Combining Neighbor Models to Improve Predictions of Age of Onset of ATTRv Carriers
PROGRESS IN ARTIFICIAL INTELLIGENCE, EPIA 2023, PT II(2023)
摘要
Transthyretin (TTR)-related familial amyloid polyneuropathy (ATTRv) is a life-threatening autosomal dominant disease and the age of onset represents the moment when first symptoms are felt. Accurately predicting the age of onset for a given patient is relevant for risk assessment and treatment management. In this work, we evaluate the impact of combining prediction models obtained from neighboring time windows on prediction error. We propose Symmetric (Sym) and Asymmetric (Asym) models which represent two different averaging approaches. These are incorporated with a weighting mechanism as to create Symmetric (Sym), Symmetric-weighted (Sym-w), Asymmetric (Asym), and Asymmetric-weighted (Asym-w). These four ensemble models are then compared to the original approach which is focused on individual regression base learners namely: Baseline (BL), Decision Tree (DT), Elastic Net (EN), Lasso (LA), Linear Regression (LR), Random Forest (RF), Ridge (RI), Support Vector Regressor (SV) and XGBoost (XG). Our results show that by aggregating predictions from neighbor models the average mean absolute error obtained by each base learner decreases. Overall, the best results are achieved by regression-based ensemble tree models as base learners.
更多查看译文
关键词
Additive ensemble,Age of onset prediction,Regression ensemble
AI 理解论文
溯源树
样例
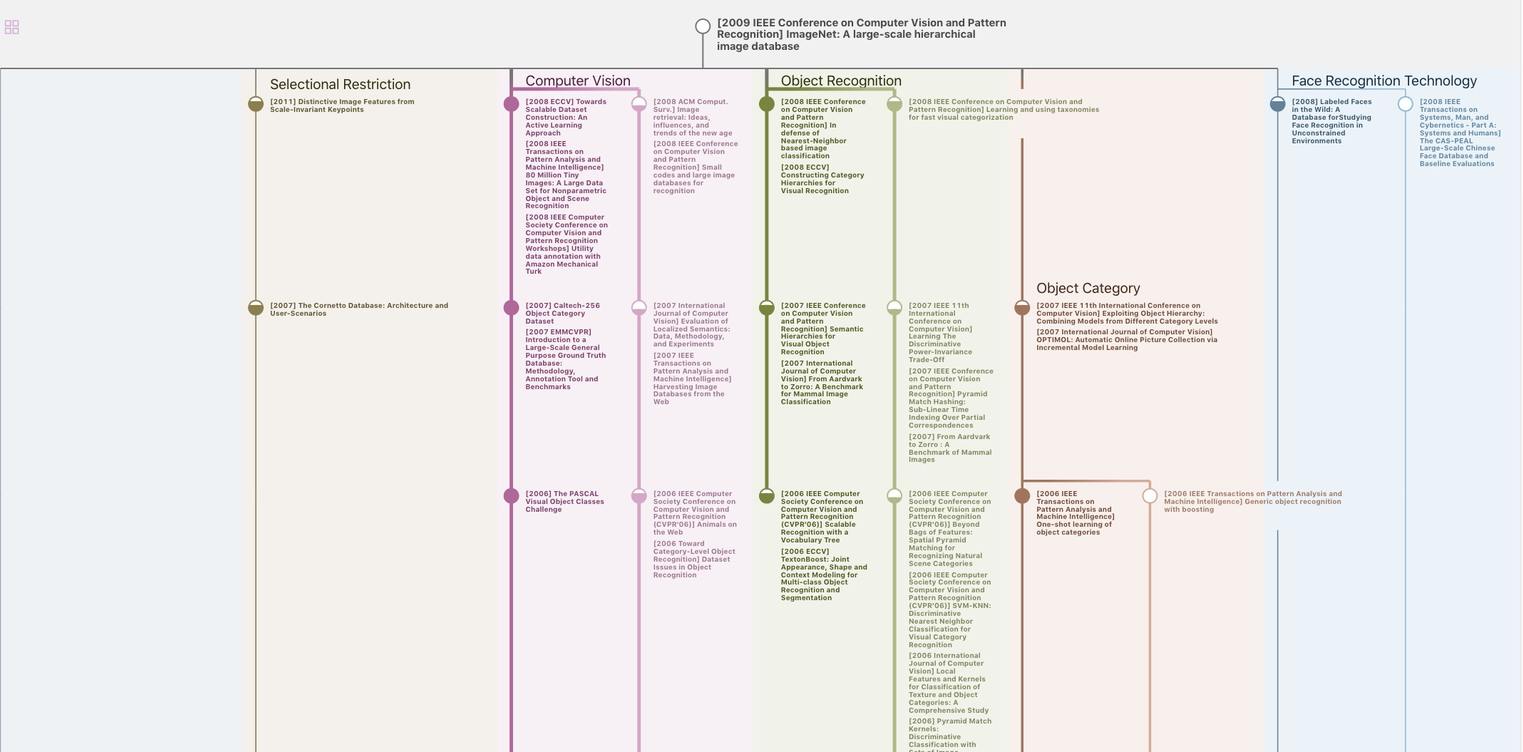
生成溯源树,研究论文发展脉络
Chat Paper
正在生成论文摘要