Automatically Identifying Sentences with Attack Behavior from Cyber Threat Intelligence Reports.
2023 8th International Conference on Data Science in Cyberspace (DSC)(2023)
摘要
In recent years, the automated extraction of attack behaviors from Cyber Threat Intelligence (CTI) reports has proven to be effective in rapidly responding to cyber threats. When extracting attack behaviors from CTI, it is crucial to identify the sentences that describe these behaviors. Identifying attack sentences from reports can be challenging due to semantic overlap between attack and non-attack sentences. To address this problem, We propose a sentence-level attack behavior identification method that integrates the semantics of sentences and labels, called BCC. It effectively identifies sentences in reports that describe attack behavior. Specifically, the BCC model uses the BERT layer to model sentences and labels by learning their similarity. The BCC employs a sliding window in the CNN layer to capture the interaction of IOC-level attack behaviors. During the model training process, we discovered that using one-hot labels leads to overconfidence. To address this issue, we introduced the LCM layer to learn the similarity between sentences and labels, capturing semantic overlap among labels. For experimental evaluation, we collected CTI reports from MANDIANT and UNIT 42 to construct a sentence-level attack behavior dataset. The dataset consists of 8,000 samples from CTI reports as the baseline corpus, and an additional 3,000 positive examples of IOC-level attack behavior sentences were generated using a customized template to prompt ChatGPT, ensuring a balanced distribution of positive and negative samples. On this dataset, the BCC model achieved significant results in identifying attack behavior sentences, with an F1 score of 94.70%. This outperformed baseline models such as EXTRACTOR (94.18%) and TTPDrill (90.98%). To validate the effectiveness of BCC in extracting subsequent attack behaviors, we conducted an experiment using five manually annotated CTIs. The results show that the BCC model significantly improves the accuracy of attack behavior extraction.
更多查看译文
关键词
Identifying Sentence Attack Behavior,BERT,Convolution,Label confusion
AI 理解论文
溯源树
样例
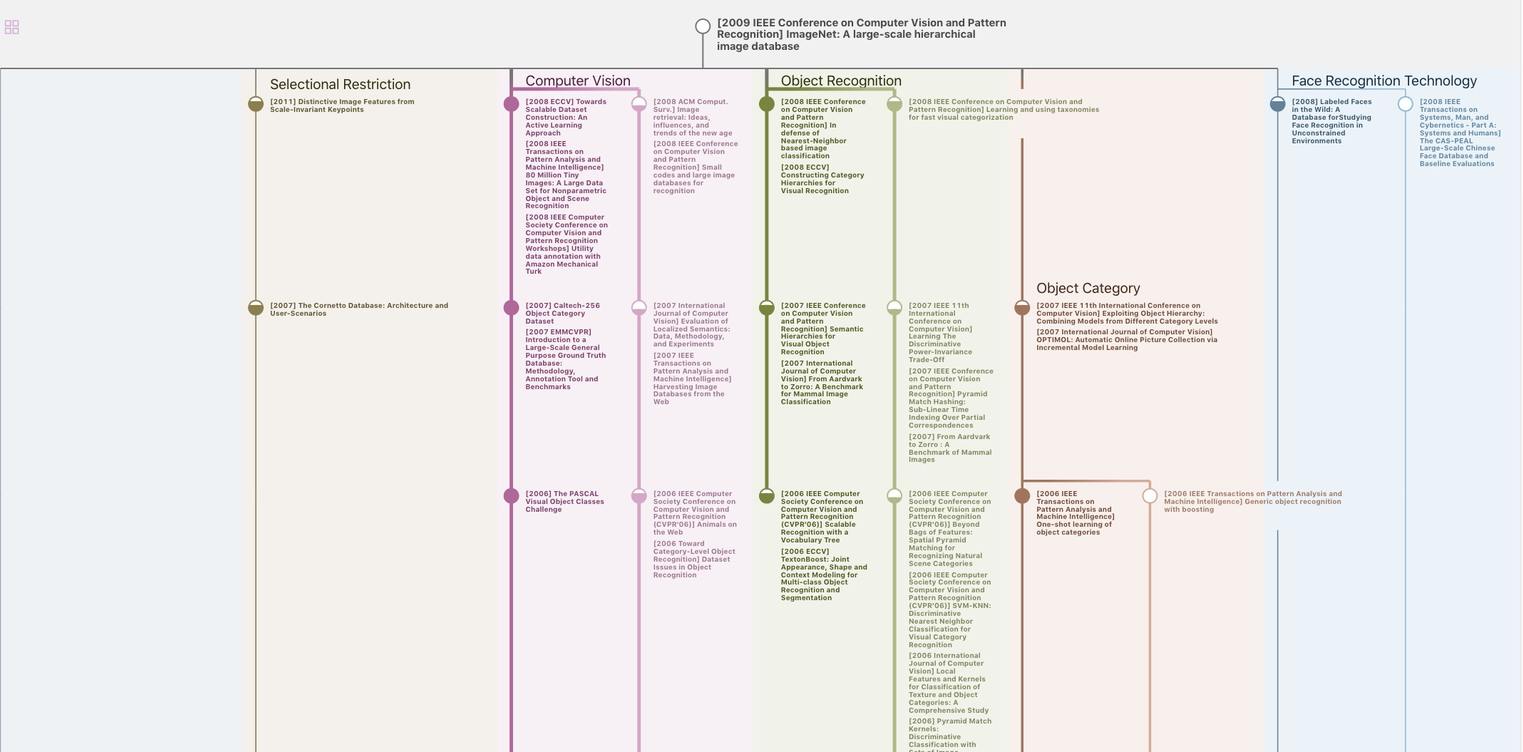
生成溯源树,研究论文发展脉络
Chat Paper
正在生成论文摘要