Explainable AI models for predicting drop coalescence in microfluidics device
CHEMICAL ENGINEERING JOURNAL(2024)
摘要
In the field of chemical engineering, understanding the dynamics and probability of drop coalescence is not just an academic pursuit, but a critical requirement for advancing process design by applying energy only where it is needed to build necessary interfacial structures, increasing efficiency towards Net Zero manufacture. This research applies machine learning predictive models to unravel the sophisticated relationships embedded in the experimental data on drop coalescence in a microfluidics device. Through the deployment of SHapley Additive exPlanations values, critical features relevant to coalescence processes are consistently identified. Comprehensive feature ablation tests further delineate the robustness and susceptibility of each model. Furthermore, the incorporation of Local Interpretable Model -agnostic Explanations for local interpretability offers an elucidative perspective, clarifying the intricate decision -making mechanisms inherent to each model's predictions. As a result, this research provides the relative importance of the features for the outcome of drop interactions. It also underscores the pivotal role of model interpretability in reinforcing confidence in machine learning predictions of complex physical phenomena that are central to chemical engineering applications.
更多查看译文
关键词
Explainable AI,Drop coalescence,Machine learning,LIME,SHAP value
AI 理解论文
溯源树
样例
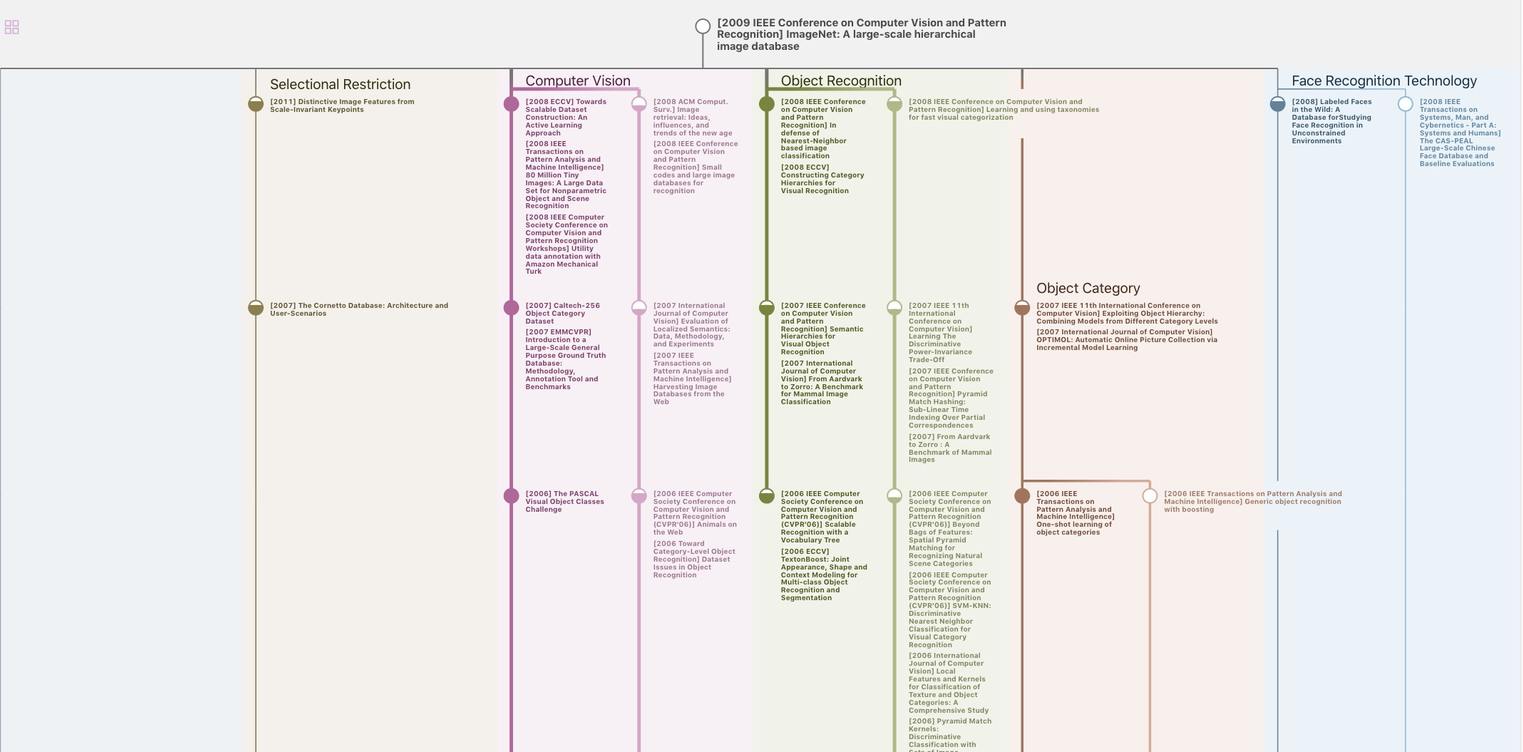
生成溯源树,研究论文发展脉络
Chat Paper
正在生成论文摘要