Predicting 4D hardness property from 3D datasets for performance-tunable material extrusion additive manufacturing
MATERIALS TODAY COMMUNICATIONS(2024)
摘要
This study explores the application of machine learning in predicting the hardness of a quaternary polymer blends during 3D printing, aiming to reduce the development and experimental costs of mixing extrusion head and to expedite the realization of multi -material co -blending printing technology. We selected four types of polymer materials: polylactic acid (PLA), thermoplastic polyurethane (TPU), polyethylene terephthalate glycol (PETG), acrylonitrile butadiene styrene (ABS), and obtained composite materials of various hardness values through random combinations of three (from four) materials. Subsequently, hyperparameter optimization of five machine learning meta -algorithms was performed using particle swarm optimization (PSO) and genetic algorithms (GA), resulting in effective predictive models. Finally, a four -in -one mixing extrusion head was manufactured, and the predicted hardness values were compared with actual measurements. This study builds on old work by employing fewer data dimensions and a limited number of training samples to achieve high-fidelity hardness predictions for a wider range of print material co -blending printing, with significant improvements in both efficiency and effectiveness. Compared with traditional methods, the design of multi -material coblending print products can be more easily and quickly realized with the use of ML predictive modeling to assist the design of multi -material co -blending print products. This in turn reduces resource waste and time costs, and can be deployed in the foreseeable future in various additive manufacturing fields (aerospace, biomedical, etc.) that require higher manufacturing costs.
更多查看译文
关键词
Polymer blends,Rapid prototyping,Machine learning,Genetic algorithm,Particle swarm optimization
AI 理解论文
溯源树
样例
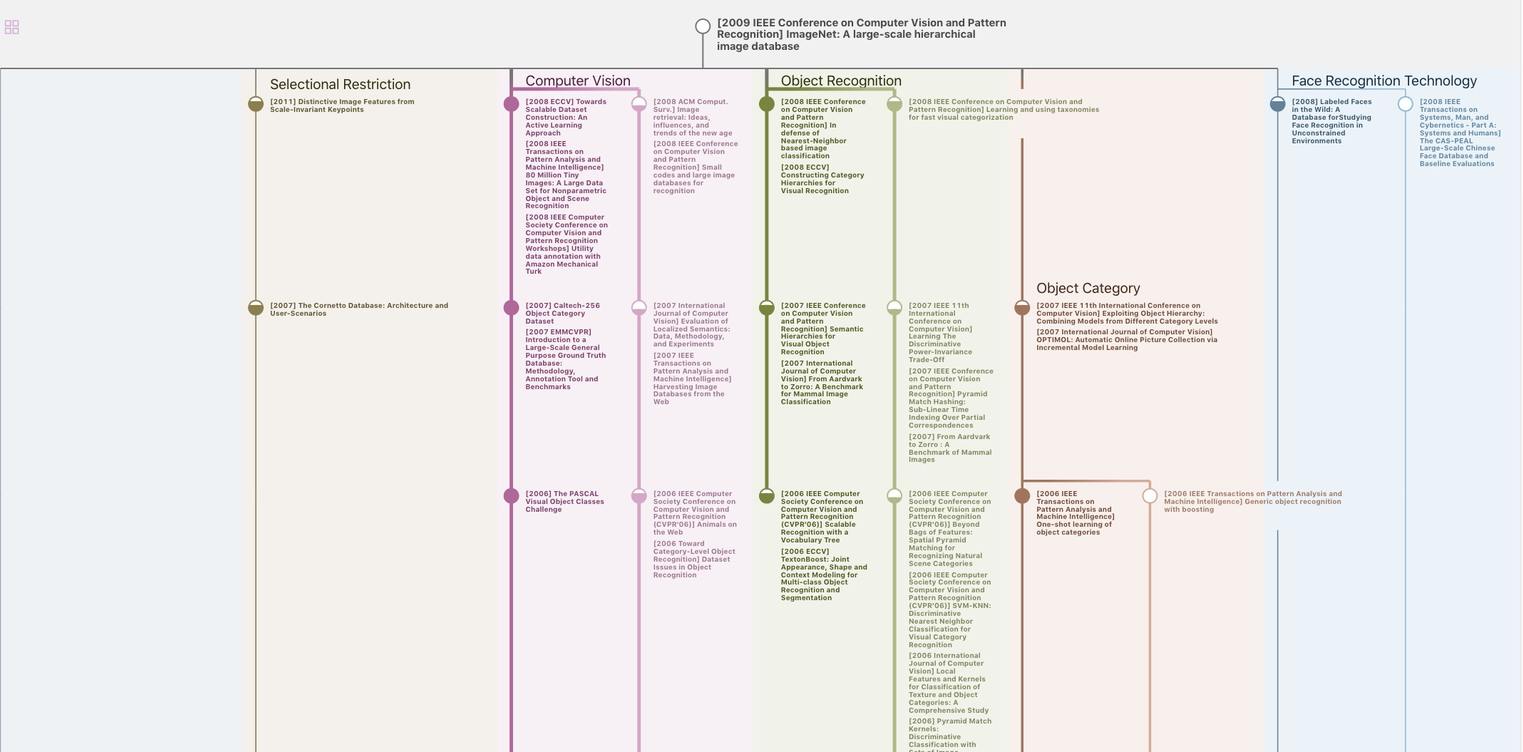
生成溯源树,研究论文发展脉络
Chat Paper
正在生成论文摘要