PROVIDENT: Development and Validation of a Machine Learning Model to Predict Neighborhood-level Overdose Risk in Rhode Island
EPIDEMIOLOGY(2024)
摘要
Background: Drug overdose persists as a leading cause of death in the United States, but resources to address it remain limited. As a result, health authorities must consider where to allocate scarce resources within their jurisdictions. Machine learning offers a strategy to identify areas with increased future overdose risk to proactively allocate overdose prevention resources. This modeling study is embedded in a randomized trial to measure the effect of proactive resource allocation on statewide overdose rates in Rhode Island (RI).We used statewide data from RI from 2016 to 2020 to develop an ensemble machine learning model predicting neighborhood-level fatal overdose risk. Our ensemble model integrated gradient boosting machine and super learner base models in a moving window framework to make predictions in 6-month intervals. Our performance target, developed a priori with the RI Department of Health, was to identify the 20% of RI neighborhoods containing at least 40% of statewide overdose deaths, including at least one neighborhood per municipality. The model was validated after trial launch.Our model selected priority neighborhoods capturing 40.2% of statewide overdose deaths during the test periods and 44.1% of statewide overdose deaths during validation periods. Our ensemble outperformed the base models during the test periods and performed comparably to the best-performing base model during the validation periods.We demonstrated the capacity for machine learning models to predict neighborhood-level fatal overdose risk to a degree of accuracy suitable for practitioners. Jurisdictions may consider predictive modeling as a tool to guide allocation of scarce resources.This modeling study is embedded within the Preventing Overdose using Information and Data from the Environment (PROVIDENT) randomized controlled trial (NCT05096429), which aims to test the effect of allocating overdose prevention resources in Rhode Island (RI) according to machine learning-based prediction of future overdose risk, in comparison to reactive responses guided by traditional surveillance reports.8 The trial's central hypothesis is that proactive resource allocation based on machine learning model predictions can more effectively reduce drug overdose-related morbidity and mortality in the context of a spatially dynamic epidemic, compared with standard resource allocation approaches using epidemiologic surveillance. The PROVIDENT trial is conducted in partnership with the RI Department of Health (RIDOH), which centralizes overdose prevention resources and infrastructure across RI. As part of an academic-state health department partnership,9 the trial randomized each of RI's 39 municipalities to an intervention condition, where model predictions are available to identify prioritized neighborhoods with highest risk of future fatal overdose, or to a control condition receiving overdose prevention resources as usual in accordance with the state's strategic plan and based on routine surveillance reporting without targeted prioritization. Details of the PROVIDENT trial design and protocol are available in a prior publication.8 This study presents the development and internal validation of the predictive model informing the intervention and describes the evaluation criteria used to assess its performance.The PROVIDENT trial seeks to determine whether machine learning models might enable public health practitioners to leverage available data to predict future community-level overdose risk and proactively allocate resources. Advances in small-area prediction using spatiotemporal machine learning methods indicate that accurate forecasting of future community overdose risk is possible.10 Such methods may bolster the impact of limited resources by prioritizing both communities with endemically high rates of overdose death and emerging overdose "hotspots." Machine learning methods leverage a broad array of surveillance data as a single, high-dimensional dataset without the need for theory-driven feature selection.11 With predictive performance as the goal, machine learning also can facilitate integration of complementary predictive approaches (e.g., spatiotemporal and tree based) through ensemble techniques.12To inform allocation of overdose prevention interventions and to maximize the impact of finite resources in RI, we developed a machine learning tool to forecast future neighborhood-level overdose burden. Our study builds on prior equity-focused work leveraging machine learning to target interventions in resource-limited settings in substance use and HIV.13-16 We uniquely focus on area-level overdose prevention interventions, partnering directly with public health practitioners. We note that the majority of machine learning work related to overdose has focused on individual-level prediction to inform clinical intervention,17-21 whereas our study focuses on community-level prediction to inform public health intervention, a novel area for the application of machine learning. Using a variety of public health, social, environmental, and economic data sources widely available to state and local health authorities in the US and across multiple domains for which prior literature has established associations with neighborhood-level overdose mortality,8 the model predicts future community-level overdose risk in neighborhoods across RI.Background: Drug overdose persists as a leading cause of death in the United States, but resources to address it remain limited. As a result, health authorities must consider where to allocate scarce resources within their jurisdictions. Machine learning offers a strategy to identify areas with increased future overdose risk to proactively allocate overdose prevention resources. This modeling study is embedded in a randomized trial to measure the effect of proactive resource allocation on statewide overdose rates in Rhode Island (RI).We used statewide data from RI from 2016 to 2020 to develop an ensemble machine learning model predicting neighborhood-level fatal overdose risk. Our ensemble model integrated gradient boosting machine and super learner base models in a moving window framework to make predictions in 6-month intervals. Our performance target, developed a priori with the RI Department of Health, was to identify the 20% of RI neighborhoods containing at least 40% of statewide overdose deaths, including at least one neighborhood per municipality. The model was validated after trial launch.Our model selected priority neighborhoods capturing 40.2% of statewide overdose deaths during the test periods and 44.1% of statewide overdose deaths during validation periods. Our ensemble outperformed the base models during the test periods and performed comparably to the best-performing base model during the validation periods.We demonstrated the capacity for machine learning models to predict neighborhood-level fatal overdose risk to a degree of accuracy suitable for practitioners. Jurisdictions may consider predictive modeling as a tool to guide allocation of scarce resources. This modeling study is embedded within the Preventing Overdose using Information and Data from the Environment (PROVIDENT) randomized controlled trial (NCT05096429), which aims to test the effect of allocating overdose prevention resources in Rhode Island (RI) according to machine learning-based prediction of future overdose risk, in comparison to reactive responses guided by traditional surveillance reports.8 The trial's central hypothesis is that proactive resource allocation based on machine learning model predictions can more effectively reduce drug overdose-related morbidity and mortality in the context of a spatially dynamic epidemic, compared with standard resource allocation approaches using epidemiologic surveillance. The PROVIDENT trial is conducted in partnership with the RI Department of Health (RIDOH), which centralizes overdose prevention resources and infrastructure across RI. As part of an academic-state health department partnership,9 the trial randomized each of RI's 39 municipalities to an intervention condition, where model predictions are available to identify prioritized neighborhoods with highest risk of future fatal overdose, or to a control condition receiving overdose prevention resources as usual in accordance with the state's strategic plan and based on routine surveillance reporting without targeted prioritization. Details of the PROVIDENT trial design and protocol are available in a prior publication.8 This study presents the development and internal validation of the predictive model informing the intervention and describes the evaluation criteria used to assess its performance.The PROVIDENT trial seeks to determine whether machine learning models might enable public health practitioners to leverage available data to predict future community-level overdose risk and proactively allocate resources. Advances in small-area prediction using spatiotemporal machine learning methods indicate that accurate forecasting of future community overdose risk is possible.10 Such methods may bolster the impact of limited resources by prioritizing both communities with endemically high rates of overdose death and emerging overdose "hotspots." Machine learning methods leverage a broad array of surveillance data as a single, high-dimensional dataset without the need for theory-driven feature selection.11 With predictive performance as the goal, machine learning also can facilitate integration of complementary predictive approaches (e.g., spatiotemporal and tree based) through ensemble techniques.12To inform allocation of overdose prevention interventions and to maximize the impact of finite resources in RI, we developed a machine learning tool to forecast future neighborhood-level overdose burden. Our study builds on prior equity-focused work leveraging machine learning to target interventions in resource-limited settings in substance use and HIV.13-16 We uniquely focus on area-level overdose prevention interventions, partnering directly with public health practitioners. We note that the majority of machine learning work related to overdose has focused on individual-level prediction to inform clinical intervention,17-21 whereas our study focuses on community-level prediction to inform public health intervention, a novel area for the application of machine learning. Using a variety of public health, social, environmental, and economic data sources widely available to state and local health authorities in the US and across multiple domains for which prior literature has established associations with neighborhood-level overdose mortality,8 the model predicts future community-level overdose risk in neighborhoods across RI.Background: Drug overdose persists as a leading cause of death in the United States, but resources to address it remain limited. As a result, health authorities must consider where to allocate scarce resources within their jurisdictions. Machine learning offers a strategy to identify areas with increased future overdose risk to proactively allocate overdose prevention resources. This modeling study is embedded in a randomized trial to measure the effect of proactive resource allocation on statewide overdose rates in Rhode Island (RI).We used statewide data from RI from 2016 to 2020 to develop an ensemble machine learning model predicting neighborhood-level fatal overdose risk. Our ensemble model integrated gradient boosting machine and super learner base models in a moving window framework to make predictions in 6-month intervals. Our performance target, developed a priori with the RI Department of Health, was to identify the 20% of RI neighborhoods containing at least 40% of statewide overdose deaths, including at least one neighborhood per municipality. The model was validated after trial launch.Our model selected priority neighborhoods capturing 40.2% of statewide overdose deaths during the test periods and 44.1% of statewide overdose deaths during validation periods. Our ensemble outperformed the base models during the test periods and performed comparably to the best-performing base model during the validation periods.We demonstrated the capacity for machine learning models to predict neighborhood-level fatal overdose risk to a degree of accuracy suitable for practitioners. Jurisdictions may consider predictive modeling as a tool to guide allocation of scarce resources.This modeling study is embedded within the Preventing Overdose using Information and Data from the Environment (PROVIDENT) randomized controlled trial (NCT05096429), which aims to test the effect of allocating overdose prevention resources in Rhode Island (RI) according to machine learning-based prediction of future overdose risk, in comparison to reactive responses guided by traditional surveillance reports.8 The trial's central hypothesis is that proactive resource allocation based on machine learning model predictions can more effectively reduce drug overdose-related morbidity and mortality in the context of a spatially dynamic epidemic, compared with standard resource allocation approaches using epidemiologic surveillance. The PROVIDENT trial is conducted in partnership with the RI Department of Health (RIDOH), which centralizes overdose prevention resources and infrastructure across RI. As part of an academic-state health department partnership,9 the trial randomized each of RI's 39 municipalities to an intervention condition, where model predictions are available to identify prioritized neighborhoods with highest risk of future fatal overdose, or to a control condition receiving overdose prevention resources as usual in accordance with the state's strategic plan and based on routine surveillance reporting without targeted prioritization. Details of the PROVIDENT trial design and protocol are available in a prior publication. 8 This study presents the development and internal validation of the predictive model informing the intervention and describes the evaluation criteria used to assess its performance.The PROVIDENT trial seeks to determine whether machine learning models might enable public health practitioners to leverage available data to predict future community-level overdose risk and proactively allocate resources. Advances in small-area prediction using spatiotemporal machine learning methods indicate that accurate forecasting of future community overdose risk is possible.10 Such methods may bolster the impact of limited resources by prioritizing both communities with endemically high rates of overdose death and emerging overdose "hotspots." Machine learning methods leverage a broad array of surveillance data as a single, high-dimensional dataset without the need for theory-driven feature selection.11 With predictive performance as the goal, machine learning also can facilitate integration of complementary predictive approaches (e.g., spatiotemporal and tree based) through ensemble techniques.12To inform allocation of overdose prevention interventions and to maximize the impact of finite resources in RI, we developed a machine learning tool to forecast future neighborhood-level overdose burden. Our study builds on prior equity-focused work leveraging machine learning to target interventions in resource-limited settings in substance use and HIV.13-16 We uniquely focus on area-level overdose prevention interventions, partnering directly with public health practitioners. We note that the majority of machine learning work related to overdose has focused on individual-level prediction to inform clinical intervention,17-21 whereas our study focuses on community-level prediction to inform public health intervention, a novel area for the application of machine learning. Using a variety of public health, social, environmental, and economic data sources widely available to state and local health authorities in the US and across multiple domains for which prior literature has established associations with neighborhood-level overdose mortality,8 the model predicts future community-level overdose risk in neighborhoods across RI.Background: Drug overdose persists as a leading cause of death in the United States, but resources to address it remain limited. As a result, health authorities must consider where to allocate scarce resources within their jurisdictions. Machine learning offers a strategy to identify areas with increased future overdose risk to proactively allocate overdose prevention resources. This modeling study is embedded in a randomized trial to measure the effect of proactive resource allocation on statewide overdose rates in Rhode Island (RI).We used statewide data from RI from 2016 to 2020 to develop an ensemble machine learning model predicting neighborhood-level fatal overdose risk. Our ensemble model integrated gradient boosting machine and super learner base models in a moving window framework to make predictions in 6-month intervals. Our performance target, developed a priori with the RI Department of Health, was to identify the 20% of RI neighborhoods containing at least 40% of statewide overdose deaths, including at least one neighborhood per municipality. The model was validated after trial launch.Our model selected priority neighborhoods capturing 40.2% of statewide overdose deaths during the test periods and 44.1% of statewide overdose deaths during validation periods. Our ensemble outperformed the base models during the test periods and performed comparably to the best-performing base model during the validation periods.We demonstrated the capacity for machine learning models to predict neighborhood-level fatal overdose risk to a degree of accuracy suitable for practitioners. Jurisdictions may consider predictive modeling as a tool to guide allocation of scarce resources.This modeling study is embedded within the Preventing Overdose using Information and Data from the Environment (PROVIDENT) randomized controlled trial (NCT05096429), which aims to test the effect of allocating overdose prevention resources in Rhode Island (RI) according to machine learning-based prediction of future overdose risk, in comparison to reactive responses guided by traditional surveillance reports.8 The trial's central hypothesis is that proactive resource allocation based on machine learning model predictions can more effectively reduce drug overdose-related morbidity and mortality in the context of a spatially dynamic epidemic, compared with standard resource allocation approaches using epidemiologic surveillance. The PROVIDENT trial is conducted in partnership with the RI Department of Health (RIDOH), which centralizes overdose prevention resources and infrastructure across RI. As part of an academic-state health department partnership,9 the trial randomized each of RI's 39 municipalities to an intervention condition, where model predictions are available to identify prioritized neighborhoods with highest risk of future fatal overdose, or to a control condition receiving overdose prevention resources as usual in accordance with the state's strategic plan and based on routine surveillance reporting without targeted prioritization. Details of the PROVIDENT trial design and protocol are available in a prior publication.8 This study presents the development and internal validation of the predictive model informing the intervention and describes the evaluation criteria used to assess its performance.The PROVIDENT trial seeks to determine whether machine learning models might enable public health practitioners to leverage available data to predict future community-level overdose risk and proactively allocate resources. Advances in small-area prediction using spatiotemporal machine learning methods indicate that accurate forecasting of future community overdose risk is possible.10 Such methods may bolster the impact of limited resources by prioritizing both communities with endemically high rates of overdose death and emerging overdose "hotspots." Machine learning methods leverage a broad array of surveillance data as a single, high-dimensional dataset without the need for theory-driven feature selection.11 With predictive performance as the goal, machine learning also can facilitate integration of complementary predictive approaches (e.g., spatiotemporal and tree based) through ensemble techniques.12To inform allocation of overdose prevention interventions and to maximize the impact of finite resources in RI, we developed a machine learning tool to forecast future neighborhood-level overdose burden. Our study builds on prior equity-focused work leveraging machine learning to target interventions in resource-limited settings in substance use and HIV.13-16 We uniquely focus on area-level overdose prevention interventions, partnering directly with public health practitioners. We note that the majority of machine learning work related to overdose has focused on individual-level prediction to inform clinical intervention,17-21 whereas our study focuses on community-level prediction to inform public health intervention, a novel area for the application of machine learning. Using a variety of public health, social, environmental, and economic data sources widely available to state and local health authorities in the US and across multiple domains for which prior literature has established associations with neighborhood-level overdose mortality,8 the model predicts future community-level overdose risk in neighborhoods across RI.Background: Drug overdose persists as a leading cause of death in the United States, but resources to address it remain limited. As a result, health authorities must consider where to allocate scarce resources within their jurisdictions. Machine learning offers a strategy to identify areas with increased future overdose risk to proactively allocate overdose prevention resources. This modeling study is embedded in a randomized trial to measure the effect of proactive resource allocation on statewide overdose rates in Rhode Island (RI).We used statewide data from RI from 2016 to 2020 to develop an ensemble machine learning model predicting neighborhood-level fatal overdose risk. Our ensemble model integrated gradient boosting machine and super learner base models in a moving window framework to make predictions in 6-month intervals. Our performance target, developed a priori with the RI Department of Health, was to identify the 20% of RI neighborhoods containing at least 40% of statewide overdose deaths, including at least one neighborhood per municipality. The model was validated after trial launch.Our model selected priority neighborhoods capturing 40.2% of statewide overdose deaths during the test periods and 44.1% of statewide overdose deaths during validation periods. Our ensemble outperformed the base models during the test periods and performed comparably to the best-performing base model during the validation periods.We demonstrated the capacity for machine learning models to predict neighborhood-level fatal overdose risk to a degree of accuracy suitable for practitioners. Jurisdictions may consider predictive modeling as a tool to guide allocation of scarce resources.This modeling study is embedded within the Preventing Overdose using Information and Data from the Environment (PROVIDENT) randomized controlled trial (NCT05096429), which aims to test the effect of allocating overdose prevention resources in Rhode Island (RI) according to machine learning-based prediction of future overdose risk, in comparison to reactive responses guided by traditional surveillance reports.8 The trial's central hypothesis is that proactive resource allocation based on machine learning model predictions can more effectively reduce drug overdose-related morbidity and mortality in the context of a spatially dynamic epidemic, compared with standard resource allocation approaches using epidemiologic surveillance. The PROVIDENT trial is conducted in partnership with the RI Department of Health (RIDOH), which centralizes overdose prevention resources and infrastructure across RI. As part of an academic-state health department partnership,9 the trial randomized each of RI's 39 municipalities to an intervention condition, where model predictions are available to identify prioritized neighborhoods with highest risk of future fatal overdose, or to a control condition receiving overdose prevention resources as usual in accordance with the state's strategic plan and based on routine surveillance reporting without targeted prioritization. Details of the PROVIDENT trial design and protocol are available in a prior publication.8 This study presents the development and internal validation of the predictive model informing the intervention and describes the evaluation criteria used to assess its performance.The PROVIDENT trial seeks to determine whether machine learning models might enable public health practitioners to leverage available data to predict future community-level overdose risk and proactively allocate resources. Advances in small-area prediction using spatiotemporal machine learning methods indicate that accurate forecasting of future community overdose risk is possible.10 Such methods may bolster the impact of limited resources by prioritizing both communities with endemically high rates of overdose death and emerging overdose "hotspots." Machine learning methods leverage a broad array of surveillance data as a single, high-dimensional dataset without the need for theory-driven feature selection.11 With predictive performance as the goal, machine learning also can facilitate integration of complementary predictive approaches (e.g., spatiotemporal and tree based) through ensemble techniques.12To inform allocation of overdose prevention interventions and to maximize the impact of finite resources in RI, we developed a machine learning tool to forecast future neighborhood-level overdose burden. Our study builds on prior equity-focused work leveraging machine learning to target interventions in resource-limited settings in substance use and HIV.13-16 We uniquely focus on area-level overdose prevention interventions, partnering directly with public health practitioners. We note that the majority of machine learning work related to overdose has focused on individual-level prediction to inform clinical intervention,17-21 whereas our study focuses on community-level prediction to inform public health intervention, a novel area for the application of machine learning. Using a variety of public health, social, environmental, and economic data sources widely available to state and local health authorities in the US and across multiple domains for which prior literature has established associations with neighborhood-level overdose mortality,8 the model predicts future community-level overdose risk in neighborhoods across RI.Background: Drug overdose persists as a leading cause of death in the United States, but resources to address it remain limited. As a result, health authorities must consider where to allocate scarce resources within their jurisdictions. Machine learning offers a strategy to identify areas with increased future overdose risk to proactively allocate overdose prevention resources. This modeling study is embedded in a randomized trial to measure the effect of proactive resource allocation on statewide overdose rates in Rhode Island (RI).We used statewide data from RI from 2016 to 2020 to develop an ensemble machine learning model predicting neighborhood-level fatal overdose risk. Our ensemble model integrated gradient boosting machine and super learner base models in a moving window framework to make predictions in 6-month intervals. Our performance target, developed a priori with the RI Department of Health, was to identify the 20% of RI neighborhoods containing at least 40% of statewide overdose deaths, including at least one neighborhood per municipality. The model was validated after trial launch.Our model selected priority neighborhoods capturing 40.2% of statewide overdose deaths during the test periods and 44.1% of statewide overdose deaths during validation periods. Our ensemble outperformed the base models during the test periods and performed comparably to the best-performing base model during the validation periods.We demonstrated the capacity for machine learning models to predict neighborhood-level fatal overdose risk to a degree of accuracy suitable for practitioners. Jurisdictions may consider predictive modeling as a tool to guide allocation of scarce resources.This modeling study is embedded within the Preventing Overdose using Information and Data from the Environment (PROVIDENT) randomized controlled trial (NCT05096429), which aims to test the effect of allocating overdose prevention resources in Rhode Island (RI) according to machine learning-based prediction of future overdose risk, in comparison to reactive responses guided by traditional surveillance reports.8 The trial's central hypothesis is that proactive resource allocation based on machine learning model predictions can more effectively reduce drug overdose-related morbidity and mortality in the context of a spatially dynamic epidemic, compared with standard resource allocation approaches using epidemiologic surveillance. The PROVIDENT trial is conducted in partnership with the RI Department of Health (RIDOH), which centralizes overdose prevention resources and infrastructure across RI. As part of an academic-state health department partnership,9 the trial randomized each of RI's 39 municipalities to an intervention condition, where model predictions are available to identify prioritized neighborhoods with highest risk of future fatal overdose, or to a control condition receiving overdose prevention resources as usual in accordance with the state's strategic plan and based on routine surveillance reporting without targeted prioritization. Details of the PROVIDENT trial design and protocol are available in a prior publication.8 This study presents the development and internal validation of the predictive model informing the intervention and describes the evaluation criteria used to assess its performance.The PROVIDENT trial seeks to determine whether machine learning models might enable public health practitioners to leverage available data to predict future community-level overdose risk and proactively allocate resources. Advances in small-area prediction using spatiotemporal machine learning methods indicate that accurate forecasting of future community overdose risk is possible.10 Such methods may bolster the impact of limited resources by prioritizing both communities with endemically high rates of overdose death and emerging overdose "hotspots." Machine learning methods leverage a broad array of surveillance data as a single, high-dimensional dataset without the need for theory-driven feature selection.11 With predictive performance as the goal, machine learning also can facilitate integration of complementary predictive approaches (e.g., spatiotemporal and tree based) through ensemble techniques. 12To inform allocation of overdose prevention interventions and to maximize the impact of finite resources in RI, we developed a machine learning tool to forecast future neighborhood-level overdose burden. Our study builds on prior equity-focused work leveraging machine learning to target interventions in resource-limited settings in substance use and HIV.13-16 We uniquely focus on area-level overdose prevention interventions, partnering directly with public health practitioners. We note that the majority of machine learning work related to overdose has focused on individual-level prediction to inform clinical intervention,17-21 whereas our study focuses on community-level prediction to inform public health intervention, a novel area for the application of machine learning. Using a variety of public health, social, environmental, and economic data sources widely available to state and local health authorities in the US and across multiple domains for which prior literature has established associations with neighborhood-level overdose mortality,8 the model predicts future community-level overdose risk in neighborhoods across RI.Background: Drug overdose persists as a leading cause of death in the United States, but resources to address it remain limited. As a result, health authorities must consider where to allocate scarce resources within their jurisdictions. Machine learning offers a strategy to identify areas with increased future overdose risk to proactively allocate overdose prevention resources. This modeling study is embedded in a randomized trial to measure the effect of proactive resource allocation on statewide overdose rates in Rhode Island (RI).We used statewide data from RI from 2016 to 2020 to develop an ensemble machine learning model predicting neighborhood-level fatal overdose risk. Our ensemble model integrated gradient boosting machine and super learner base models in a moving window framework to make predictions in 6-month intervals. Our performance target, developed a priori with the RI Department of Health, was to identify the 20% of RI neighborhoods containing at least 40% of statewide overdose deaths, including at least one neighborhood per municipality. The model was validated after trial launch.Our model selected priority neighborhoods capturing 40.2% of statewide overdose deaths during the test periods and 44.1% of statewide overdose deaths during validation periods. Our ensemble outperformed the base models during the test periods and performed comparably to the best-performing base model during the validation periods.We demonstrated the capacity for machine learning models to predict neighborhood-level fatal overdose risk to a degree of accuracy suitable for practitioners. Jurisdictions may consider predictive modeling as a tool to guide allocation of scarce resources.This modeling study is embedded within the Preventing Overdose using Information and Data from the Environment (PROVIDENT) randomized controlled trial (NCT05096429), which aims to test the effect of allocating overdose prevention resources in Rhode Island (RI) according to machine learning-based prediction of future overdose risk, in comparison to reactive responses guided by traditional surveillance reports.8 The trial's central hypothesis is that proactive resource allocation based on machine learning model predictions can more effectively reduce drug overdose-related morbidity and mortality in the context of a spatially dynamic epidemic, compared with standard resource allocation approaches using epidemiologic surveillance. The PROVIDENT trial is conducted in partnership with the RI Department of Health (RIDOH), which centralizes overdose prevention resources and infrastructure across RI. As part of an academic-state health department partnership,9 the trial randomized each of RI's 39 municipalities to an intervention condition, where model predictions are available to identify prioritized neighborhoods with highest risk of future fatal overdose, or to a control condition receiving overdose prevention resources as usual in accordance with the state's strategic plan and based on routine surveillance reporting without targeted prioritization. Details of the PROVIDENT trial design and protocol are available in a prior publication.8 This study presents the development and internal validation of the predictive model informing the intervention and describes the evaluation criteria used to assess its performance.The PROVIDENT trial seeks to determine whether machine learning models might enable public health practitioners to leverage available data to predict future community-level overdose risk and proactively allocate resources. Advances in small-area prediction using spatiotemporal machine learning methods indicate that accurate forecasting of future community overdose risk is possible.10 Such methods may bolster the impact of limited resources by prioritizing both communities with endemically high rates of overdose death and emerging overdose "hotspots." Machine learning methods leverage a broad array of surveillance data as a single, high-dimensional dataset without the need for theory-driven feature selection.11 With predictive performance as the goal, machine learning also can facilitate integration of complementary predictive approaches (e.g., spatiotemporal and tree based) through ensemble techniques.12To inform allocation of overdose prevention interventions and to maximize the impact of finite resources in RI, we developed a machine learning tool to forecast future neighborhood-level overdose burden. Our study builds on prior equity-focused work leveraging machine learning to target interventions in resource-limited settings in substance use and HIV.13-16 We uniquely focus on area-level overdose prevention interventions, partnering directly with public health practitioners. We note that the majority of machine learning work related to overdose has focused on individual-level prediction to inform clinical intervention,17-21 whereas our study focuses on community-level prediction to inform public health intervention, a novel area for the application of machine learning. Using a variety of public health, social, environmental, and economic data sources widely available to state and local health authorities in the US and across multiple domains for which prior literature has established associations with neighborhood-level overdose mortality,8 the model predicts future community-level overdose risk in neighborhoods across RI.
更多查看译文
关键词
machine learning,overdose,opioid,Super Learner,gradient boosting machine,Rhode Island
AI 理解论文
溯源树
样例
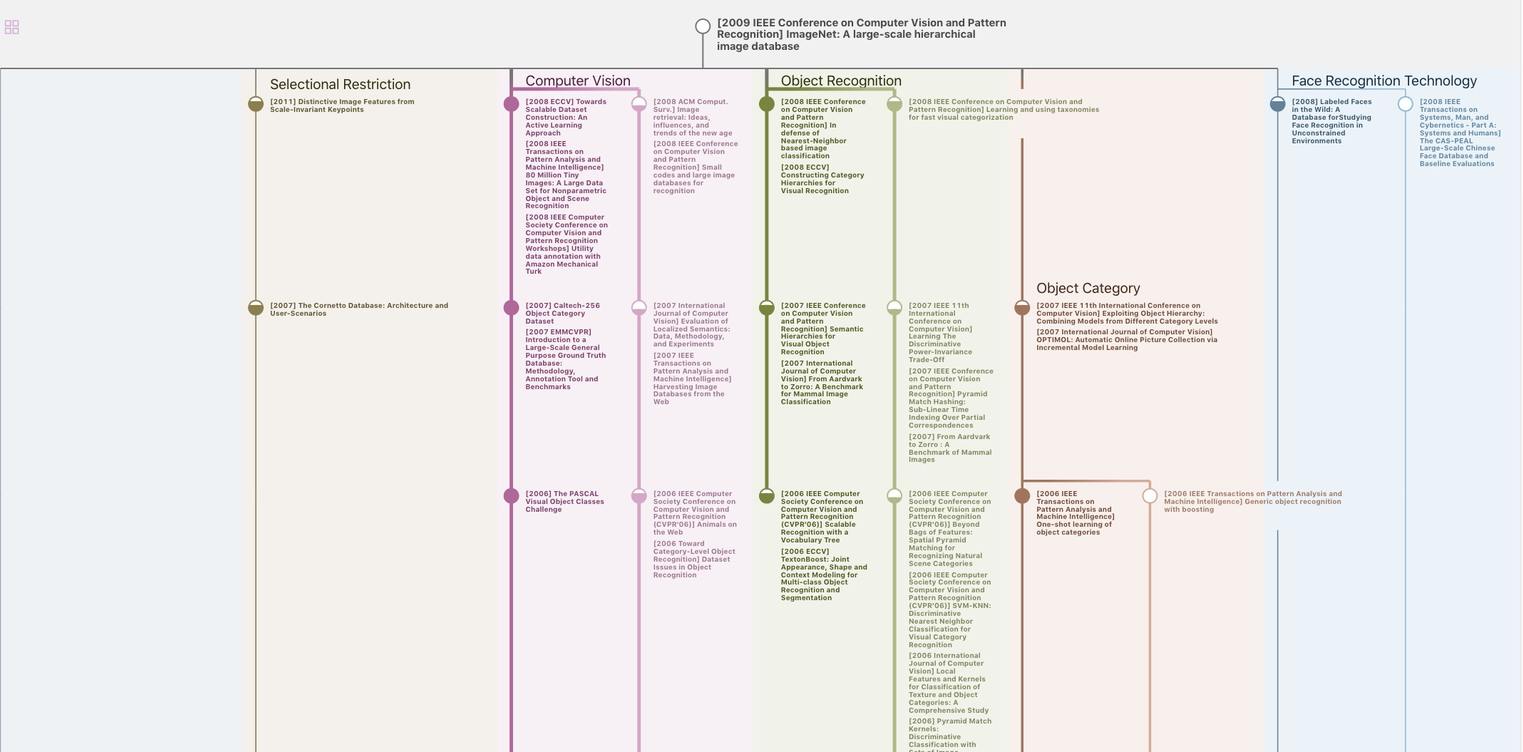
生成溯源树,研究论文发展脉络
Chat Paper
正在生成论文摘要