Disentangling Three Different Conflicts at Non-Response/semantic and Response Levels in a New Stroop-Matching Task
Learning and motivation(2024)
摘要
Stroop-like tasks have been used to examine adaptive control in order to overcome distracting information. Debate about the source of interference in Stroop-like tasks is ongoing, and two main sources of conflicts have been consistently mentioned: response and non-response (e.g., semantic) conflicts. Different behavioral protocols have been proposed to disentangle the contribution of each level of conflict. In the new Stroop-matching task adopted in this study, participants had to match the relevant Stroop attribute (color or word, depending on the instructions) to two color-words or two colored bars laterally presented, before emitting their manual responses – with only between-attributes matches being adopted. Response latency and accuracy were analyzed. By means of specific comparisons among conditions, in which the relevant and irrelevant Stroop attribute changed, our results allowed three different sources of interference to be disentangled at the non-response/semantic and response levels, which affect accuracy and/or response latency: the congruency, the response-set and relationship effects. Our new Stroop-matching task and the results obtained were critically discussed and compared with others. We found evidence to corroborate the studies that support separate and independent sources of conflict, and the asymmetric pattern of interference of each Stroop attribute (color and word). Finally, we concluded that Stroop-like tasks can present at least three different conflicts, at both the non-response and response levels, giving support to the multistage models of Stroop interferences, and indicating that task demands are the critical point to determine which (and how) the conflicts will affect the behavioral performance.
更多查看译文
关键词
Stroop task,Stroop interference,Congruency effect,Response -set effect,Conflict resolution,Reaction times
AI 理解论文
溯源树
样例
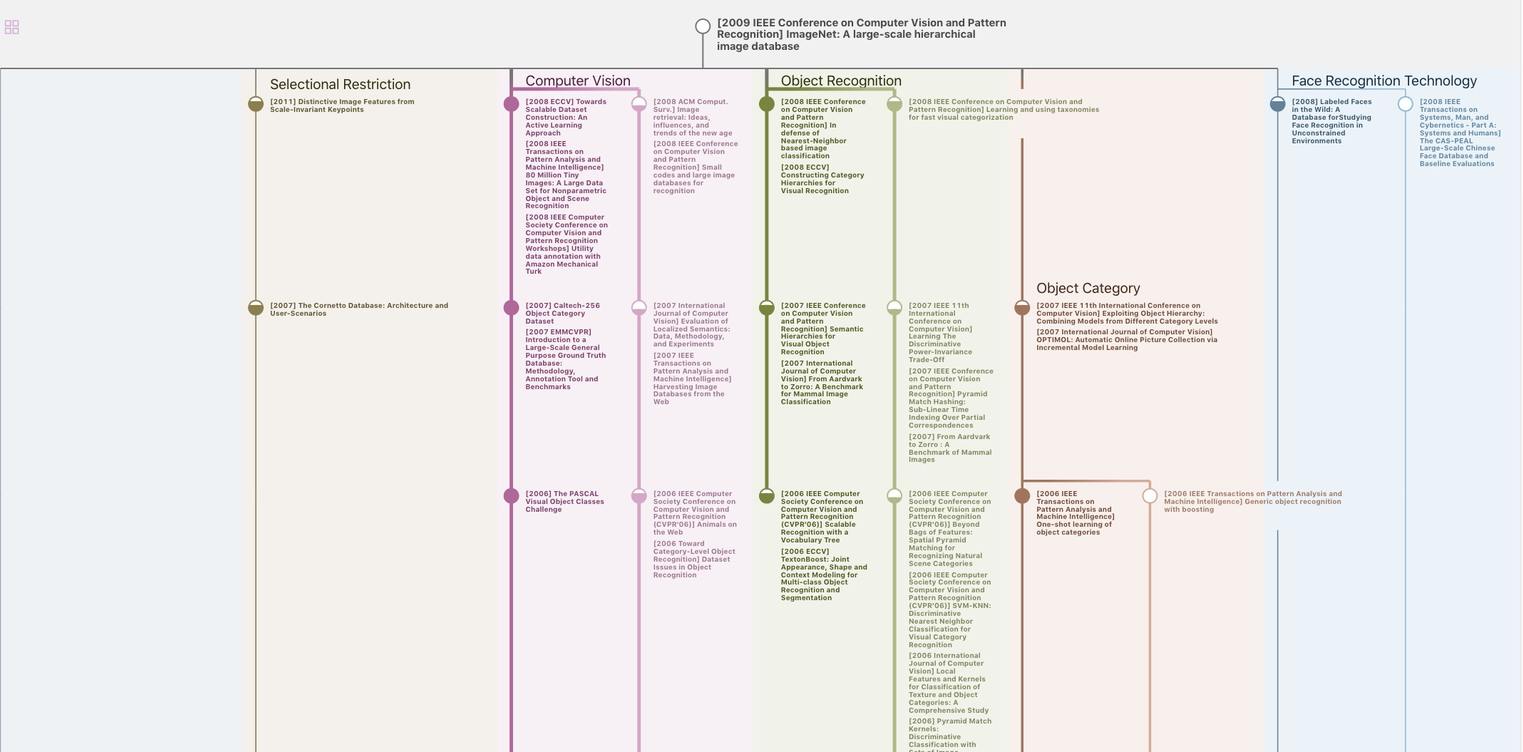
生成溯源树,研究论文发展脉络
Chat Paper
正在生成论文摘要