RoSA: Accurate Parameter-Efficient Fine-Tuning via Robust Adaptation
CoRR(2024)
摘要
We investigate parameter-efficient fine-tuning (PEFT) methods that can
provide good accuracy under limited computational and memory budgets in the
context of large language models (LLMs). We present a new PEFT method called
Robust Adaptation (RoSA) inspired by robust principal component analysis that
jointly trains low-rank and highly-sparse components on
top of a set of fixed pretrained weights to efficiently approximate the
performance of a full-fine-tuning (FFT) solution. Across a series of
challenging generative tasks such as grade-school math and SQL query
generation, which require fine-tuning for good performance, we show that RoSA
outperforms LoRA, pure sparse fine-tuning, and alternative hybrid methods at
the same parameter budget, and can even recover the performance of FFT on some
tasks. We provide system support for RoSA to complement the training algorithm,
specifically in the form of sparse GPU kernels which enable memory- and
computationally-efficient training, and show that it is also compatible with
low-precision base weights, resulting in the first joint representation
combining quantization, low-rank and sparse approximations. Our code is
accessible at https://github.com/IST-DASLab/RoSA.
更多查看译文
AI 理解论文
溯源树
样例
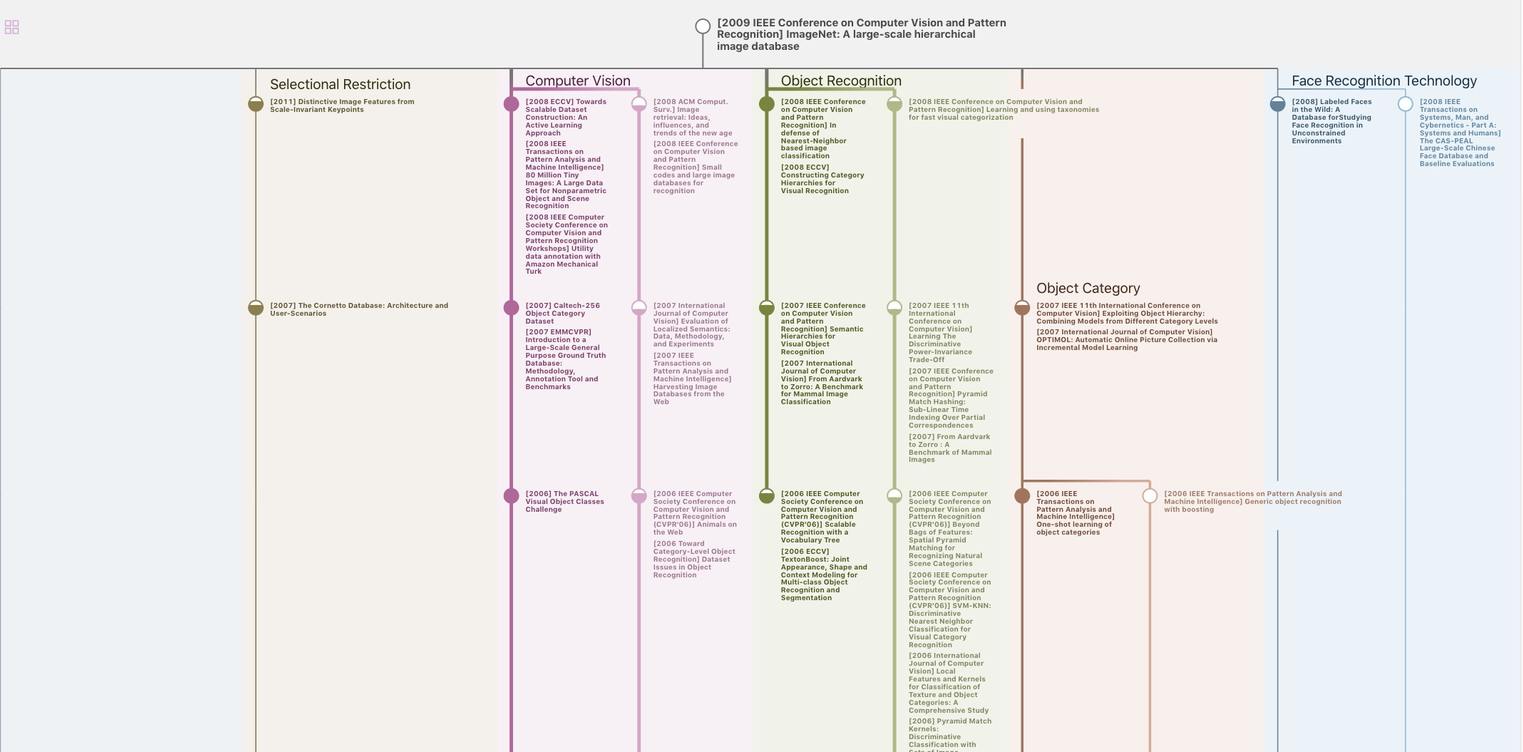
生成溯源树,研究论文发展脉络
Chat Paper
正在生成论文摘要