Learning to detect extreme objects for remote sensing images
International Journal of Machine Learning and Cybernetics(2024)
摘要
The scale and shape of objects in remote sensing images varies greatly, and thus the object detection of remote sensing image has always been a relatively challenging task. Focusing on the problem of multi-scale and extreme aspect ratio object detection, this paper proposes the extreme object detection net (EODNet). Firstly, we design a fractal-based feature fusion network (FFFN) by stacking different multilayer elementary convolutional blocks based on the self-similarity feature of fractals, so that the fused feature maps can fully retain the original features to facilitate the extraction of objects of different sizes. The parallel and serial asymmetric convolution block is fitted objects by forming receptive fields that approximate the shape of the object for feature maps used to detect large-sized objects. Following this, the width-height sensitive loss function is designed, which adapts to multi-scale objects and is sensitive to shape. Finally, the Extreme-soft- non maximum suppression (NMS) realizes the removal of extreme overlapping boxes and avoids the accidental deletion of dense object prediction boxes. The proposed method is tested on the NWPU VHR-10 and RSOD datasets, and the results are compared with mainstream object detection algorithms, verifying the effectiveness of the proposed method, as the mAP on the two datasets is 96.1% and 95.4%, respectively.
更多查看译文
关键词
Extreme aspect ratio,Multi-scale,Object detection,Remote sensing images,Self-similarity,Shape sensitive
AI 理解论文
溯源树
样例
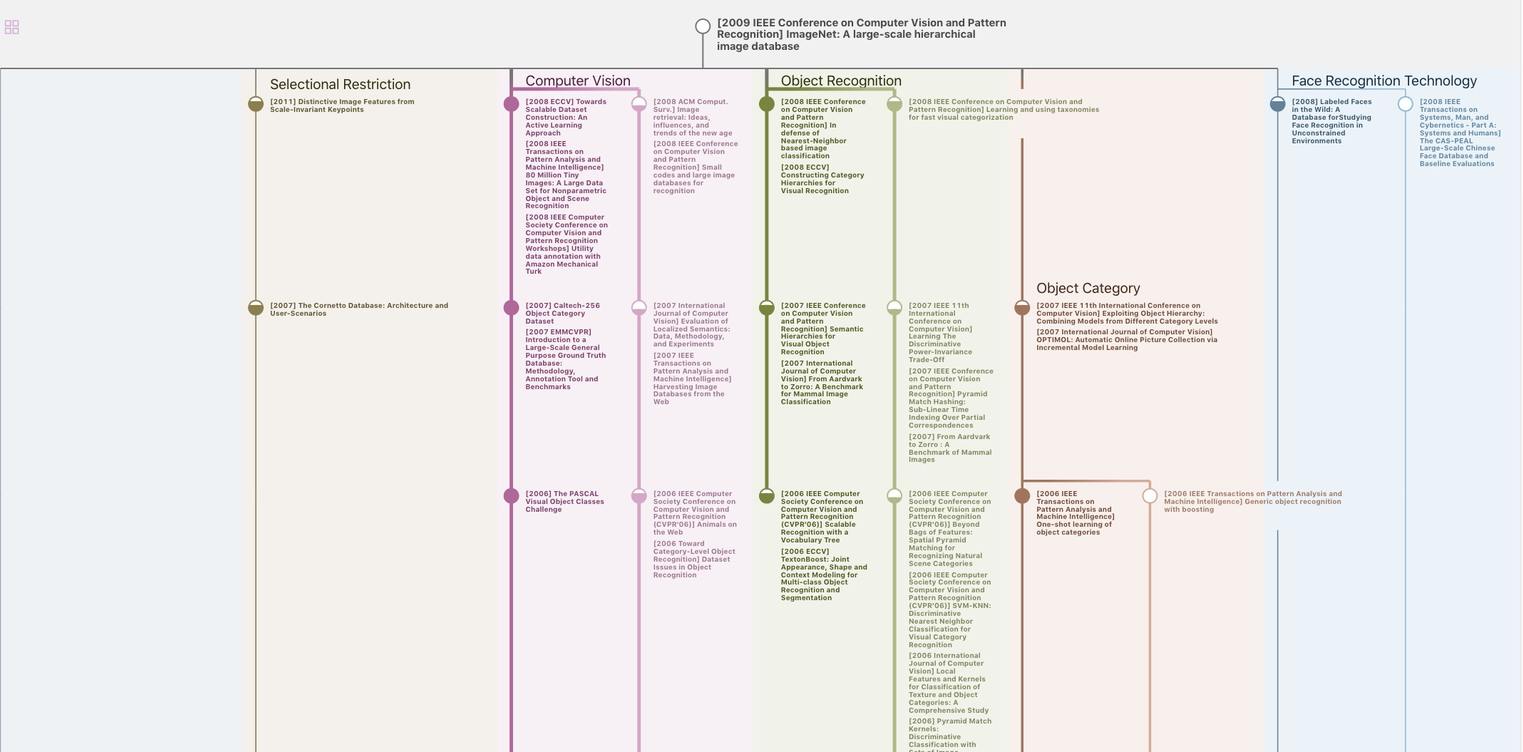
生成溯源树,研究论文发展脉络
Chat Paper
正在生成论文摘要