Estimating canopy chlorophyll in slash pine using multitemporal vegetation indices from uncrewed aerial vehicles (UAVs)
Precision Agriculture(2024)
摘要
Canopy Chlorophyll Content (CCC) is an important physiological indicator that reflects the growth stage of trees. Accurate estimation of CCC facilitates dynamic monitoring and efficient forest management. In this study, we used high-resolution remote sensing images obtained by uncrewed aerial vehicles (UAVs) equipped with multispectral sensors (red, green, blue, near-infrared, and red-edge) to estimate CCC of lodgepole pine ( Pinus elliottii ). Our aim was to determine the optimal machine learning model between support vector regression (SVR) and random forest regression (RFR) for predicting CCC and to evaluate the effectiveness of multispectral bands along with 21 vegetation indices (VIs) in the estimation process. Individual tree boundaries were derived from the canopy height model (CHM) based on three-dimensional (3D) point clouds generated using structure from motion. These images, combined with continuous field measurements from January to December, provided comprehensive data for our analysis. The results showed that the SVR method outperformed the RFR method in estimating leaf chlorophyll content (LCC), with fitting R 2 values up to 0.692 and RMSE values up to 0.168 mg⋅g −1 . Overall, the study highlights the potential of UAV-based remote sensing for multitemporal forest monitoring, offering advances in precision forestry and tree breeding.
更多查看译文
关键词
Uncrewed aerial vehicles (UAVs),Multispectral and multitemporal,Canopy chlorophyll content,Vegetation indices,Pinus elliottii
AI 理解论文
溯源树
样例
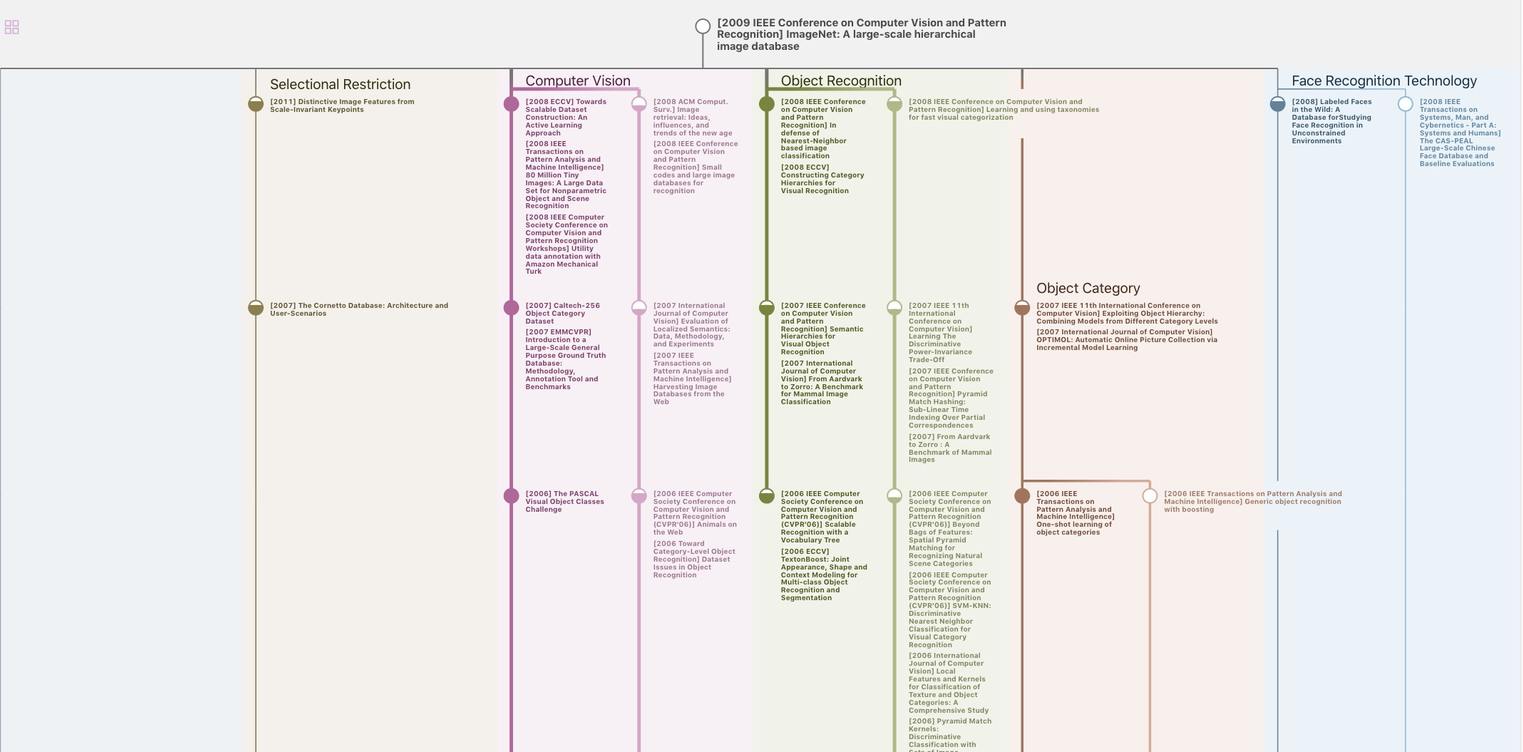
生成溯源树,研究论文发展脉络
Chat Paper
正在生成论文摘要