Prediction of medial knee contact force using multisource fusion recurrent neural network and transfer learning
Medical & biological engineering & computing(2024)
摘要
Estimation of knee contact force (KCF) during gait provides essential information to evaluate knee joint function. Machine learning has been employed to estimate KCF because of the advantages of low computational cost and real-time. However, the existing machine learning models do not adequately consider gait-related data’s temporal-dependent, multidimensional, and highly heterogeneous nature. This study is aimed at developing a multisource fusion recurrent neural network to predict the medial condyle KCF. First, a multisource fusion long short-term memory (MF-LSTM) model was established. Then, we developed a transfer learning strategy based on the MF-LSTM model for subject-specific medial KCF prediction. Four subjects with instrumented tibial prostheses were obtained from the literature. The results showed that the MF-LSTM model could predict medial KCF to a certain high level of accuracy (the mean of ρ = 0.970). The transfer learning model improved the prediction accuracy (the mean of ρ = 0.987). This study shows that the MF-LSTM model is a powerful and accurate computational tool for medial KCF prediction. Introducing transfer learning techniques could further improve the prediction performance for the target subject. This coupling strategy can help clinicians accurately estimate and track joint contact forces in real time. Graphical abstract Gait-related data are temporal-dependent, multidimensional, and highly heterogeneous. Therefore, we designed a multisource fusion recurrent neural network (MF-LSTM) structure and introduced a transfer learning strategy to predict knee contact force (KCF) during gait.
更多查看译文
关键词
Knee contact force,Long short-term memory network,Transfer learning,Multisource fusion
AI 理解论文
溯源树
样例
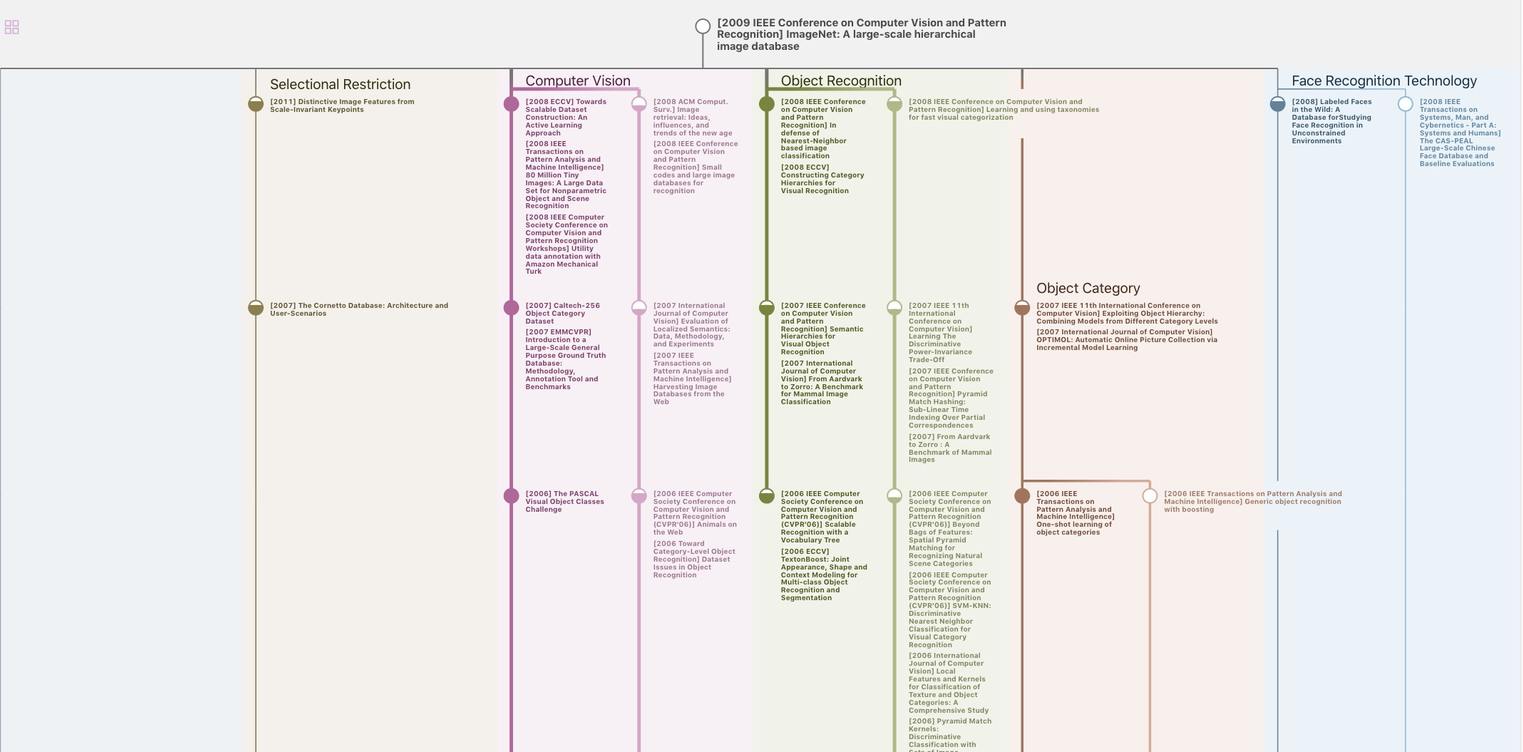
生成溯源树,研究论文发展脉络
Chat Paper
正在生成论文摘要