An interpretable composite CNN and GRU for fine-grained martial arts motion modeling using big data analytics and machine learning
Soft Computing(2024)
摘要
Martial arts involve highly complex motions with intricate poses and maneuvers. Current video analysis methods face challenges in robustly scaling across diverse data while accurately modeling the nuances of techniques and forms. This research develops a deep learning framework integrating convolutional neural networks (CNN) and Gated Recurrent Units (GRU) on Apache Spark to model martial arts poses and motions. The CNN extracts hierarchical visual features, highlighting athletes and movements in multimedia data. The GRU layers leverage temporal dynamics by processing pose sequences and actions via gating mechanisms. The GRU maintains an internal state, updating it based on current inputs and previous states. Its gates selectively retain context enabling the GRU to summarize variable-length time series into fixed pose encodings. The framework concatenates CNN visual features and GRU sequential states to provide interpretable pose representations. This granular encoding captures subtle posture details for fine-grained technique analysis. Comparative experiments validate the approach through accurate classification on UCF101 and precise pose estimation on the MADS dataset. The Spark integration enables scalable distributed training on these datasets. Specifically, the approach achieves 94% validation accuracy on martial arts action recognition. It also demonstrates robust performance with low MSE of 2.48 and MAE of 1.89 for pose estimation. The validated framework powers applications such as augmented coaching, optimized training regimes, progress tracking, and skill assessment to enhance martial arts mastery. Overall, the research pioneers an interpretable deep learning solution tailored for fine-grained martial arts training through scalable spatio-temporal modeling of complex motions.
更多查看译文
关键词
Martial art training,Convolutional neural networks,Gated recurrent units (GRU),Pose estimation,Action recognition,Deep learning
AI 理解论文
溯源树
样例
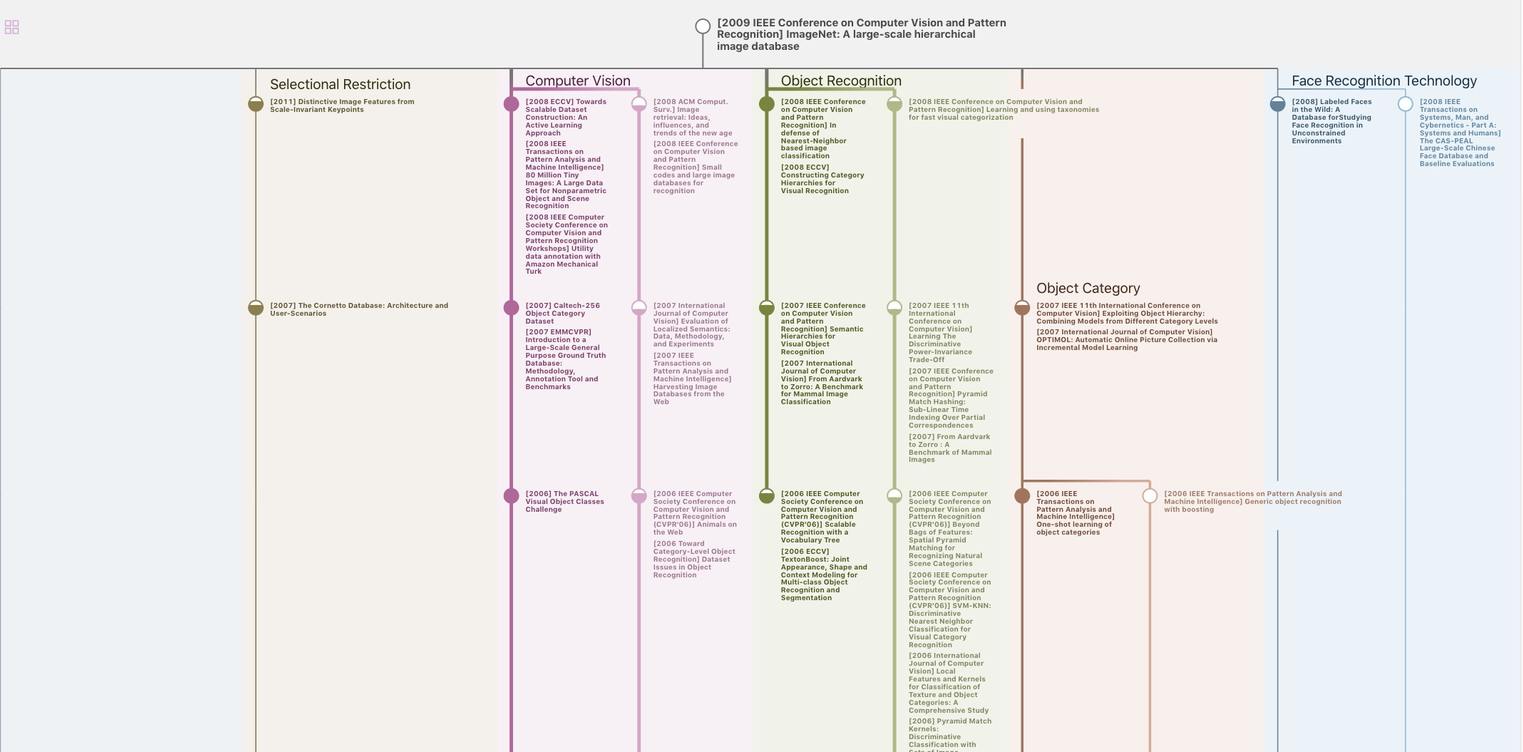
生成溯源树,研究论文发展脉络
Chat Paper
正在生成论文摘要