Incorporating attribute-level aligned comparative network for generalized zero-shot learning
NEUROCOMPUTING(2024)
摘要
The key challenge of zero -shot learning (ZSL) is to sufficiently disentangle each latent attribute from the class -level semantic annotations of images, thereby achieving a desirable semantic transfer to unseen classes with the disentangled attributes. However, most existing studies tackle the ZSL task with a strict classlevel alignment strategy that may yield insufficient disentanglement: (1) this strategy simply aligns holistic visual feature with its associated class -level semantic vector for each image; (2) the class -level semantic vectors have limited diversity and complex compositions of attributes. To address these issues, we propose an incorporating attribute -level aligned comparative network, i.e., IAAC-net, that develops the alignment strategy of ZSL to the attribute level. IAAC-net aims to establish diversified attribute -level and refined class -level alignments to facilitate attribute disentanglement and simultaneously improve zero -shot generalization. By further proposing a confusion -aware loss, the model is forced to rectify the disentanglement of indistinguishable attributes, which leads to a more accurate attribute disentanglement. The proposed IAAC-net yields significant improvements over the strong baselines, leading to new state-of-the-art performances on three popular challenging benchmarks, i.e., CUB, SUN, and AWA2.
更多查看译文
关键词
Zero-shot learning,Visual-semantic alignment,Representation learning,Transfer learning,Image classification
AI 理解论文
溯源树
样例
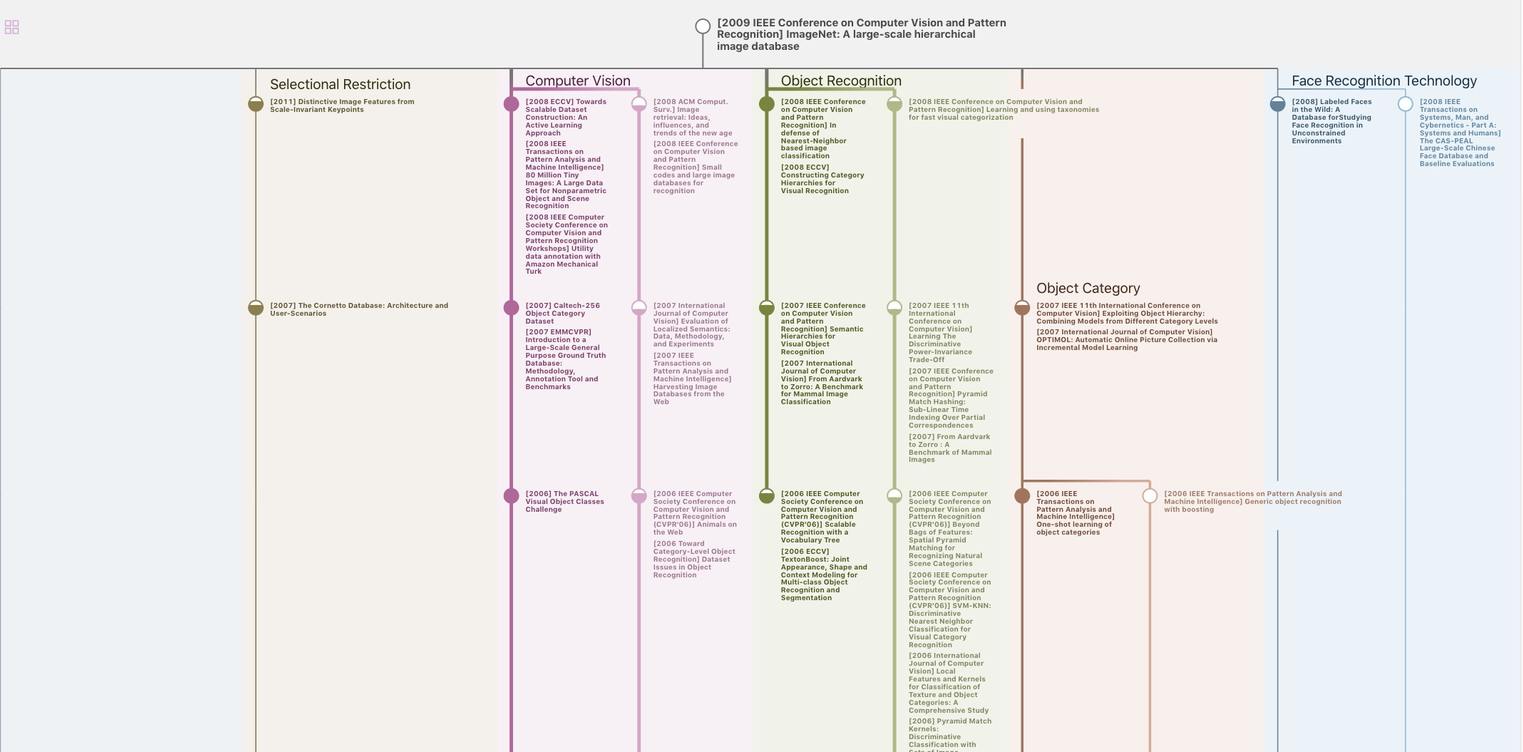
生成溯源树,研究论文发展脉络
Chat Paper
正在生成论文摘要