Improving the performance and explainability of knowledge tracing via Markov blanket
INFORMATION PROCESSING & MANAGEMENT(2024)
摘要
Knowledge tracing predicts student knowledge acquisition states during learning. Traditional knowledge tracing methods suffer from poor prediction performance; however, recent studies have significantly improved prediction performance through the incorporation of deep neural networks. However, prediction results generated from deep knowledge tracing methods are typically difficult to explain. To solve this issue, a knowledge tracing model using Markov blankets was proposed to improve the interpretability of knowledge tracing. The proposed method uses the Markov blanket of the target variable as a subset of features and applies interpretable machine learning techniques to knowledge tracing. The results from the ablation experiments demonstrate that the feature subspace created by the Markov blanket is substantially effective for prediction. The proposed model also performs better than several other knowledge tracing models on two widely used datasets, i.e., Junyi and ASSISTments. Furthermore, the use of Markov blanket -based features provides high interpretability for predicting knowledge mastery states, elucidating the impact of these features on student knowledge acquisition. Moreover, this enables the use of previously considered low -correlation features, which may possess important latent causal relationships.
更多查看译文
关键词
Knowledge tracing,Markov blanket,Interpretable models,Educational AI,Causal discovery
AI 理解论文
溯源树
样例
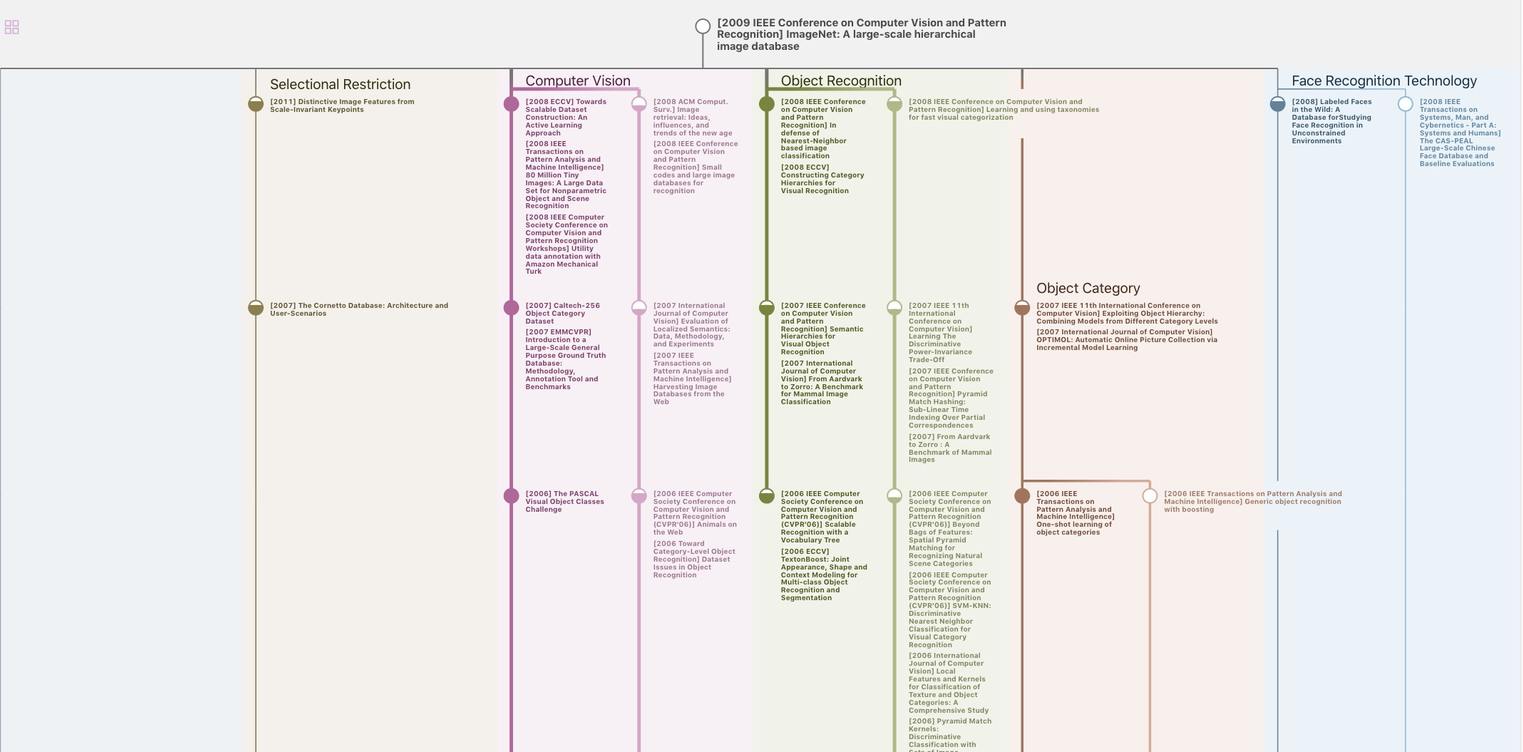
生成溯源树,研究论文发展脉络
Chat Paper
正在生成论文摘要