A Multi-stage Multi-modal Classification Model for DeepFakes Combining Deep Learned and Computer Vision Oriented Features
INFORMATION SYSTEMS SECURITY, ICISS 2023(2023)
摘要
Recent advances in deep learning have empowered media synthesis and alteration to achieve levels of realism that were previously unheard of. Artificial intelligence is a potent tool that may be used to modify digital data, such as images, videos, and audio files, through the use of emerging deepfake technologies. Deepfake technology has the potential to significantly affect the reliability of multimedia data through the synthesis of fake media. Significant ramifications arise from this for individuals, organizations, and society at large. With the pace and accessibility of social media, convincing deepfakes can swiftly reach millions of people and adversely influence public opinion. To this end, we propose a multi-modal feature-based classification model that can distinguish between deepfake and real videos efficiently. We have used prefabricated image features as well as a variety of Convolutional Neural Network (CNN) model-generated features, including ResNet50, ResNet101, VGG16, and VGG19. The fake videos are taken up for further investigation to detect their source of origin. We propose a CNN-based classifier for deepfake detection and also explore the efficiency of multiple feature-based classifiers in this respect. This enables us to evaluate the comparative performance of both. The proposed model achieves an accuracy of 99.06% on deepfake classification and 98.75% on source identification when tested on a publicly available FaceForensics++ dataset.
更多查看译文
关键词
Artificial Intelligence,Convolutional Neural Network,Digital Forensics,Deepfakes,Faceforensics
AI 理解论文
溯源树
样例
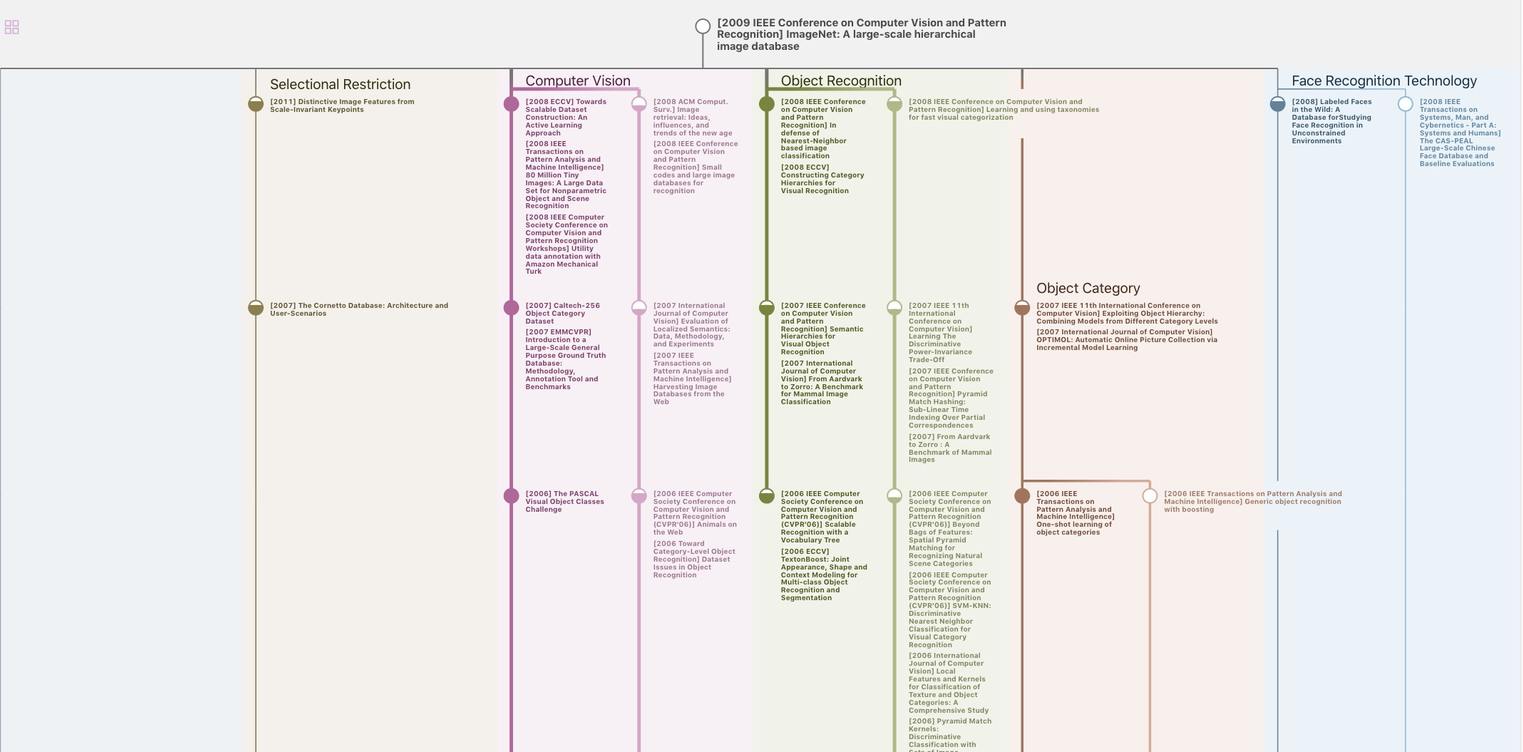
生成溯源树,研究论文发展脉络
Chat Paper
正在生成论文摘要