All-optical Encryption/decryption of DmPSK Signals with Key Steganography for Photonic Layer Security.
OPTICS EXPRESS(2023)
Northeastern Univ | Chongqing Univ Posts & Telecommun
Abstract
In the 5th Generation Fixed networks (F5G) era, full-fiber-connected optical networks support emerging bandwidth-hungry services. However, optical networks are vulnerable to attack by tapping or other methods, which has been paid more and more attention in modern optical infrastructure. Therefore, optical Exclusive OR (XOR) encryption/decryption for advanced modulation formats used for F5G appears as one of the promising technologies to guarantee security. Some current solutions either leave potential security hazards because of the degenerate four-wave mixing and keys without special treatment or are cost-consuming due to employing multiple wavelengths and coherent receivers. In this paper, a novel all-optical encryption/decryption system for Differential m -Phase Shift Keying (D m PSK) signals occupying only a single wavelength with key steganography is proposed and simulated. The proposed system mainly consists of a generalized XOR (GXOR) part and a steganography part. We implement the GXOR part by the cascaded IQ Mach-Zehnder Modulators and the steganography part by the Equvilent-Phase-Shifted Super-Structured Fiber Bragg Grating (EPS-SSFBG). The numerical simulation results demonstrate that the GXOR implementation can achieve reconfigurable encryption/decryption of DQPSK ( m = 4) or D8PSK ( m = 8) signals merely with a single wavelength and a differential direct-detection receiver. And the EPS-SSFBG can enhance key security in a cost-efficient method as well. The system can work at the recorded bit rate of 260Gbps, which can be applied to enhance the photonic layer security in F5G and beyond.
MoreTranslated text
Key words
Passive Optical Network,Photonic Signal Processing,Digital Signal Processing,Optical Performance Monitoring
求助PDF
上传PDF
View via Publisher
AI Read Science
AI Summary
AI Summary is the key point extracted automatically understanding the full text of the paper, including the background, methods, results, conclusions, icons and other key content, so that you can get the outline of the paper at a glance.
Example
Background
Key content
Introduction
Methods
Results
Related work
Fund
Key content
- Pretraining has recently greatly promoted the development of natural language processing (NLP)
- We show that M6 outperforms the baselines in multimodal downstream tasks, and the large M6 with 10 parameters can reach a better performance
- We propose a method called M6 that is able to process information of multiple modalities and perform both single-modal and cross-modal understanding and generation
- The model is scaled to large model with 10 billion parameters with sophisticated deployment, and the 10 -parameter M6-large is the largest pretrained model in Chinese
- Experimental results show that our proposed M6 outperforms the baseline in a number of downstream tasks concerning both single modality and multiple modalities We will continue the pretraining of extremely large models by increasing data to explore the limit of its performance
Upload PDF to Generate Summary
Must-Reading Tree
Example
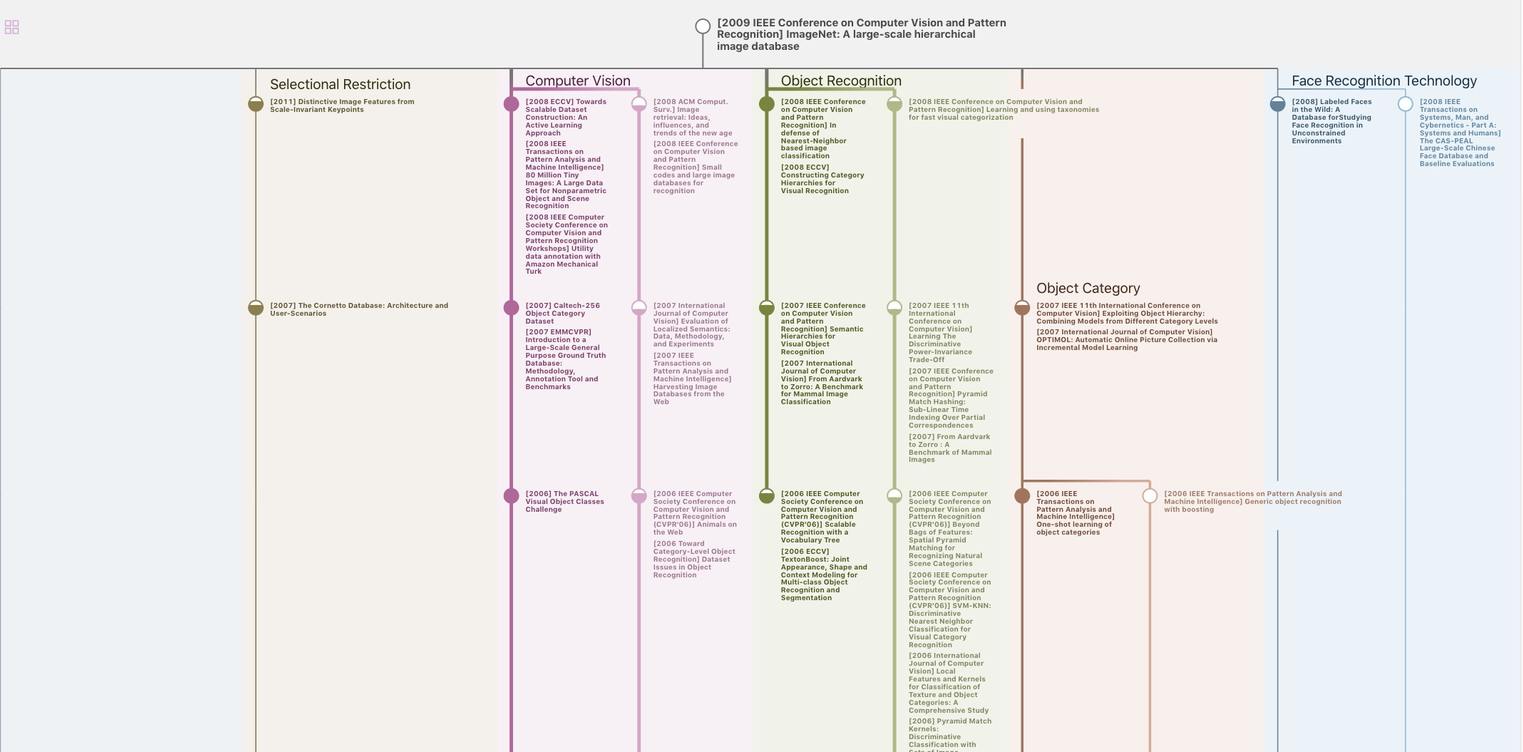
Generate MRT to find the research sequence of this paper
Related Papers
Demonstration of 10 Gbps, All-Optical Encryption and Decryption System Utilizing Soa Xor Logic Gates
2008
被引用39 | 浏览
2005
被引用55 | 浏览
2012
被引用41 | 浏览
2001
被引用70 | 浏览
2011
被引用11 | 浏览
2018
被引用18 | 浏览
2019
被引用12 | 浏览
2020
被引用9 | 浏览
2021
被引用9 | 浏览
Data Disclaimer
The page data are from open Internet sources, cooperative publishers and automatic analysis results through AI technology. We do not make any commitments and guarantees for the validity, accuracy, correctness, reliability, completeness and timeliness of the page data. If you have any questions, please contact us by email: report@aminer.cn
Chat Paper