Importance Analysis Method Application for Feature Selection in a Classification.
2023 7th International Conference on System Reliability and Safety (ICSRS)(2023)
摘要
Classification is often used method in Machine Learning applications. Different factors cause the efficiency of this method. One of the very important factors is the quality of initial data, which among other things, includes a sufficient, but not excessive, number of input attributes. The procedure for defining a sufficient number of input attributes is known as feature selection. There are approaches to feature selection that form three groups: filter, wrapper, and embedded methods. Filters are used for any classifier and have lower computational complexity. The wrapper methods depend on the algorithm of classifier induction. A classification algorithm evaluates a subset of selected attributes according to a classification measure. These methods have high performance, but they require a lot of computation time to execute. Embedded methods perform attribute selection in the process of the classifier induction and depend on the classifier and algorithm of its induction. In this paper, we propose a new wrapper method based on the well-known approach in reliability analysis which is importance analysis. The proposed method's advantage is the independency of the classifier type and acceptable computational complexity. Therefore, it can be used for any type of classifier and the selected attributes are evaluated by the classification measures that increase the classifier's efficiency.
更多查看译文
关键词
Importance analysis,Structure function,Classification,attribute selection,Fuzzy Decision Tree (FDT)
AI 理解论文
溯源树
样例
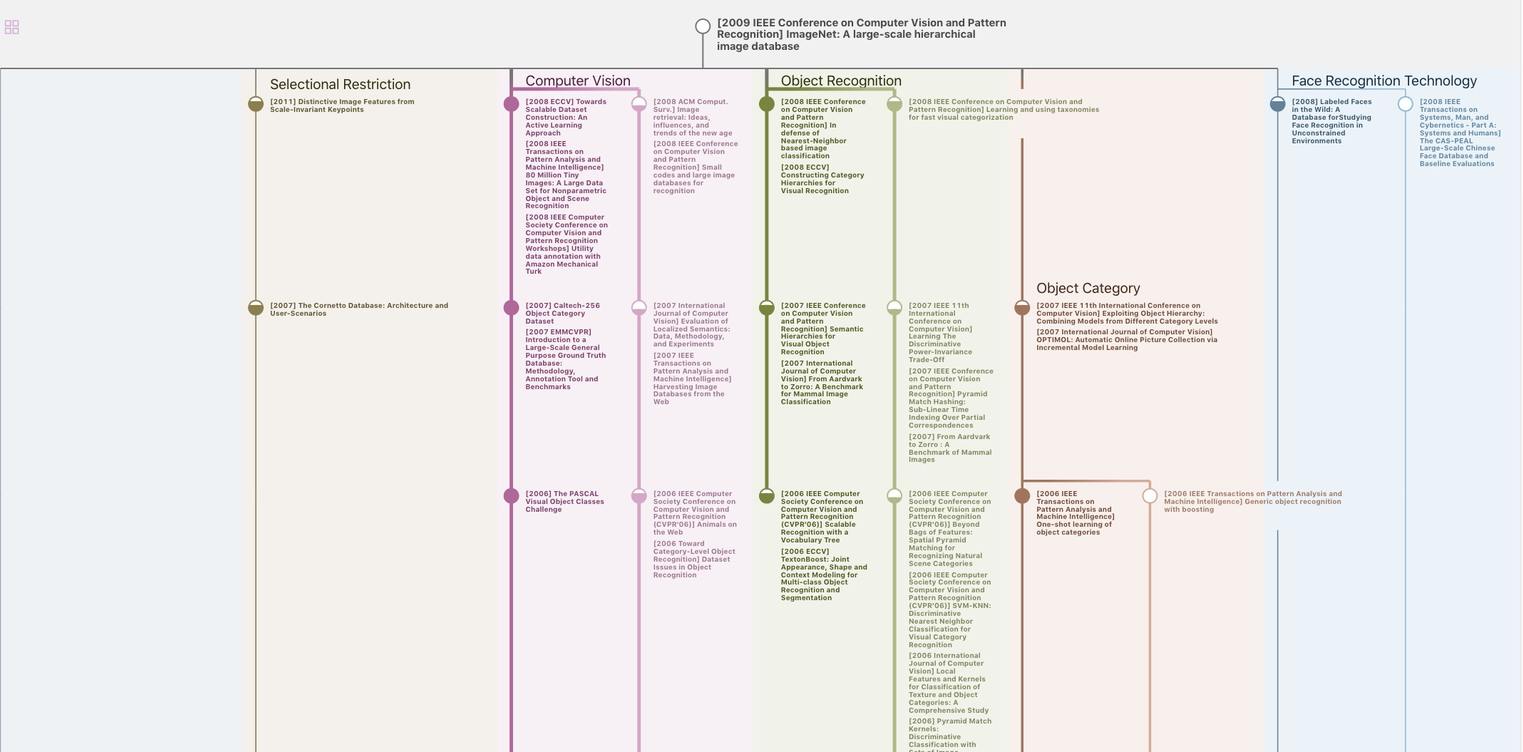
生成溯源树,研究论文发展脉络
Chat Paper
正在生成论文摘要