Energy-efficient Decentralized Learning via Graph Sparsification
CoRR(2024)
摘要
This work aims at improving the energy efficiency of decentralized learning
by optimizing the mixing matrix, which controls the communication demands
during the learning process. Through rigorous analysis based on a
state-of-the-art decentralized learning algorithm, the problem is formulated as
a bi-level optimization, with the lower level solved by graph sparsification. A
solution with guaranteed performance is proposed for the special case of
fully-connected base topology and a greedy heuristic is proposed for the
general case. Simulations based on real topology and dataset show that the
proposed solution can lower the energy consumption at the busiest node by
54
更多查看译文
关键词
Sparse Graph,Decentralized Learning,Energy Consumption,Mixing Matrix,Bilevel Optimization,Benchmark,Convergence Rate,Wireless Networks,Parameter Vector,Stochastic Gradient,Hyperparameter Tuning,Optimization Framework,Cost Model,Total Energy Consumption,Laplacian Matrix,Semidefinite Programming,Link Weights
AI 理解论文
溯源树
样例
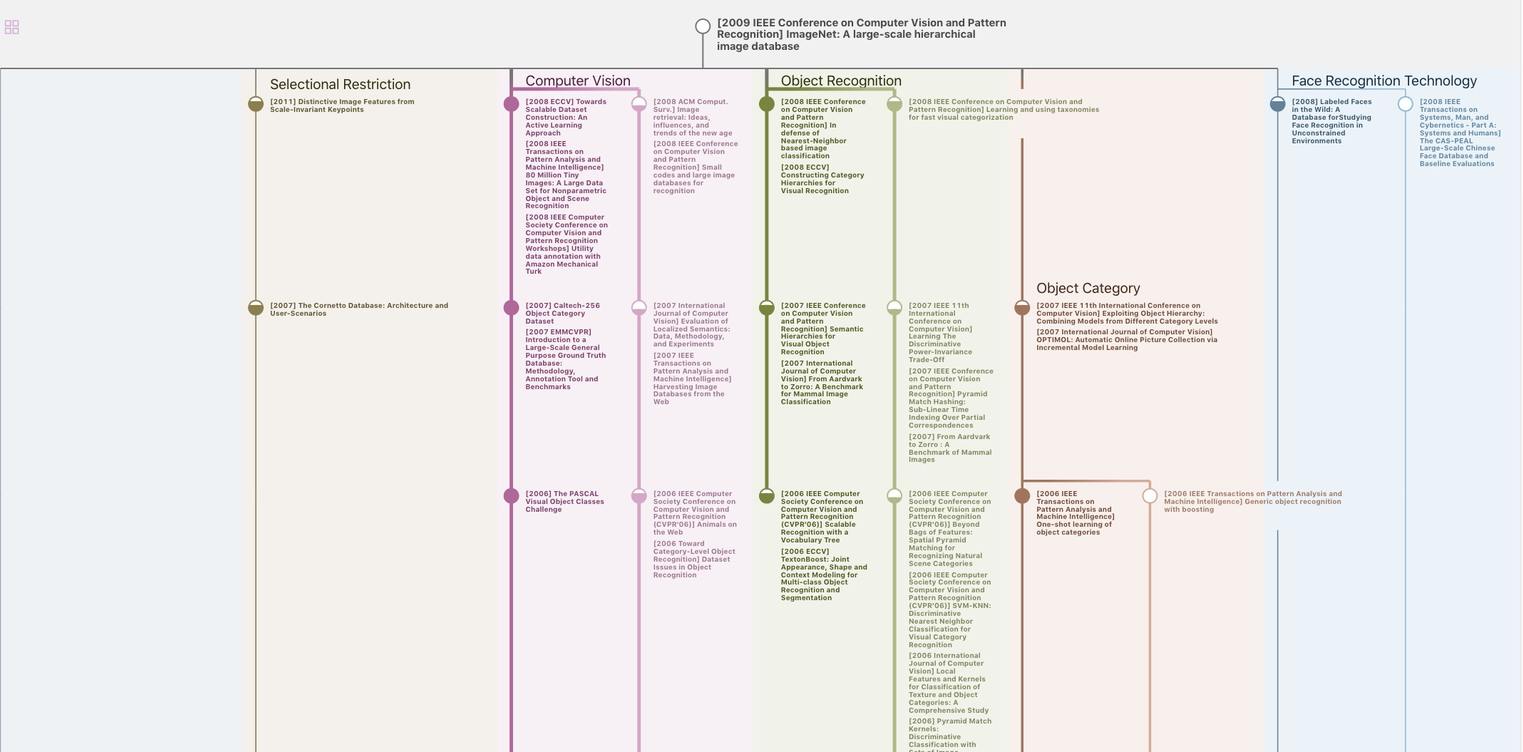
生成溯源树,研究论文发展脉络
Chat Paper
正在生成论文摘要