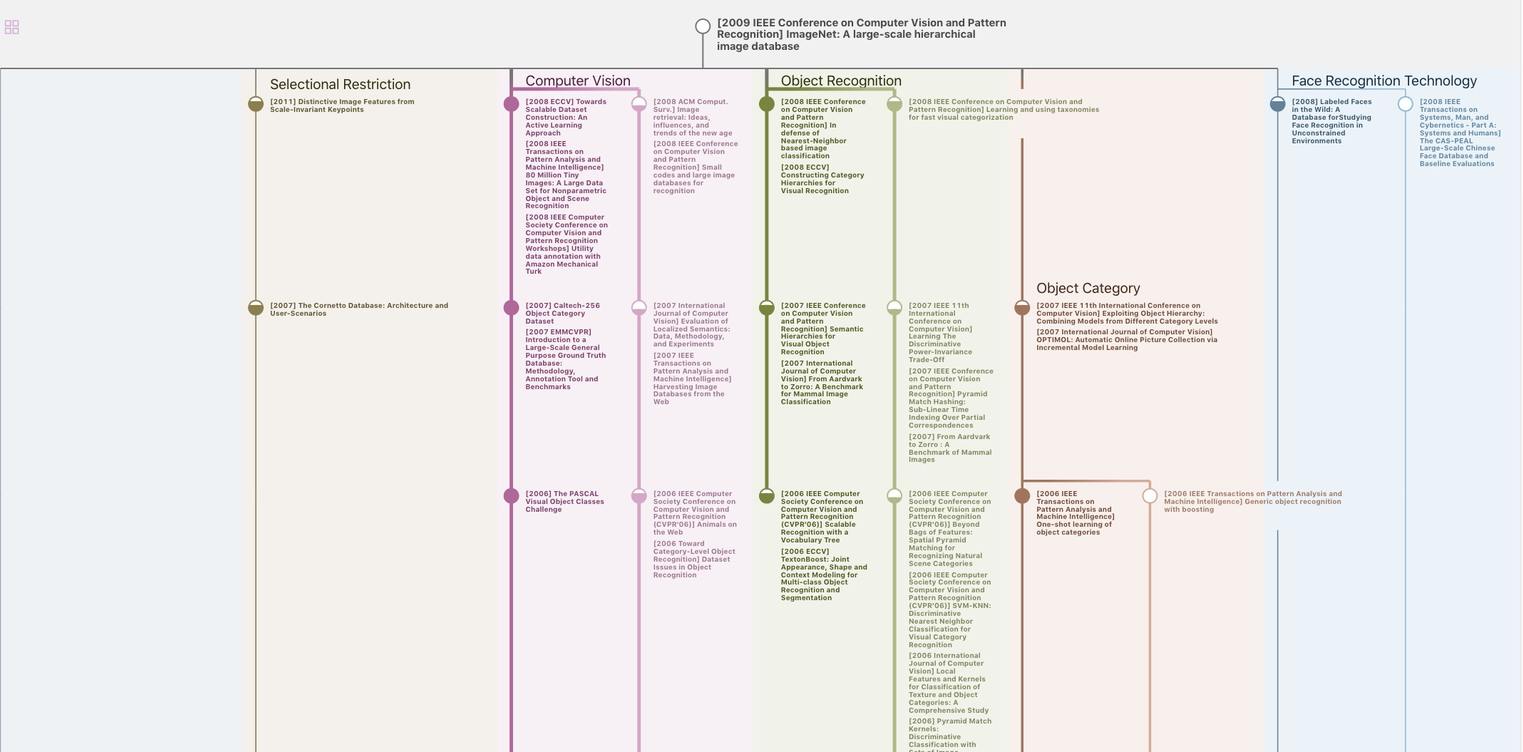
Generate MRT to find the research sequence of this paper
Related Papers
2017
被引用1085 | 浏览
2018
被引用7 | 浏览
2021
被引用10 | 浏览
Data Disclaimer
The page data are from open Internet sources, cooperative publishers and automatic analysis results through AI technology. We do not make any commitments and guarantees for the validity, accuracy, correctness, reliability, completeness and timeliness of the page data. If you have any questions, please contact us by email: report@aminer.cn
Chat Paper
GPU is busy, summary generation fails
Rerequest