TLEE: Temporal-Wise and Layer-Wise Early Exiting Network for Efficient Video Recognition on Edge Devices
IEEE INTERNET OF THINGS JOURNAL(2024)
摘要
With the explosive growth in video streaming comes a rising demand for efficient and scalable video understanding. State-of-the-art video recognition approaches based on convolutional neural network (CNN) have shown promising performance by adopting 2-D or 3-D CNN architectures. However, the large data volumes, high resource demands, and strict latency requirements have hindered the wide application of these solutions on resource-constrained Internet of Things (IoT) and edge devices. To address this issue, we propose a novel framework called TLEE that enables the input samples the abilities of both temporal-wise and layer-wise early exiting on 2-D CNN backbones for efficient video recognition. TLEE consists of three types of modules: 1) gating module (GM); 2) branch module (BM); and 3) feature reuse module (FRM). The GM determines for an input video from which frame of this video to exit the per-frame computation, while the BM determines for an input frame from which layer of the CNN backbone to exit the per-layer computation. Besides, based on the accumulated features of frame sequences from exit branches, the FRM generates effective video representations to enable more efficient predictions. Extensive experiments on benchmark data sets demonstrate that the proposed TLEE can significantly outperform the state-of-the-art approaches in terms of computational cost and inference latency, while maintaining competitive recognition accuracy. In addition, we verify the superiority of TLEE on the typical edge device NVIDIA Jetson Nano.
更多查看译文
关键词
Dynamic inference,early exit,edge devices,efficient neural networks,video recognition
AI 理解论文
溯源树
样例
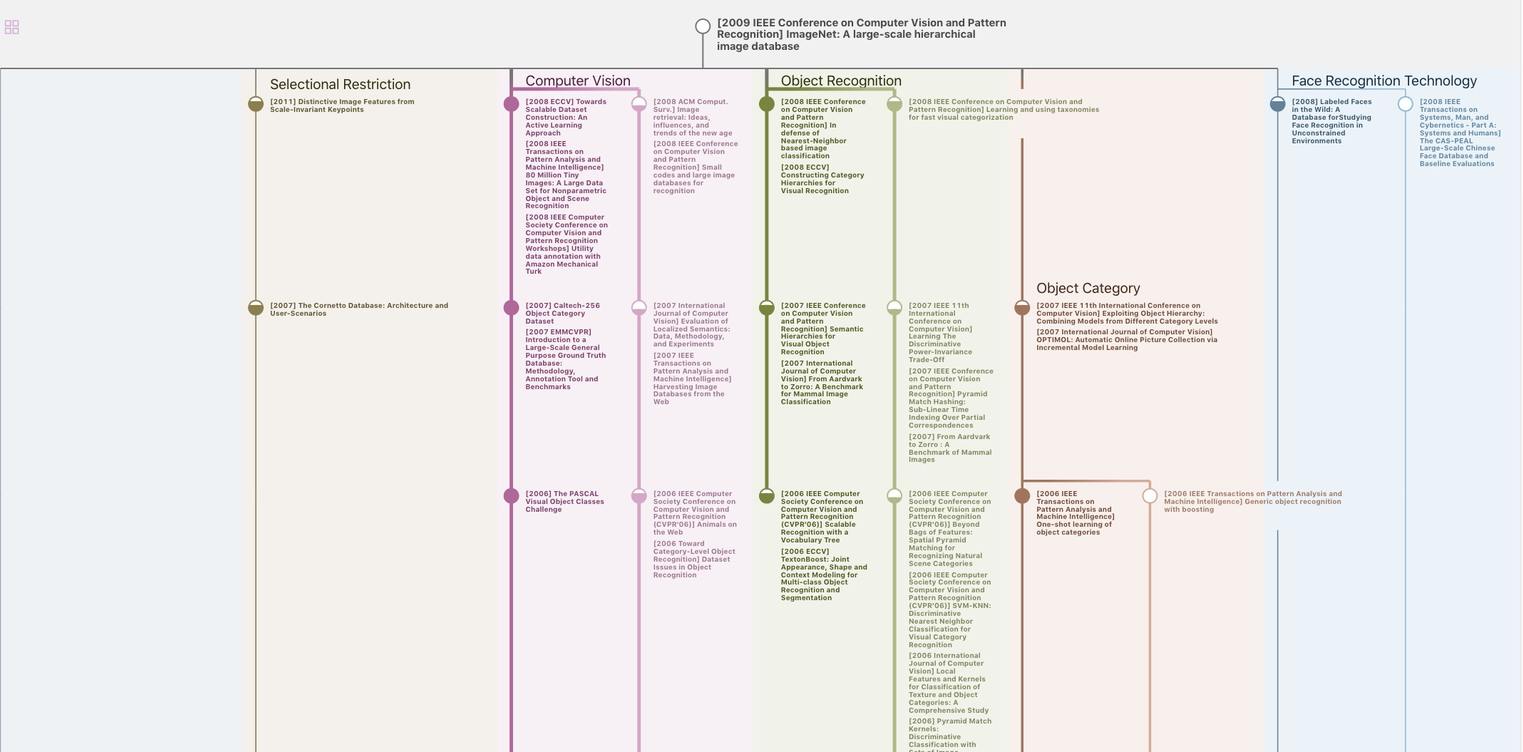
生成溯源树,研究论文发展脉络
Chat Paper
正在生成论文摘要