Image Classification Network Enhancement Methods Based on Knowledge Injection
CoRR(2024)
摘要
The current deep neural network algorithm still stays in the end-to-endtraining supervision method like Image-Label pairs, which makes traditionalalgorithm is difficult to explain the reason for the results, and theprediction logic is difficult to understand and analyze. The current algorithmdoes not use the existing human knowledge information, which makes the modelnot in line with the human cognition model and makes the model not suitable forhuman use. In order to solve the above problems, the present invention providesa deep neural network training method based on the human knowledge, which usesthe human cognition model to construct the deep neural network training model,and uses the existing human knowledge information to construct the deep neuralnetwork training model. This paper proposes a multi-level hierarchical deeplearning algorithm, which is composed of multi-level hierarchical deep neuralnetwork architecture and multi-level hierarchical deep learning framework. Theexperimental results show that the proposed algorithm can effectively explainthe hidden information of the neural network. The goal of our study is toimprove the interpretability of deep neural networks (DNNs) by providing ananalysis of the impact of knowledge injection on the classification task. Weconstructed a knowledge injection dataset with matching knowledge data andimage classification data. The knowledge injection dataset is the benchmarkdataset for the experiments in the paper. Our model expresses the improvementin interpretability and classification task performance of hidden layers atdifferent scales.
更多查看译文
关键词
image classification,explainable model,knowl-edge graph
AI 理解论文
溯源树
样例
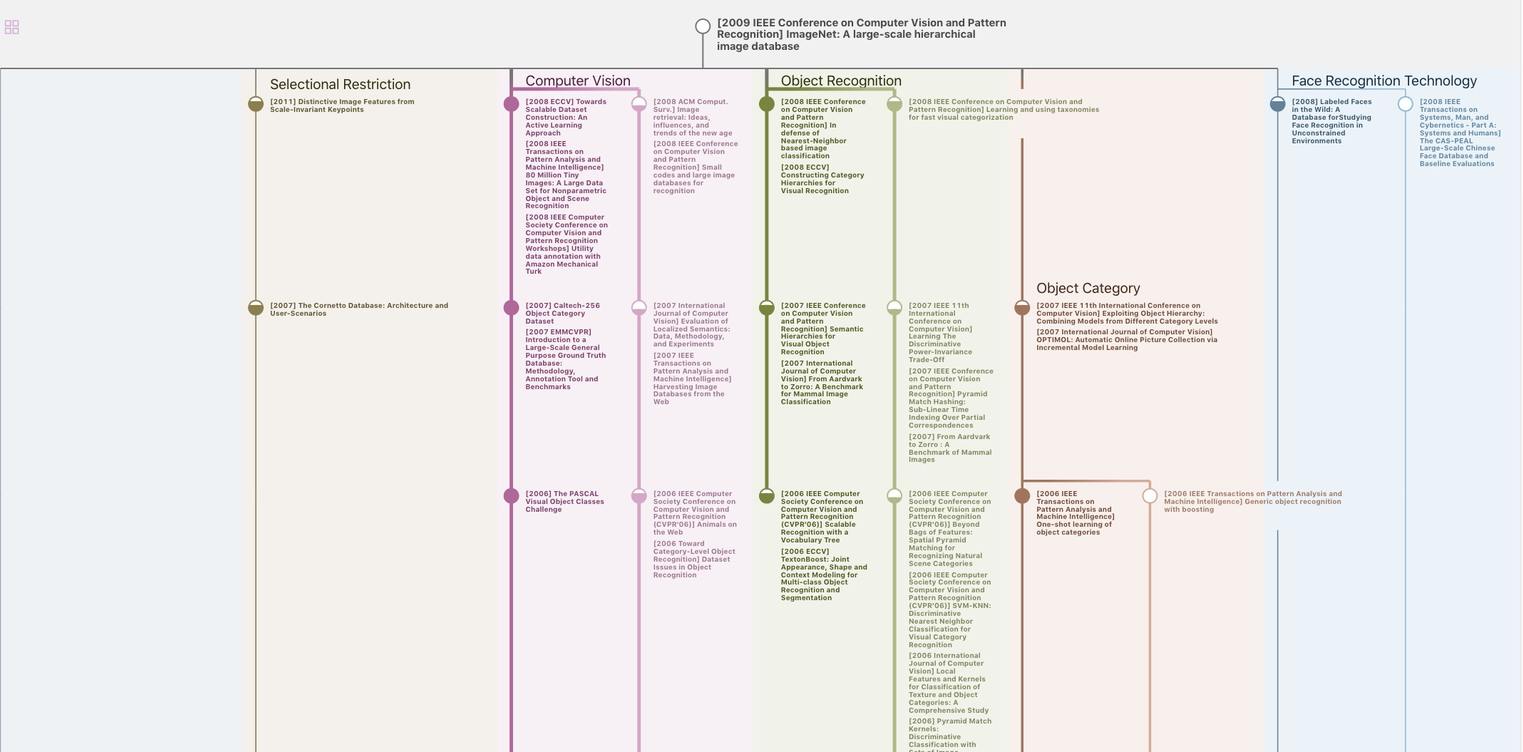
生成溯源树,研究论文发展脉络
Chat Paper
正在生成论文摘要